In the world of machine learning, building a model that performs well is only part of the challenge. The true measure of success lies in how that model behaves when faced with data it has never seen before. This is where validation data steps in as a critical checkpoint in the machine learning workflow.
Validation data is a portion of the dataset that is set aside during the training process. Unlike the training data, it is not used to teach the model. Instead, it’s used to evaluate how well the model is learning and, more importantly, whether it’s learning the right things. It acts as a mirror, reflecting the model’s ability to generalize—its capacity to make accurate predictions on real-world, unseen data.
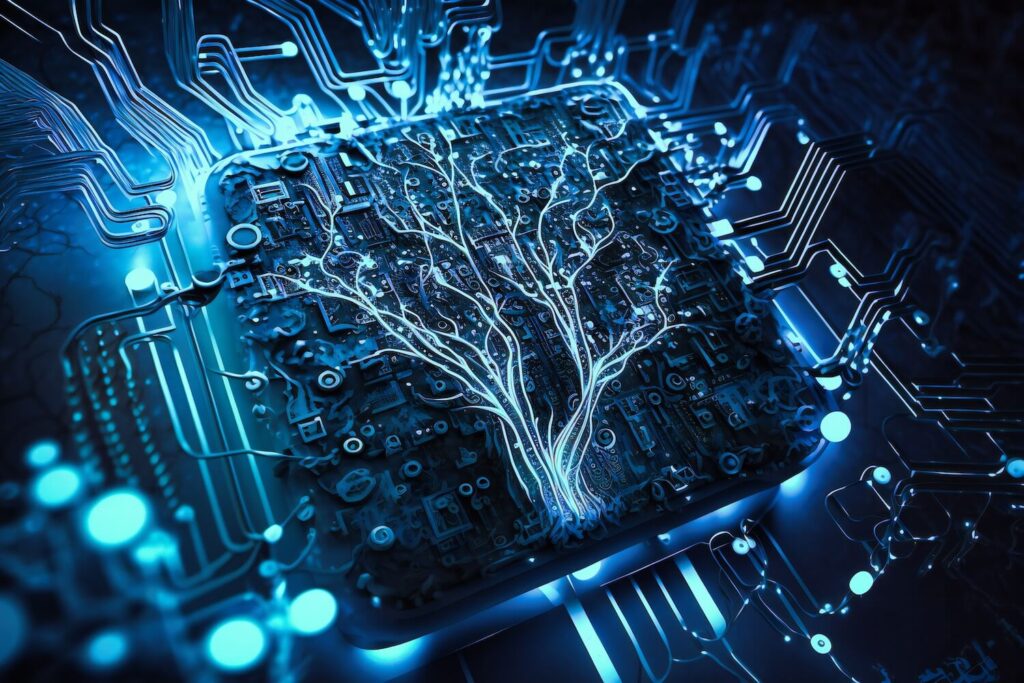
Understanding Validation Data
The Role of Validation Sets in ML
Models in the machine learning field learn from data to recognize patterns, make decisions based on them, and apply those patterns to new data. The worth of those models is measured in the way they deal with unseen data.
The validation data helps in the ML process by allowing professionals to have a measure of how well the model will perform in real-world cases. It assesses the capability and effectiveness of a model. The training phase for a machine learning model is the period in which parameter adjustments are applied so that it is aligned with training data.
However, in case the model fits "too closely" to this data, it may excel on the training set but may fail when being presented with new data – this is called “overfitting”. The validation data helps in the early detection of overfitting and helps the model remain reliable.
The validation data essentially brings to light both the strengths and weaknesses of a model before it’s applied. It helps in fine-tuning the models via hyperparameter and configuration fine-tuning while balancing bias with variance – key influencer elements of the overall model performance.
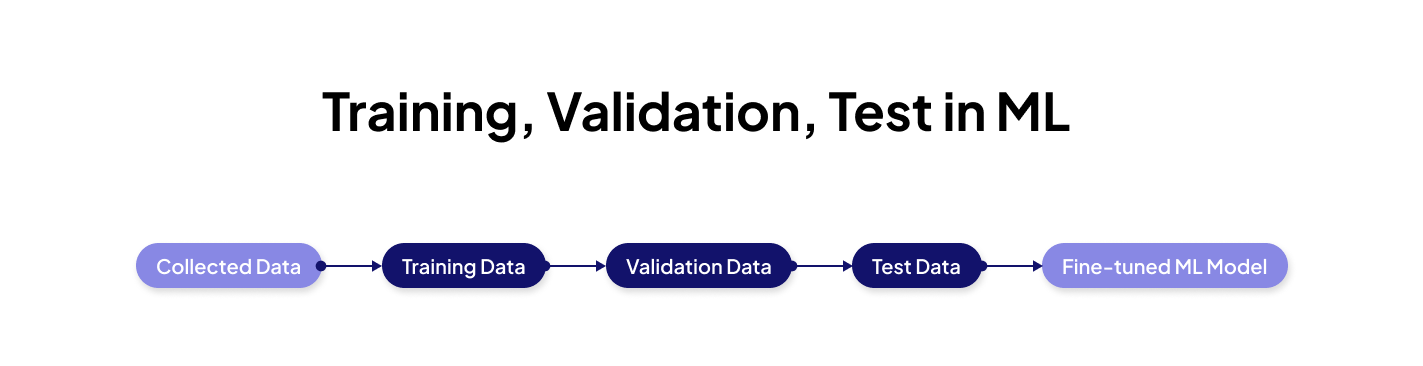
Comparing Training, Testing, and Validation Sets
Distinguishing between these three types of datasets is essential in machine learning practice.
Training Data
This dataset is mainly utilized for building and training the model. It assists the model in recognizing patterns and making predictions based on provided information.
Let’s say you’re building a spam filter for emails. The training data will include a bunch of emails labeled as “spam” or “not spam.” The model uses this to learn what typical spam looks like — words like “lottery,” “win now,” or suspicious links.
Validation Data
Validation data serves as a bridge between training and testing, ensuring that the model performs effectively on data. During the model training phase, this data plays a role in adjusting the model’s parameters to prevent overfitting.
You try different versions of your spam filter: one that looks at just the subject line, and another that looks at both the subject and content. You use validation data (emails the model hasn’t seen before) to see which version is working better. It helps you decide what settings (or “hyperparameters”) to go with.
Testing Data
The testing dataset is vital for assessing how well the model works on new, unfamiliar data before deployment.
Now that you’ve trained your spam filter and fine-tuned it using validation data, you test it on completely new emails. These emails were never used during training or validation. If your model catches spam accurately here, it’s ready to go live.
Best Practices for Preparing Validation Data
Representativeness
It is clearly understood that the validation set should represent all types of data and all the situations that the model is going to face after deployment. For instance, when conducting a study on image classification, the validation set should include images from categories in proportions that mirror real-world distributions.
Size and Balance
Another critical point here is the size of the validation set. It usually has to make up 10-20% of the entire dataset. Validation set has to be balanced across classes or outcomes not to bias predictions made by the model.
Separation from Training Data
The goal is to ensure that validation data is disjoint from the training dataset. This is done to prevent data leakage that may produce over-optimistic performance evaluations. A study published in the “Journal of Analysis and Testing” highlights the importance of separating training and validation datasets in machine learning model evaluation. It emphasizes that having a sensible data splitting strategy is crucial for building a model with good generalization performance.
Consistency over Time (for time-series data)
When working with time-series data, there is great attention that has to be put into making sure the validation data is chronologically lined up with the training set. To that end, the validation dataset should be drawn from the same period or from similar seasonal and cyclical patterns.
Data Preprocessing
The validation data needs to undergo preparation and refining steps to maintain its consistency. This includes handling missing values, standardizing or normalizing the data, and encoding variables.
The Influence of Data Quality and Quantity on Model Performance
The quality and quantity of validation data directly impact the accuracy and reliability of machine learning models. Key elements deemed to be part of data quality cover precision, completeness, consistency, and relevance. High-quality data is crucial for training and validating models. On the other hand, poor data quality – in the form of inaccuracy, missing values, inconsistency, and irrelevance – can lead to misleading outcomes. This will make the model learn the wrong patterns and relationships that will affect its performance on new data.
Quantity refers to the volume of data used for training, validating, and testing the model. Having the right amount of data is crucial for the model to grasp the underlying patterns and complexities of the dataset. Insufficient or inadequate data may result in the model failing to capture the intricacies of the problem domain. This can lead to underfitting – a scenario where the model is too simplistic to explain the dataset.
On the other hand, having a large amount of data from various sources can help the model make better predictions on new data that it hasn't seen before. However, it's crucial to keep in mind that too much data can lead to wasted resources and potentially overinflated performance evaluations.
Both the quality and quantity of data are vital in determining the effectiveness of validation datasets. Validation data should mirror real-world scenarios that the model will encounter after deployment.
Finding a balance between data quality and quantity is essential for model performance. High-quality data can compensate for its quantity by providing varied examples for the model to learn from. Conversely, having a large dataset can help address some quality issues by enabling the model to recognize patterns in noisy environments.
Methods for Generating Validation Data
Creating validation data involves splitting the original dataset into training and validation subsets. Here are some of the most common data splitting techniques used for creating validation sets:
The Holdout Approach
The holdout technique divides the dataset into two parts: one for training and one for validation/testing purposes. While this method is straightforward, its reliability may be compromised if the split doesn't accurately represent the distribution of data. A common ratio of data splitting is 70% for training and 30% for validation and testing to prevent overfitting and ensure the model performs well on unseen data.
from sklearn.model_selection import train_test_split # Example: Split 70% training and 30% validation X_train, X_val, y_train, y_val = train_test_split(X, y, test_size=0.3, random_state=42)
Simple and fast, but might not capture full data patterns if your dataset is small or unbalanced.
K-Fold Cross-Validation
In K-Fold Cross-Validation the dataset is divided into k equal-sized folds, with each serving as a validation set in turns. The model is then trained on k-1 folds and validated on the remaining fold, rotating until each fold has been used for validation. This method is preferred over holdout as it reduces bias and variance in evaluating the model.
from sklearn.model_selection import KFold kf = KFold(n_splits=5) for train_index, val_index in kf.split(X): X_train, X_val = X[train_index], X[val_index] y_train, y_val = y[train_index], y[val_index] # Train your model here
Good for reducing variance and getting a more stable performance estimate.
Stratified K-Fold Cross-Validation
This is a variation of k-fold cross-validation where each part includes a similar distribution of class labels as the original dataset. It's beneficial for datasets with imbalanced class distribution as it ensures each fold represents the dataset accurately and yields model performance metrics.
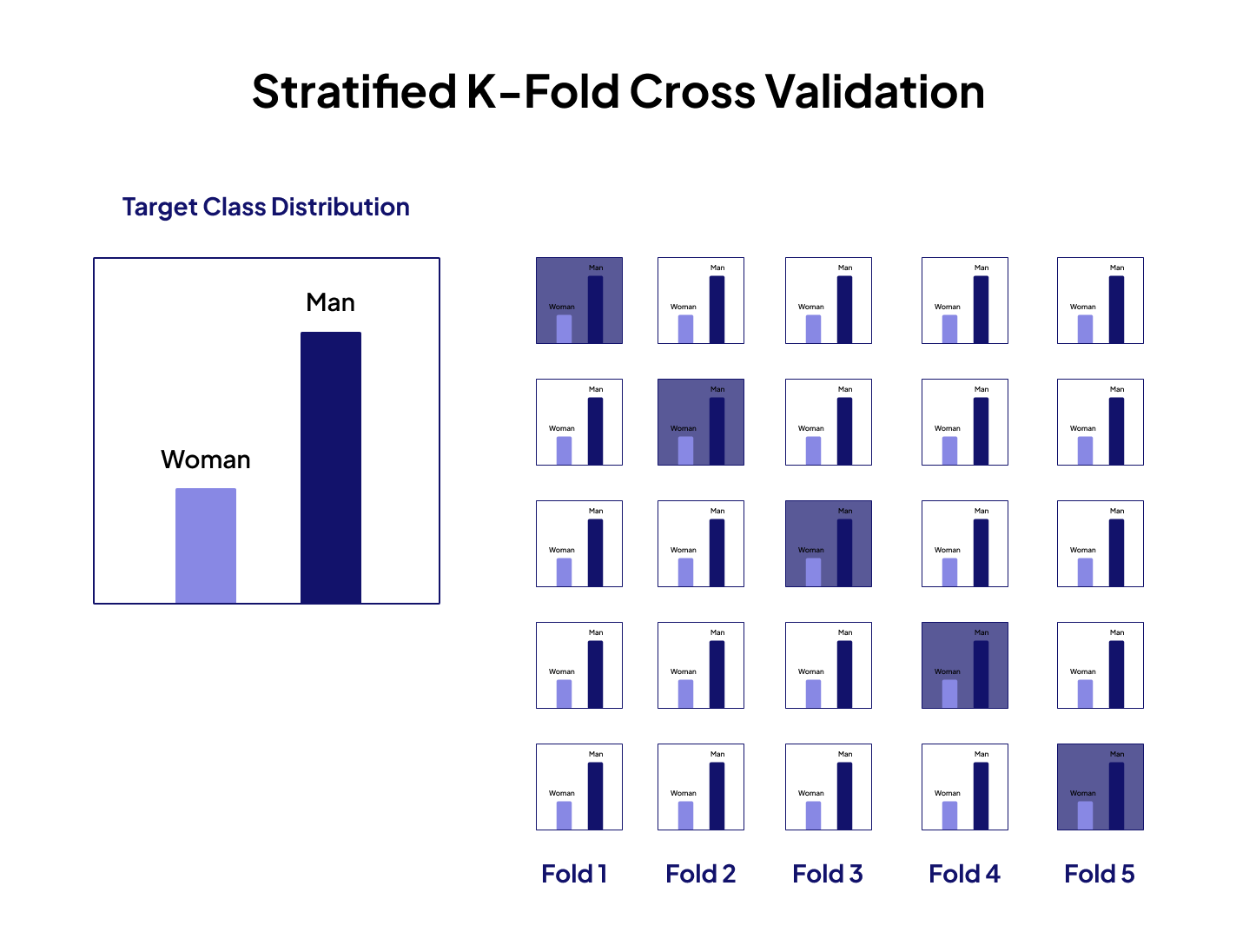
It helps keep class proportions consistent in each fold — great for tasks like fraud detection or medical diagnosis.
from sklearn.model_selection import StratifiedKFold skf = StratifiedKFold(n_splits=5) for train_index, val_index in skf.split(X, y): X_train, X_val = X[train_index], X[val_index] y_train, y_val = y[train_index], y[val_index]
Leave One Out Cross Validation (LOOCV)
LOOCV is an extreme form of k-fold cross-validation. Each iteration uses all data points except one for training and reserves the omitted point for validation. Although LOOCV can be resource-intensive, it maximizes the utilization of both training and validation data.
from sklearn.model_selection import LeaveOneOut loo = LeaveOneOut() for train_index, val_index in loo.split(X): X_train, X_val = X[train_index], X[val_index] y_train, y_val = y[train_index], y[val_index]
Very accurate, but slow for large datasets — usually used for small data.
Time-Series Cross-Validation
This method is designed specifically for time-series data. It aims to ensure that the validation set corresponds to the same time frame as the training set, preventing any leakage of temporal information. This technique involves incrementally moving the training window and testing on the following period. For example, in forecasting financial trends, time-series cross-validation can effectively evaluate how well a model can predict data by considering time-related patterns and trends.
from sklearn.model_selection import TimeSeriesSplit tscv = TimeSeriesSplit(n_splits=5) for train_index, val_index in tscv.split(X): X_train, X_val = X[train_index], X[val_index] y_train, y_val = y[train_index], y[val_index]
Keeps your model honest by only training on past data and validating on future steps — great for stock prediction, sales forecasting, etc.
A recent research has highlighted the significance of choosing the right data splitting technique to enhance model performance. It shows that while all data splitting methods can bring comparable results for large datasets, different methods used for small datasets can dissimilarly impact model performance. The optimal choice of data splitting technique depends on the data itself.
Using Validation Datasets in Model Training: Step-by-Step
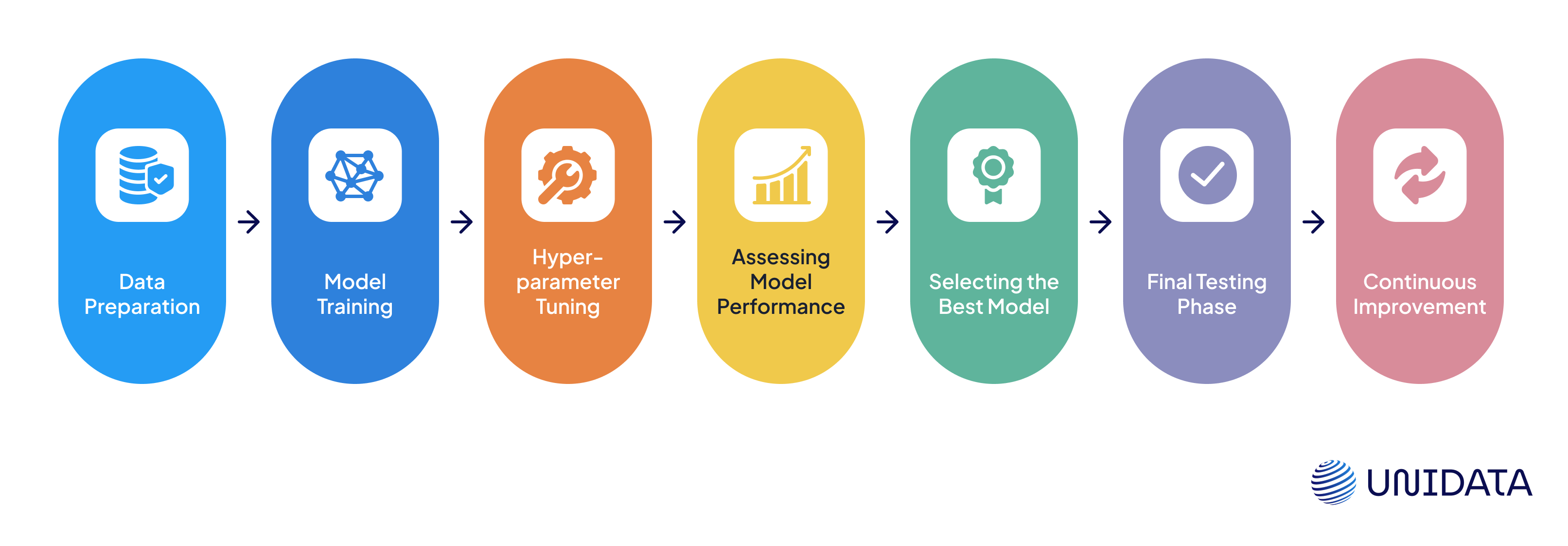
1. Data Preparation
Before incorporating the validation dataset, it is essential to preprocess both the training and validation datasets. This includes addressing missing values, feature scaling, and encoding variables. For instance, if normalization or standardization methods are used, make sure that the same transformation parameters are applied to both the training and validation sets – this will maintain data consistency.
2. Model Training
Begin by training your model with the provided training dataset. During this phase, the model learns how to correlate input features with the target variable.
3. Hyperparameter Tuning
Make use of the validation set to fine-tune your model’s hyperparameters. Hyperparameters refer to configuration settings that shape how a machine learning model operates and can significantly impact its performance.
Methods such as grid search, random search, or Bayesian optimization can be utilized for this task.
4. Assessing Model Performance
Evaluate how well the model performs on the validation set to gauge its performance on new data. Common metrics used for evaluation include accuracy, precision, recall, and F1-score for classification tasks, and mean squared error or mean absolute error for regression tasks.
5. Selecting the Best Model
Opt for the model or configuration that demonstrates the best performance on the validation dataset. This is critical because it's not always the model that performs best on the training data that will do well on unseen data.
6. Final Testing Phase
Once the model has been selected based on its performance in validation tests it undergoes testing, using a test dataset to evaluate its real-world effectiveness.
7. Continuous Improvement
Refine the model design, feature engineering, and data preprocessing based on the results from the validation and test sets to enhance its performance. This ongoing process is the foundation of ML model creation.
Challenges Encountered During Validation
Data Quality and Availability
High-quality validation data is key to correct model performance. The problem with well-labeled validation data is that it’s complex to acquire and very expensive in fields such as healthcare or finance.
Data augmentation, anomaly detection, and the creation of synthetic data help in increasing not just the quantity but also the quality of data. Collaboration with data brokers and participation in data exchange programs also enhance the availability of data. Annotation services from domain experts can be used to reduce the count of errors and biases in the datasets.
Avoiding Overfitting to Validation Data
Regularly using validation data to tune the model may result in overfitting, where it exhibits good performance within the validation set, but has difficulties with the unseen data after deployment. This defeats the reason for having a validation set.
Cross-validation methods should be used to prevent overfitting of the validation data. In this method, the validation set is rotated systematically, allowing the model to be tested on various subsets of the data. Furthermore, the regularization methods, L1 and L2, could be adapted to manage the complexity of a model. Make sure that the model is not overly tuned to the specifics of the validation set – generalize the learning patterns and use early stopping during training.
Data drift
Changes in real-world data distribution over time can lead to "data drift," where models validated on historical data perform poorly on current data.
To address this issue, continuous monitoring and revalidation are necessary.
This shows that the data drift can be controlled, simultaneously raising early warning over declining model performances resulting from data distribution changes. A managed action system that includes concept drift management and adaptive learning can prevent data drifts.
Class Imbalance
Managing class distributions in datasets presents challenges during validation. The models may perform correctly while making predictions for the majority class but overlook characteristics of the minority classes, hence showing incorrect performance metrics.
Potential solutions could be data resampling to balance classes, using the minority over-sampling technique (SMOTE), and cost-sensitive learning, where minority classes are prioritized. These approaches help prevent bias towards the majority class.
Data Validation Tools
To ensure the accuracy, consistency, and reliability of datasets, especially in large-scale projects, data validation tools play a critical role. These tools help identify errors, enforce data quality standards, and streamline the process of detecting anomalies before the data is used for analysis or modeling.
Tool | Description | Key Advantages | Disadvantages |
---|---|---|---|
Astera | An end-to-end data integration and validation platform with a no-code interface, ideal for business users | - User-friendly UI (no-code) - Data profiling, cleansing, deduplication - Automates validation workflows - Connects easily to databases, cloud, and apps | - Less customizable for advanced users - Geared more toward small to mid-sized businesses |
Informatica | A powerful enterprise-grade data integration and validation suite with rich functionality for large-scale operations | - Advanced data quality and validation tools - Metadata and governance features - High scalability and performance - Strong support for data lineage and transformation | - High cost of licensing - Steeper learning curve - Heavier infrastructure requirements |
Talend | A flexible, open-source-based platform with strong cloud and big data support, offering both free and enterprise versions | - Open-source and cost-effective - Rich data profiling and validation features - Built-in real-time quality metrics - Strong in cloud and big data integrations | Community version lacks some enterprise features May require more configuration Performance may vary by setup |
OpenRefine | A free, open-source tool for cleaning and transforming messy data. Ideal for individual analysts and smaller datasets | - Great for quick data cleanup - Intuitive UI - Supports various file types | - Limited for large-scale/enterprise use - Fewer automation features - No real-time integration |
Ataccama ONE | An all-in-one platform for data quality, governance, and MDM (Master Data Management). AI-powered suggestions included | - AI-assisted data profiling - Supports large enterprises - Data governance integration | - Enterprise-oriented pricing - Requires onboarding/training - Not open source |
Dataedo | Primarily a data documentation tool, but includes features like data classification and validation rules | - Data catalog + validation - Great metadata explorer - Easy to collaborate with teams | - Focused more on documentation - Less validation automation - May require integration with other tools |
IBM InfoSphere QualityStage | Part of IBM’s data management suite, focused on cleansing, matching, and validating enterprise data | - Enterprise-ready - Deep cleansing and matching - Good for legacy systems | - Expensive and complex - Steep learning curve - Requires IBM ecosystem |
Apache Griffin | An open-source data quality framework for big data environments, especially for Hadoop/Spark | - Real-time data quality tracking - Scalable for large data lakes - Works well with big data pipelines | - Best for engineering-heavy teams - Limited UI and user support - May require customization |
Conclusion
Validation data is more than a checkpoint in machine learning development—it’s a critical component that ensures a model’s predictions aren’t just accurate, but dependable in real-world conditions. It provides a structured way to evaluate performance, tune hyperparameters, and avoid common pitfalls like overfitting or biased evaluation.
By carefully preparing and using validation datasets—whether through holdout methods, cross-validation, or specialized approaches for time-series and imbalanced data—practitioners can develop models that truly generalize beyond their training environments. Moreover, choosing the right tools and techniques to validate data at scale ensures consistency, quality, and long-term adaptability.
Ultimately, the strength of any machine learning model hinges on the quality of decisions made during development. And validation data plays a central role in those decisions, helping turn experimental code into production-ready intelligence.