Document Annotation Services
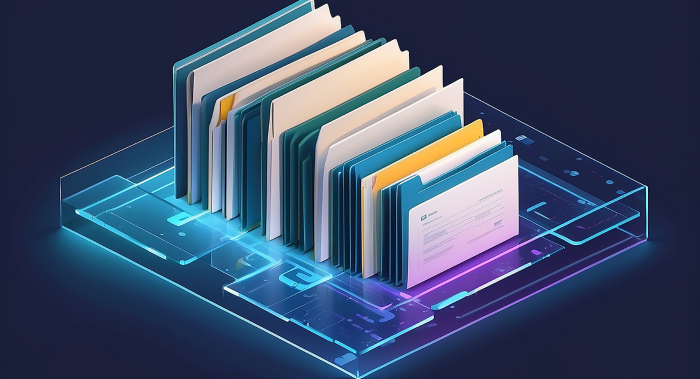
Unidata specializes in comprehensive document annotation services, providing precise labeling and tagging of textual documents to optimize information retrieval, improve document categorization, and enable in-depth content analysis across various industries and applications. Our meticulous approach ensures high-quality annotations that enhance the effectiveness of your data-driven projects
Trusted by the world’s leading tech brands
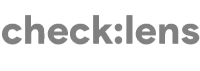
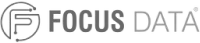
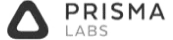
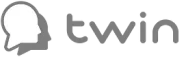
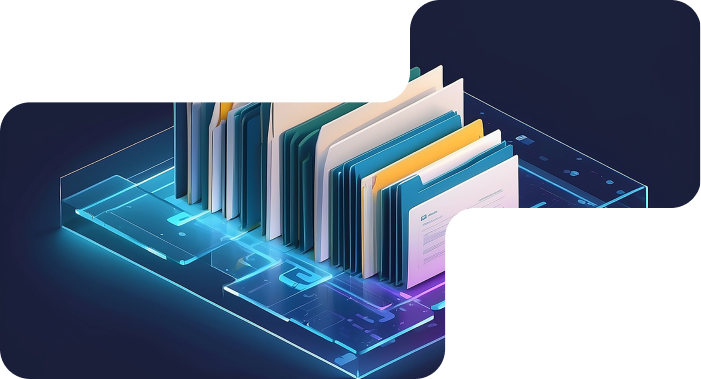
24/7*
- 6+
- years experience with various projects
- 79%
- Extra growth for your company.
What is Documents Annotation?
Document annotation is the process of systematically labeling and tagging elements within textual documents to enhance their usability and facilitate meaningful data extraction. This technique involves identifying and classifying various components, such as entities, topics, sentiments, and relationships, within the text, thereby transforming unstructured data into structured information. Document annotation is essential for applications like natural language processing (NLP), information retrieval, and content analysis, enabling organizations to improve search capabilities, automate categorization, and derive valuable insights from their data.How We Deliver Document Annotation Services
Software We Use for Document Annotation Services
Types of Document Annotation Services
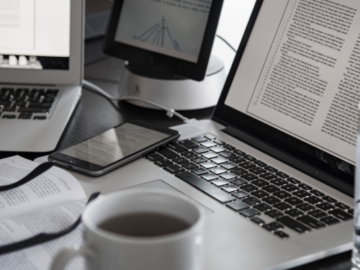
Text Classification
Text classification involves assigning predefined categories or labels to entire documents or sections of text. This service is commonly used for organizing and categorizing content like emails, legal documents, news articles, or research papers.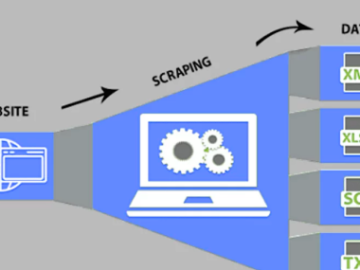
Named Entity Recognition (NER)
Named Entity Recognition focuses on identifying and labeling specific entities in a document, such as names of people, organizations, dates, locations, and other significant entities. This is often used in legal, financial, and healthcare documents to extract key information.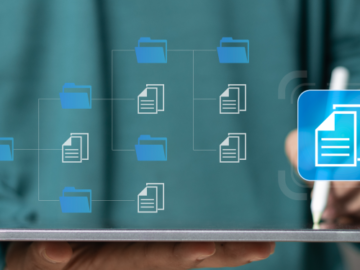
Sentiment Analysis
Sentiment analysis involves identifying and annotating the emotional tone or sentiment (positive, negative, or neutral) expressed within the text. It is commonly used in customer reviews, social media posts, and feedback analysis.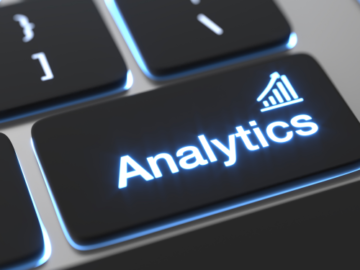
Document Segmentation
This service involves dividing a document into meaningful sections or segments, such as chapters, paragraphs, or sections of interest. It’s frequently used in long documents like contracts, manuals, or research papers to facilitate easier navigation and processing.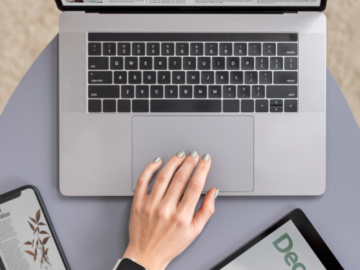
Content Labeling and Tagging
Content labeling and tagging assign specific labels to portions of text or entire documents based on subject matter, themes, or keywords. This is useful for indexing and search functionality within content management systems or digital libraries.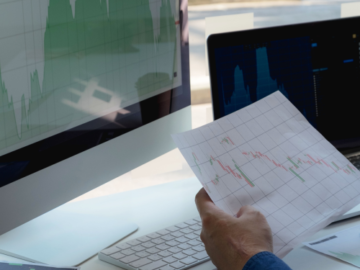
Key Phrase and Keyword Extraction
This service identifies and annotates important keywords or key phrases that summarize the main ideas or concepts within a document. It is useful for search engine optimization (SEO), content summarization, and topic identification.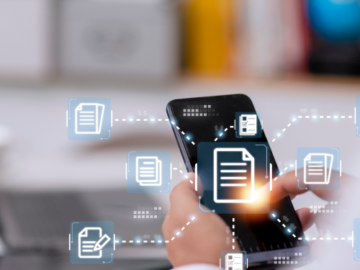
Semantic Role Labeling (SRL)
Semantic role labeling involves annotating the underlying meaning of sentences by identifying subjects, objects, verbs, and other key components. It is often used in natural language processing tasks like machine translation or information retrieval.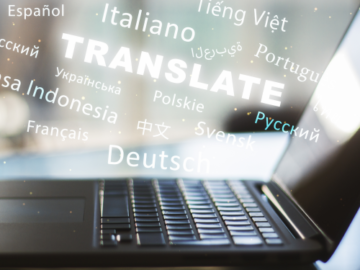
Optical Character Recognition (OCR) Annotation
OCR annotation involves annotating scanned documents or images of text to identify and label printed or handwritten text. This is widely used for converting scanned documents into editable and searchable formats.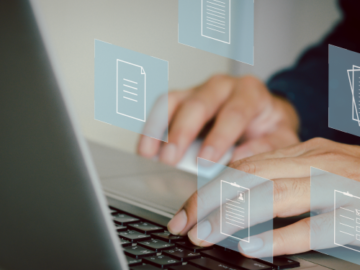
Table and Form Annotation
This type of annotation focuses on identifying and labeling tables, forms, or structured data within documents, often required for extracting financial statements, invoices, or other structured documents in tabular form.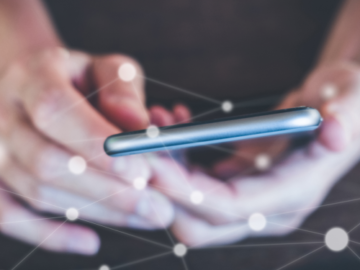
Summarization
Document summarization involves creating concise annotations that capture the core ideas or themes of a document. This is particularly useful for legal, academic, or technical documents where a quick overview is needed.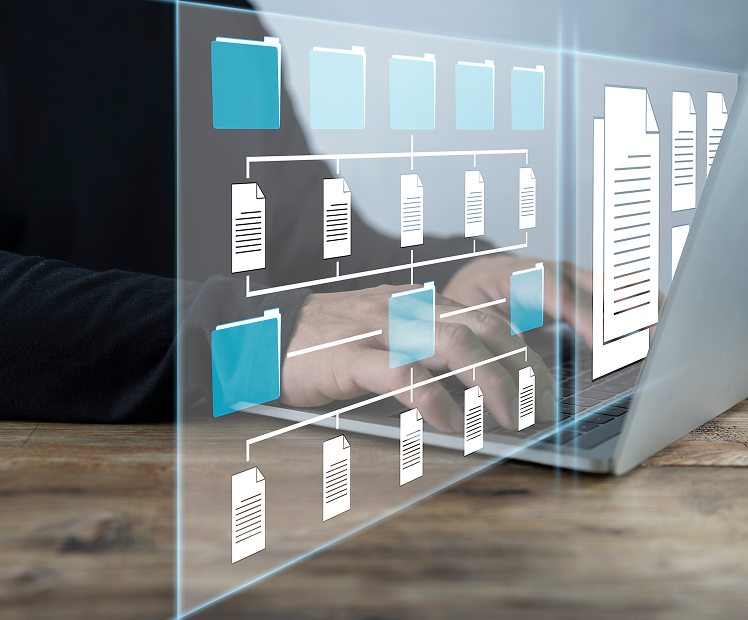
Metadata Annotation
Metadata annotation includes adding descriptive information to documents, such as authorship, creation date, file type, and other relevant data. This is especially useful for digital asset management and archival purposes.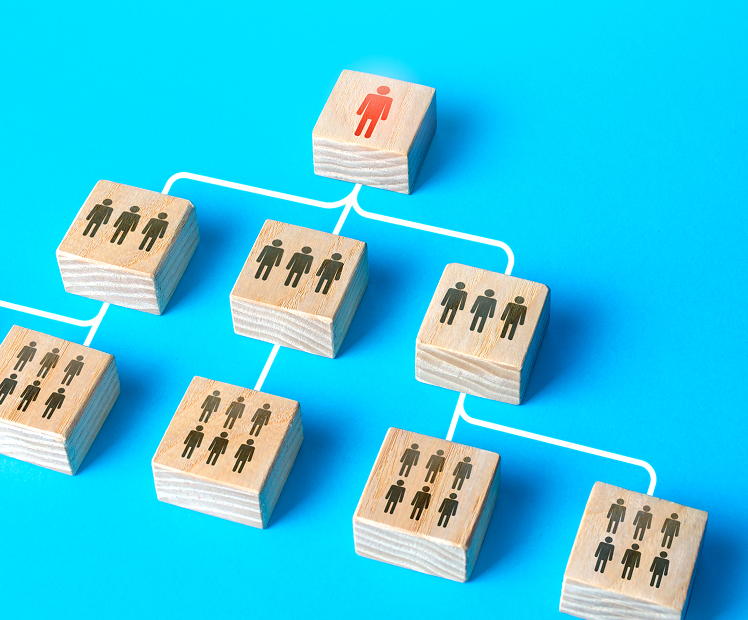
Relation Extraction
This service involves identifying and annotating relationships between entities within a document, such as connections between people, organizations, or events. It is often used in research, journalism, or investigative reporting.Document Annotation Use Cases
-
01
Healthcare
In healthcare, document annotation is used to label key information in medical records, clinical notes, and research papers. By annotating text with details like symptoms, diagnoses, and treatment plans, AI can help doctors and medical staff quickly find relevant information, improving decision-making. This process is also crucial for organizing patient histories and enabling more efficient care coordination. -
02
Legal
In the legal industry, annotation helps lawyers and paralegals organize case files, contracts, and court rulings. By annotating legal documents with key clauses, definitions, and references to case law, AI can quickly identify relevant legal precedents and terms. Annotated contracts also help automate the review process, improving efficiency and reducing the time spent searching through lengthy legal documents. -
03
Finance
This service is essential for organizing and analyzing financial reports, investment analyses, and transaction records. By labeling key information such as financial figures, investment terms, and customer data, AI can assist in quickly extracting important insights. It also plays a role in identifying potential fraud by annotating transaction histories and flagging unusual patterns. -
04
Education
Annotation is used to help organize and categorize educational materials, such as textbooks, research papers, and study guides. By labeling key concepts, definitions, and explanations, AI systems can help students locate and access information more easily. Annotated educational content also allows for personalized learning experiences, enabling tailored study tools based on individual needs. -
05
Retail & E-commerce
In retail and e-commerce, it is applied to product descriptions, customer reviews, and sales data. By labeling information related to product features, customer feedback, and transaction history, AI can enhance product searches and recommendations. Annotating customer service interactions helps improve the accuracy of chatbots, allowing for better customer support and faster responses. -
06
Manufacturing
This technology helps improve quality control and inventory management. By labeling documents such as maintenance logs, production reports, and inspection checklists, AI systems can quickly identify issues or patterns that might affect production. Annotating manuals and technical documents also help workers find important information faster, improving the efficiency of maintenance and repair tasks. -
07
Security & Surveillance
In security and surveillance, document annotation is used to label security reports, incident logs, and surveillance footage. By annotating these documents with key information such as timestamps, individuals involved, and potential threats, AI can help security teams quickly identify and address issues. Annotated reports also make it easier to track incidents and analyze trends over time, enhancing overall security management. -
08
Marketing
In the marketing industry, tagging is used to label customer feedback, campaign reports, and market research. By tagging important data like customer preferences, purchase behavior, and advertising effectiveness, AI can help marketers identify trends and optimize campaigns. Annotating competitive analysis documents also provides valuable insights into market positioning and consumer behavior.
Other Services
Ready to get started?
Tell us what you need — we’ll reply within 24h with a free estimate
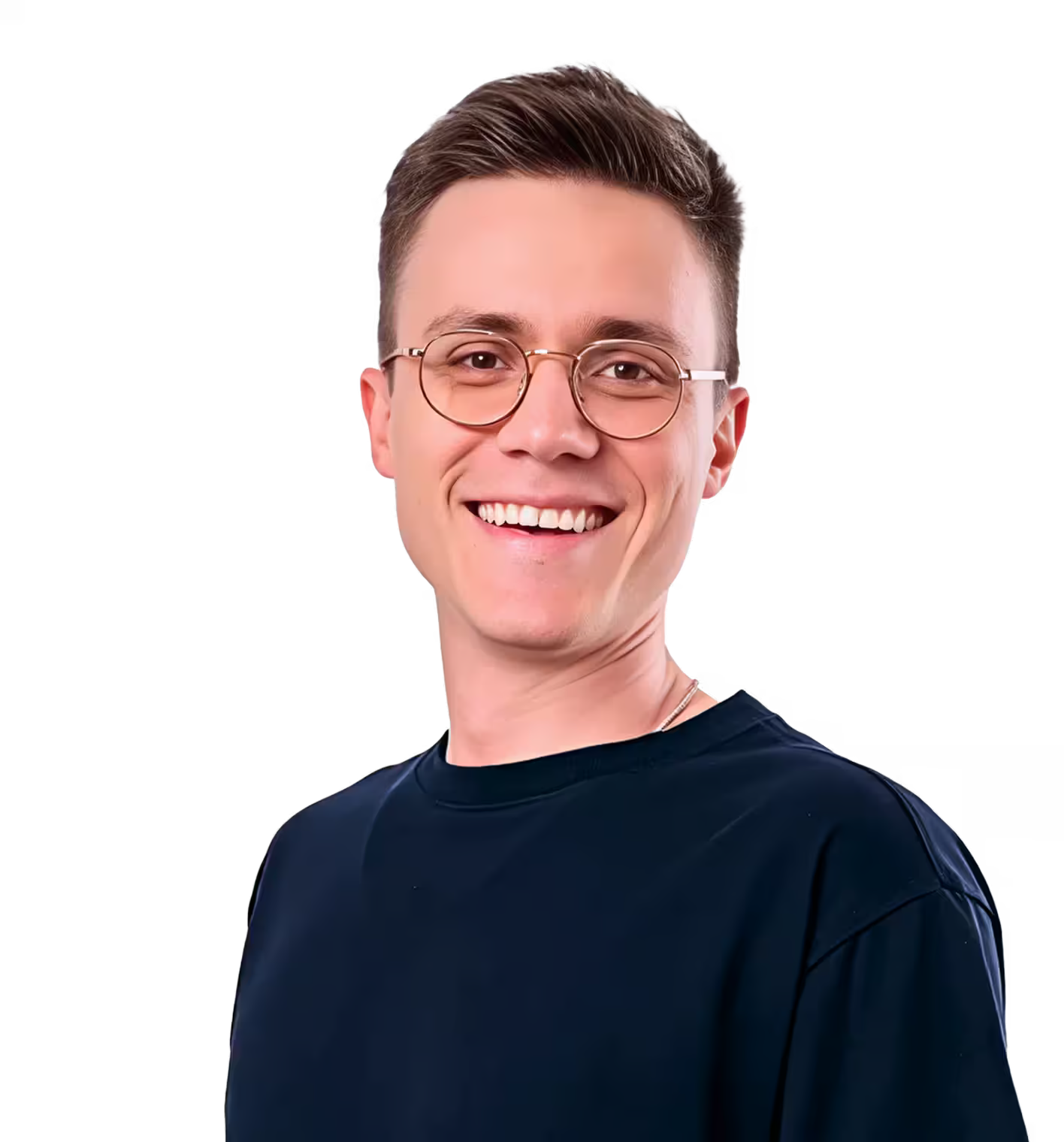
- Andrew
- Head of Client Success
— I'll guide you through every step, from your first
message to full project delivery
Thank you for your
message
We use cookies to enhance your experience, personalize content, ads, and analyze traffic. By clicking 'Accept All', you agree to our Cookie Policy.