3D Point Cloud Services
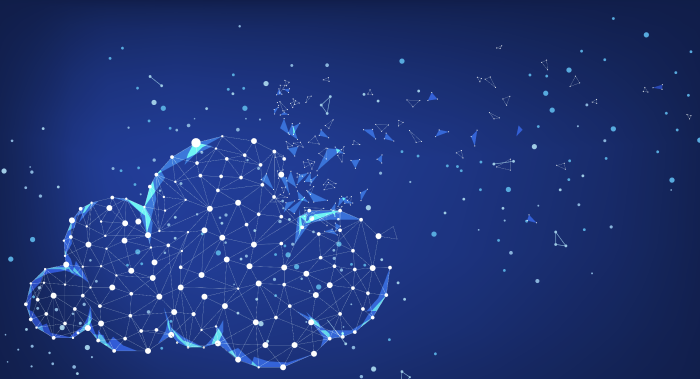
Unidata provides advanced 3D point cloud annotation services, specializing in meticulous labeling and tagging of 3D point cloud data to significantly improve object detection, scene understanding, and spatial analysis across a wide range of industries and applications.
Trusted by the world’s leading tech brands
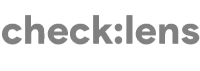
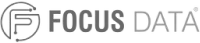
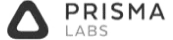
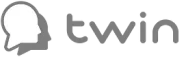
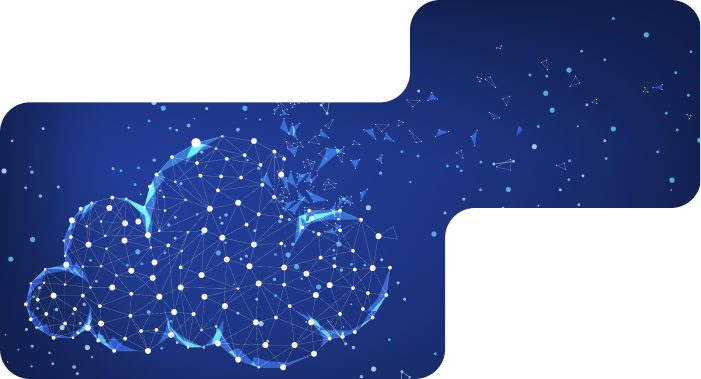
Advantages
SLA over projects
24/7*
24/7*
- 6+
- years experience with various projects
- 79%
- Extra growth for your company.
3D Point Cloud
What is 3D Point Cloud Annotation?
3D point cloud annotation is the process of labeling and tagging data collected from three-dimensional point clouds, which are representations of physical objects and environments created through technologies such as LIDAR, laser scanning, or photogrammetry. This specialized annotation involves identifying and marking key features, objects, and spatial relationships within the point cloud to enhance the understanding and interpretation of three-dimensional structures. By providing precise annotations—such as bounding boxes, semantic labels, and key points—3D point cloud annotation supports various applications, including autonomous vehicle navigation, robotics, urban planning, and virtual reality.How we deliver 3d point cloud services
The best software for 3d point cloud annotation tasks
Types of 3d point cloud annotation services
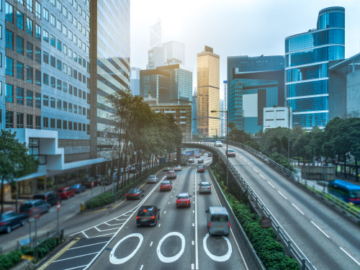
3D Bounding Box Annotation
This type of annotation involves drawing 3D bounding boxes around objects in a point cloud to define their spatial boundaries. Each box captures the height, width, and depth of the object, helping in object detection and classification tasks.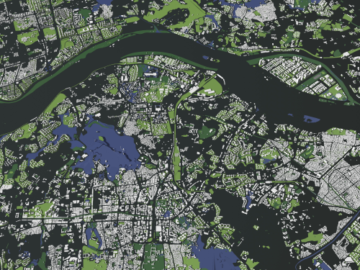
3D Semantic Segmentation
In 3D semantic segmentation, each point in the point cloud is labeled with a class corresponding to the object or surface it represents. This type of annotation is used to classify different parts of the environment or objects within a 3D space.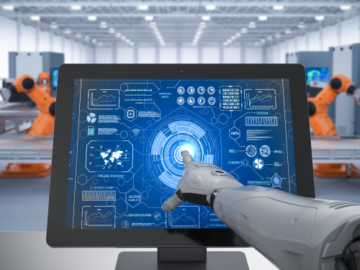
3D Instance Segmentation
Similar to semantic segmentation, instance segmentation goes further by not only labeling each point but also distinguishing between different instances of the same object class within a point cloud. Each instance is uniquely identified.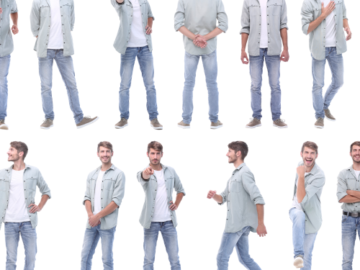
3D Object Tracking
3D object tracking involves identifying and following the movement of objects across multiple frames in a sequence of point clouds. This form of annotation tracks the object's position, orientation, and trajectory in 3D space.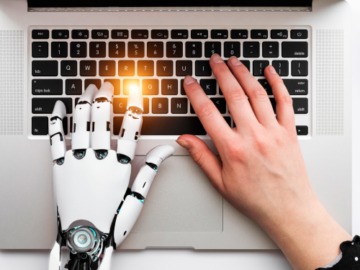
3D Keypoint Annotation
3D keypoint annotation involves marking specific points of interest on objects within a point cloud, such as corners, edges, or joints. These keypoints help in understanding the geometry and structure of objects in 3D space.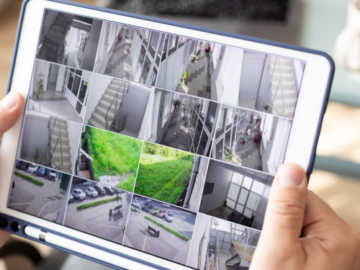
3D Lane and Road Marking Annotation
This annotation type is specifically used for marking lanes, road boundaries, and other relevant features in point clouds captured by LiDAR or other 3D sensors. It helps autonomous vehicles in navigating roads safely.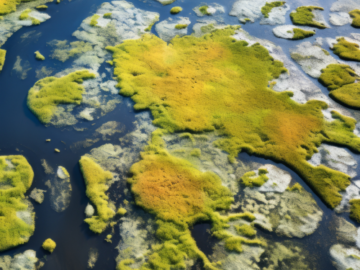
3D Plane and Surface Annotation
In this form of annotation, planar surfaces and other geometric shapes within a point cloud are identified and labeled. This can include walls, floors, and other large surfaces within a 3D environment.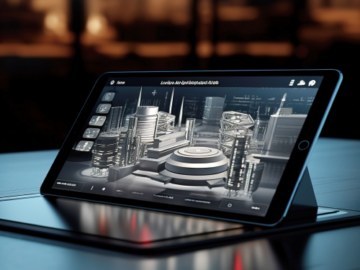
3D Volume Annotation
Volume annotation involves labeling volumetric regions within a point cloud. This is particularly useful in applications where understanding the full 3D shape and volume of objects or regions is important.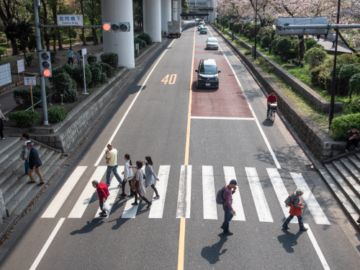
3D Object Classification
3D object classification involves labeling objects within a point cloud with specific classes. This type of annotation helps in recognizing and categorizing different objects based on their geometric shape and spatial orientation.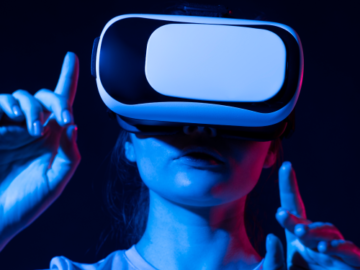
3D Environment Mapping
Environment mapping annotation is used to label and map out entire 3D environments within a point cloud, such as indoor spaces, urban areas, or natural landscapes. This helps in creating detailed 3D maps for navigation and simulation.3D Point Cloud Use Cases
-
01
Healthcare
In healthcare, 3D Point Cloud services are used for reconstructing anatomical models from CT scans or MRIs, providing detailed visualizations of organs and tissues. By converting medical imaging data into 3D Point Clouds, AI can assist in planning surgeries, diagnosing conditions, and monitoring patient recovery. The high-resolution data allows for more precise treatment decisions, improving patient care and surgical outcomes. -
02
Automotive (Autonomous Vehicles)
In the automotive industry, these services are essential for developing autonomous vehicles. By processing data from LiDAR and other sensors, AI can generate 3D Point Clouds that map the surrounding environment, including pedestrians, vehicles, and obstacles. These Point Clouds help AI systems navigate complex road environments, improving vehicle safety and decision-making in real-time, and allowing self-driving cars to avoid accidents. -
03
Construction & Architecture
In construction and architecture, it helps create accurate 3D models of buildings, construction sites, and infrastructure. By scanning physical structures with LiDAR, architects, and engineers can generate detailed Point Clouds that assist in planning, designing, and modifying buildings. These models ensure that construction projects adhere to specifications, improve planning, and enable more effective management of resources. -
04
Agriculture
In agriculture, 3D Point Cloud solutions help monitor crop health and land conditions by converting aerial images from drones or satellites into 3D Point Clouds. These Point Clouds provide a comprehensive view of fields, helping farmers assess the health of crops, detect diseases, and optimize irrigation systems. 3D models allow farmers to make data-driven decisions to improve crop yield, reduce resource waste, and manage farmland more efficiently. -
05
Real Estate
This technique is used to generate virtual property tours and detailed models of buildings. By capturing 3D data of properties, real estate agents can provide prospective buyers with immersive experiences, allowing them to explore homes remotely. 3D Point Clouds also assist in urban planning and architectural design, helping developers visualize the spatial layout of properties and surrounding areas. -
06
Security & Surveillance
In security and surveillance, these 3D services help generate detailed 3D models of environments, enhancing threat detection and monitoring. By scanning areas such as airports, stadiums, or industrial sites with LiDAR, AI systems can create Point Clouds that help security teams track movements and analyze potential threats. This technology improves real-time monitoring and response, providing more accurate and detailed surveillance footage. -
07
Manufacturing
Point Cloud is employed for quality control and equipment maintenance. By scanning products or machinery with LiDAR, manufacturers can create 3D Point Clouds that detect defects or wear and tear. This enables AI systems to identify production issues, automate inspection processes, and predict when machines will need maintenance, leading to improved product quality and minimized downtime. -
08
Entertainment & Media
3D Point Cloud services help create realistic 3D models for movies, video games, and virtual reality (VR) environments. By capturing real-world objects or scenes in high detail, AI can use Point Clouds to generate digital replicas for CGI (computer-generated imagery) and immersive experiences. 3D Point Cloud data enhances the realism of visual effects, making content more engaging for audiences.
Other Services
Ready to get started?
Tell us what you need — we’ll reply within 24h with a free estimate
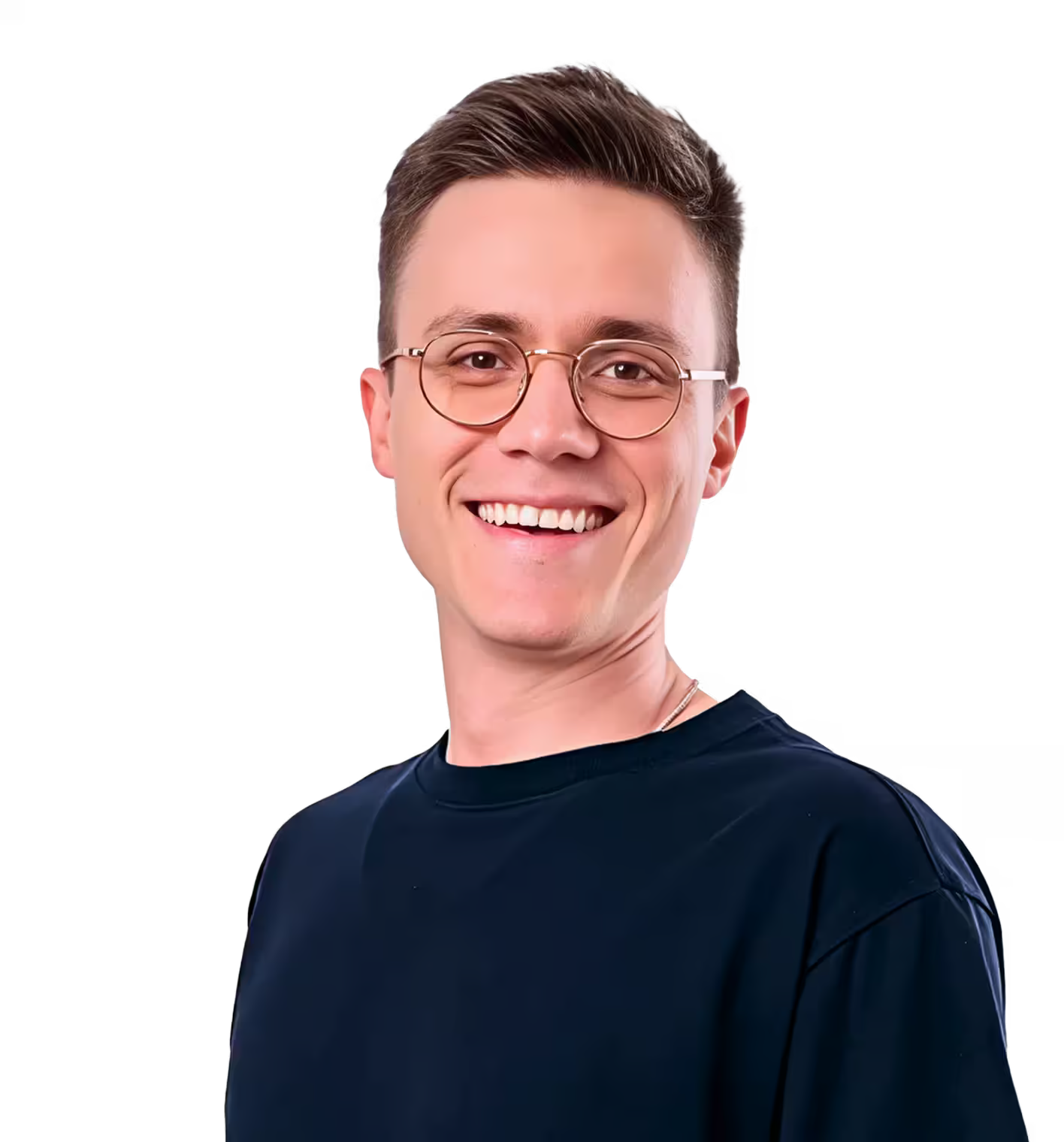
- Andrew
- Head of Client Success
— I'll guide you through every step, from your first
message to full project delivery
Thank you for your
message
We use cookies to enhance your experience, personalize content, ads, and analyze traffic. By clicking 'Accept All', you agree to our Cookie Policy.