1. Introduction: Why POS Tagging Still Matters in the Age of LLMs
Language is alive. It breathes, evolves, and resists being stuffed into tidy boxes. Yet to build machines that understand language, we need some structure. That’s where POS (parts-of-speech) tagging comes in. Think of it like labeling all the parts of a sentence so a machine knows who’s doing what to whom.
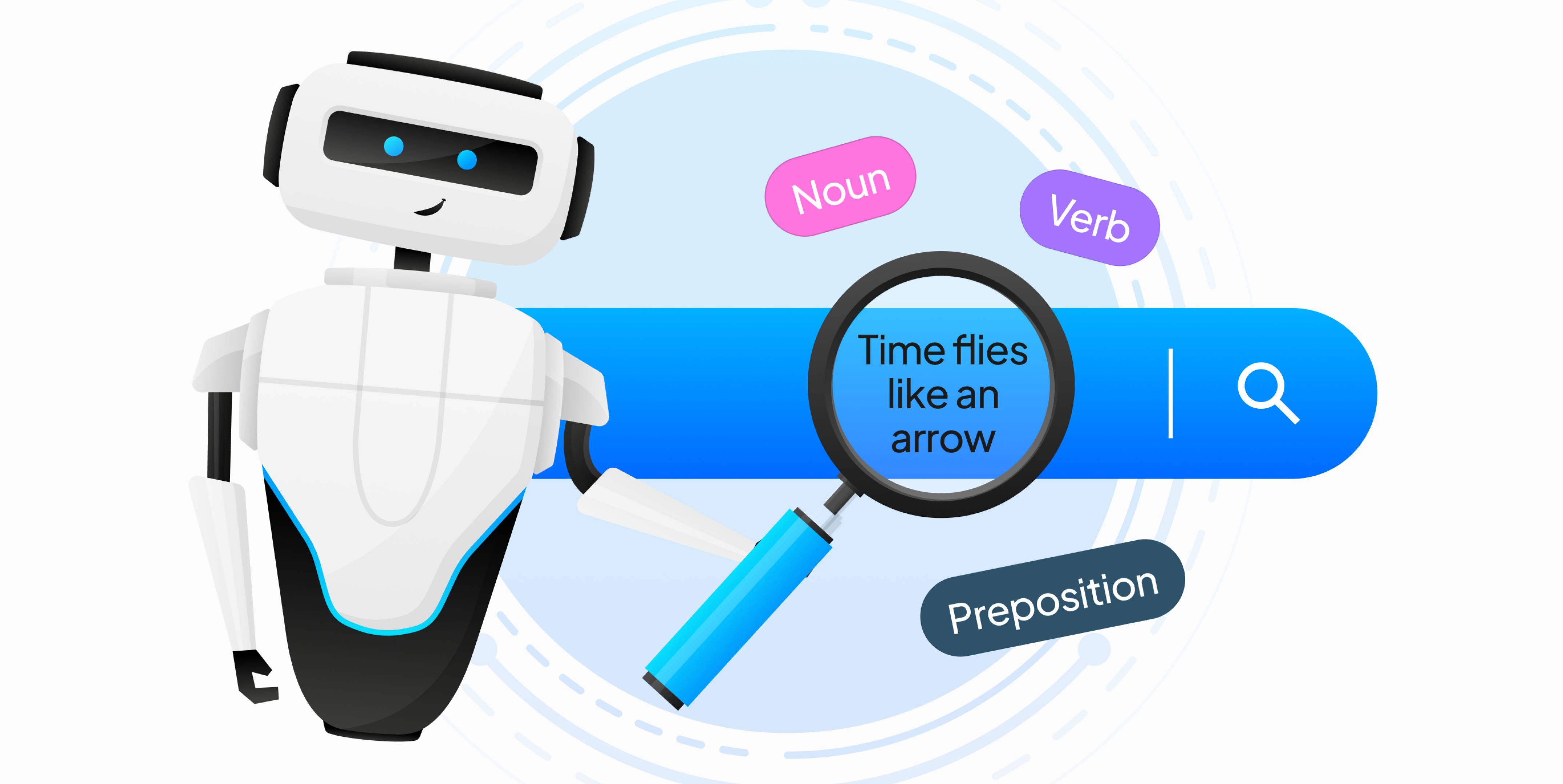
In a world obsessed with large language models (LLMs), POS tagging might sound a bit... vintage. But don’t be fooled. It’s the skeleton that supports a lot of modern NLP muscle. From improving syntactic parsing to powering grammar checkers, voice assistants, and translation engines, POS tagging continues to punch above its weight.
And here’s a fun stat: according to a 2023 survey by NLP-progress.com, over 60% of foundational NLP pipelines still include POS tagging as a preprocessing step—even in deep learning workflows.
2. What is POS Tagging? An Intuitive Primer
Parts-of-speech tagging, or POS tagging, is the task of labeling each word in a sentence with its part of speech. In simple terms, this means figuring out if a word is a noun, verb, adjective, adverb, pronoun, or something else. These labels help computers make sense of how words fit together in a sentence.
Imagine text as a jumbled puzzle. POS tags help machines organize that puzzle by showing how words relate to each other. For example, if a word is marked as an adjective, like "quick," it probably describes a noun. If it’s tagged as a verb, like "run," it shows an action. This kind of structure helps machines understand sentences more like humans do.
Let’s look at a classic example: "Time flies like an arrow."
- Is "flies" a noun (as in insects) or a verb (as in flying)?
- Is "like" a verb (as in enjoy) or a preposition (as in similar to)?
This sentence is tricky. Without context, it could mean different things. POS tagging helps clear up this confusion. In this sentence, "flies" is most likely a verb, and "like" is a preposition. POS taggers use patterns and context to figure that out.
Modern systems don’t just check dictionaries. They look at nearby words, sentence structure, and common usage patterns. A statistical or neural model might see "flies like an arrow" and recognize that in similar cases, "flies" acts as a verb. This kind of learning helps machines understand language more accurately.
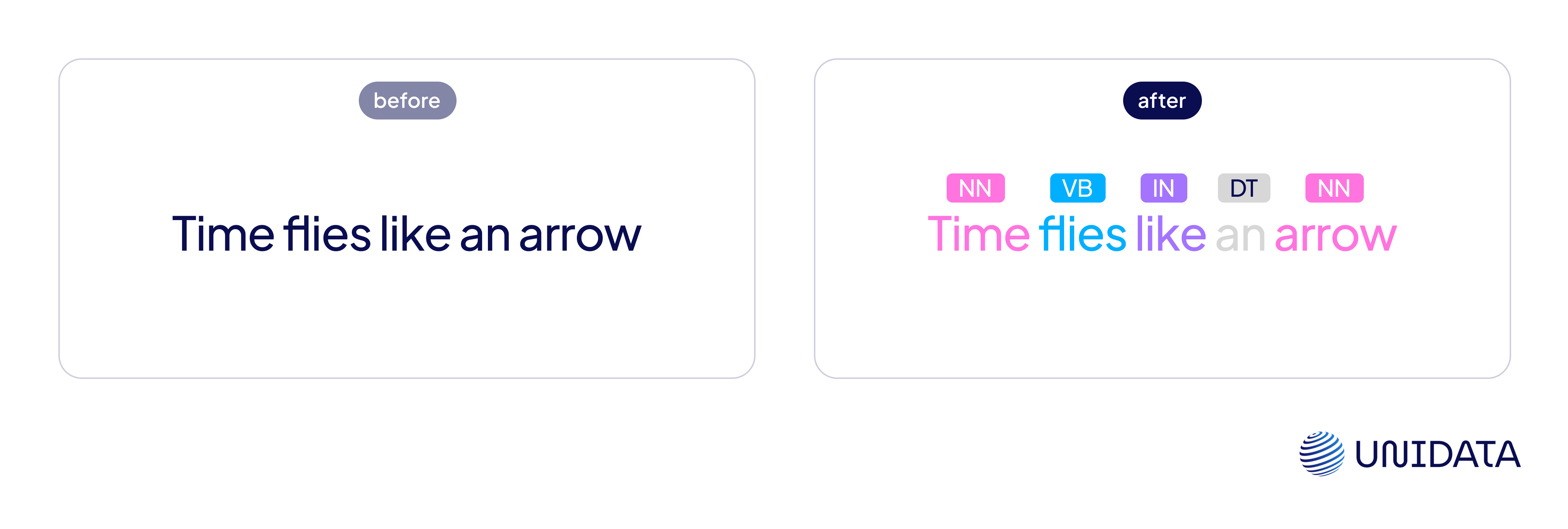
3. A Quick History: From Rule-Based to Neural Networks
Before we had attention mechanisms and transformers, NLP folks built grammars by hand. Rule-based taggers were painstakingly written, usually requiring extensive linguistic expertise.
Then came statistical models in the late 80s and 90s. Hidden Markov Models (HMMs) and Conditional Random Fields (CRFs) could learn from labeled corpora and handle probabilistic transitions between tags.
Now? We’re in the era of neural tagging. BiLSTM and Transformer-based models (like BERT) have largely outpaced their predecessors in accuracy and contextual sensitivity.
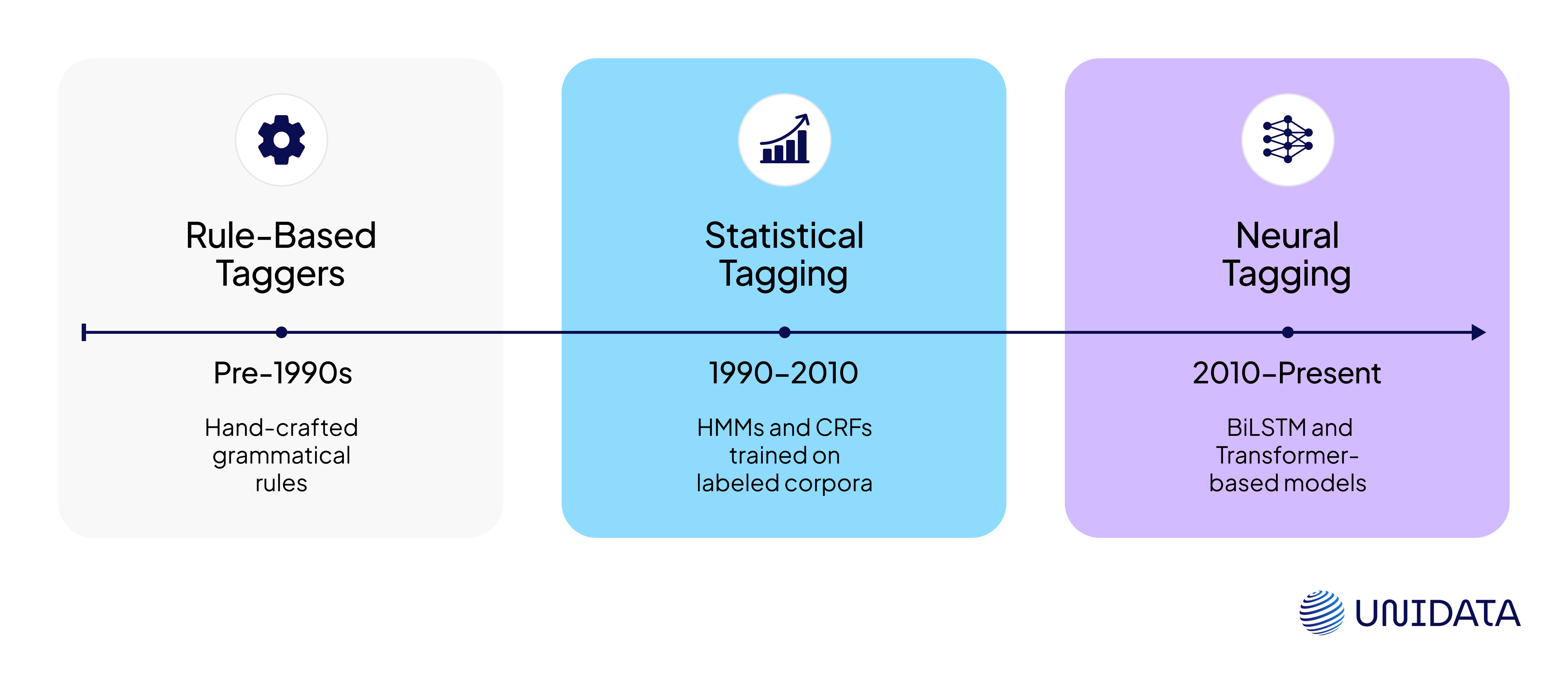
4. POS Categories Explained
A tag is only as helpful as the label behind it. Here are the major POS categories used in the Penn Treebank tagset:
Tag | Description | Example Words |
---|---|---|
NN | Noun (singular) | cat, tree, algorithm |
NNS | Noun (plural) | cats, ideas, datasets |
VB | Verb (base form) | run, eat, predict |
VBD | Verb (past tense) | ran, ate, predicted |
JJ | Adjective | red, quick, efficient |
RB | Adverb | quickly, well, always |
IN | Preposition | in, on, of |
DT | Determiner | a, the, some |
Different tagsets (e.g., Universal POS Tagset, Brown Corpus) vary slightly, but the idea remains: tell the model what grammatical role each word plays.
Workflow of POS Tagging in NLP
Before we get into the tagging methods, let’s walk through how POS tagging actually works in a typical NLP pipeline. Think of it as a series of steps that transform raw, messy text into a structured format with helpful labels.
1. Text Preprocessing
Tokenization: First, we split the input text into smaller units called tokens. These are usually words or punctuation marks.
Normalization: Then, we clean up the tokens. This may include converting text to lowercase, removing special characters, or adjusting punctuation to make things more consistent.
2. Preparing the Input for the Model
We can’t feed plain words into a machine. Tokens must be converted into numbers or vectors. This step might use word embeddings, one-hot encoding, or break words into smaller parts called subwords (used in models like BERT).
3. Running the POS Tagging Model
This is where the magic happens. We use a tagging model—could be rule-based, statistical, or neural—to assign a POS tag to each token. Every word gets labeled based on how it's used in the sentence.
4. Postprocessing the Output
Once tagging is done, we might clean up or fix any mistakes. Sometimes we apply extra rules or adjust tags to fit a specific tagging standard.
5. Putting It to Use
Finally, the tagged text is passed into other NLP tools. These could include dependency parsers, named entity recognizers, or translation systems. POS tags make their jobs easier and more accurate.
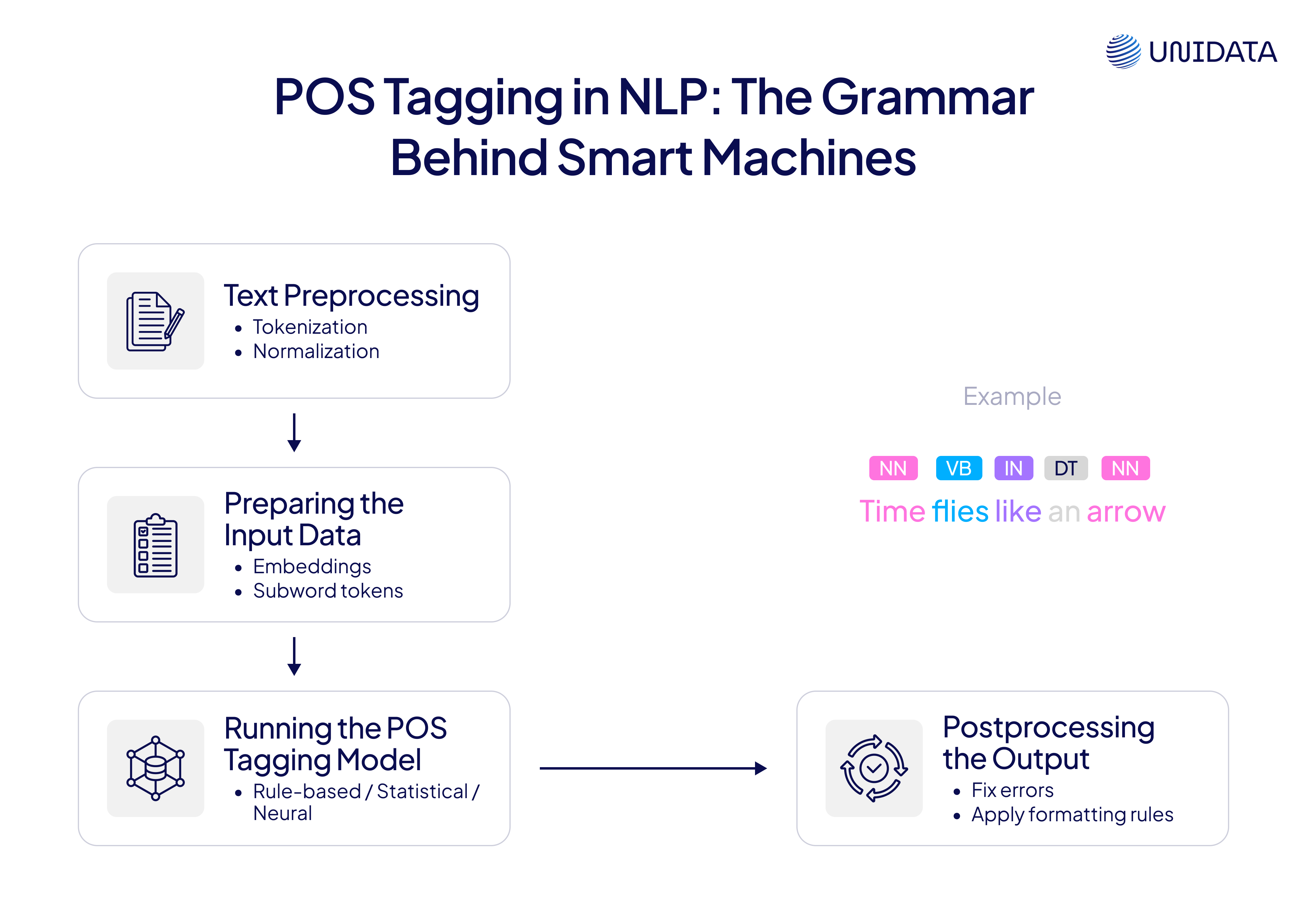
5. Methods of POS Tagging
Rule-Based Tagging
The OG approach. It uses hand-written rules to decide the tag of each word. These rules look at things like how a word ends or what kind of word comes before or after it.
For example, if a word ends in "-ly," it’s probably an adverb. If it follows a noun and describes it, it might be an adjective.
Rule-Based Tagging is easy to understand. You can also edit the rules to suit your domain. However, this method doesn’t work well with new or unknown data. Writing and updating rules is time-consuming.
Transformation-Based Tagging
Also known as Brill Tagging, this method sits between rule-based and statistical models. It starts with simple tagging—often based on word frequency or default rules—and then improves results by applying transformation rules.
Here’s how it works:
First, assign initial tags using basic guesses or a lexicon.
Then, go through the tagged text and fix errors using learned rules. For example, change a verb to a noun if it's followed by a determiner.
These rules aren’t manually written. They’re learned from training data. The model figures out which transformations improve accuracy the most and applies them in sequence.
As for the pros, this method is easier to understand than statistical models and it learns rules directly from data.
However, Transformation-based tagging is llower at runtime than some other methods and less accurate than modern neural models.
This method was popular in the 90s and was used in early POS taggers like the one created by Eric Brill. It’s not state-of-the-art today, but it’s still useful for teaching and smaller projects.
Statistical Tagging
As data grew, so did smarter models. Statistical methods came next. These models don’t rely on rules—they learn from real examples in labeled datasets.
- HMM (Hidden Markov Model): Predicts the most likely tag for a word based on how often it appears and what tags usually come before or after it.
- CRF (Conditional Random Field): A stronger model that looks at the whole sentence to decide tags. It can use more features and spot patterns HMMs miss.
These models learn from corpora like the Penn Treebank. The more data they see, the better they get.
Neural Approaches
Deep learning changed the game. Neural models learn word meanings and patterns from huge datasets without hand-coding.
- BiLSTM: A type of neural network that reads the sentence both forward and backward. This helps it understand context from both sides.
- Transformer-based models: These include BERT, RoBERTa, and XLM-R. They use self-attention to understand how words relate across the whole sentence. With fine-tuning, they can predict POS tags with high accuracy.
Neural models now lead the field. They outperform older methods by 2–5% on most benchmarks (source: ACL Anthology 2022). They’re powerful, flexible, and adapt well to different languages and domains.
6. Key Challenges in POS Tagging and Advantages
Even though POS tagging has come a long way, it still faces real challenges. Let’s break down a few of the biggest ones:
Ambiguity: Some words can mean different things in different sentences. For example, “can” could be a container or a way to express ability. Models need to figure that out using context, and it’s not always easy.
Domain Shift: A model trained on news articles might struggle with tweets, legal contracts, or medical notes. The vocabulary, tone, and grammar all change, and models often don’t adapt well across domains.
Multiword Expressions: Phrases like “kick the bucket” don’t mean what they literally say. Models that tag word-by-word can miss the real meaning.
Code-Switching: People often mix languages, especially in social media. A sentence might start in English and end in Spanish. That’s tough for monolingual models to handle.
Even state-of-the-art (SOTA) models still trip up when text is noisy, informal, or from an unexpected source.
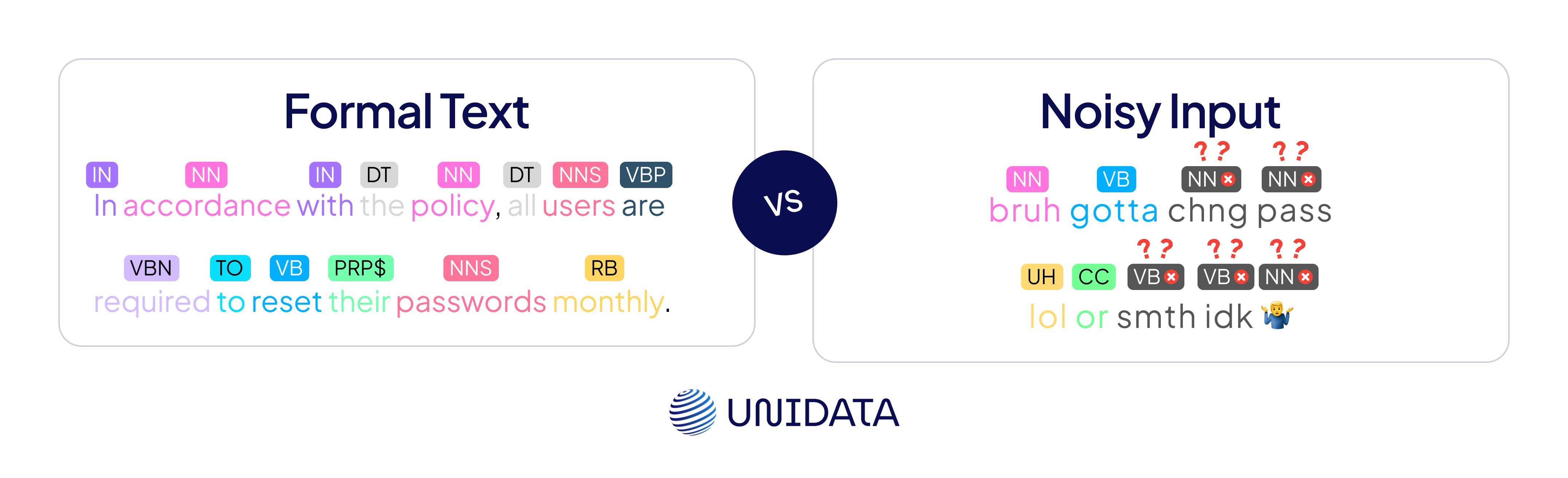
7. POS Tagging in Multilingual NLP
Most of the world doesn’t speak English, and that creates both a challenge and an opportunity. Every language has its own grammar rules. Some don’t even have spaces between words. Others use different word orders entirely.
Universal POS tagsets try to bring some order to the chaos. They offer a shared set of tags that work across languages. Projects like Universal Dependencies help researchers use consistent labels in over 100 languages.
Multilingual models like mBERT and XLM-R are a big step forward. They can learn from many languages at once and transfer knowledge from high-resource to low-resource ones. But some languages still don’t have enough training data, so the models don’t perform as well.
8. Tools and Libraries You Can Use
Want to try POS tagging? Here are the top libraries:
Tool | Language Support | Method | Notes |
---|---|---|---|
NLTK | English | Rule + HMM | Good for learning |
spaCy | 60+ languages | CNN | Fast and production-ready |
Stanza | 66 languages | BiLSTM | Stanford's official NLP suite |
Flair | Multilingual | Embeddings + BiLSTM | Flexible and easy to use |
AllenNLP | English | Transformer | Research-grade models |
9. Final Thoughts: Future Trends and Takeaways
POS tagging isn’t going out of style. It’s evolving. Expect to see:
- Task-unified tagging: Joint models that do POS + NER + parsing.
- Prompt-based tagging: Using zero-shot LLMs with smart prompts.
- Greater multilingual expansion: Including endangered and dialect-rich languages.
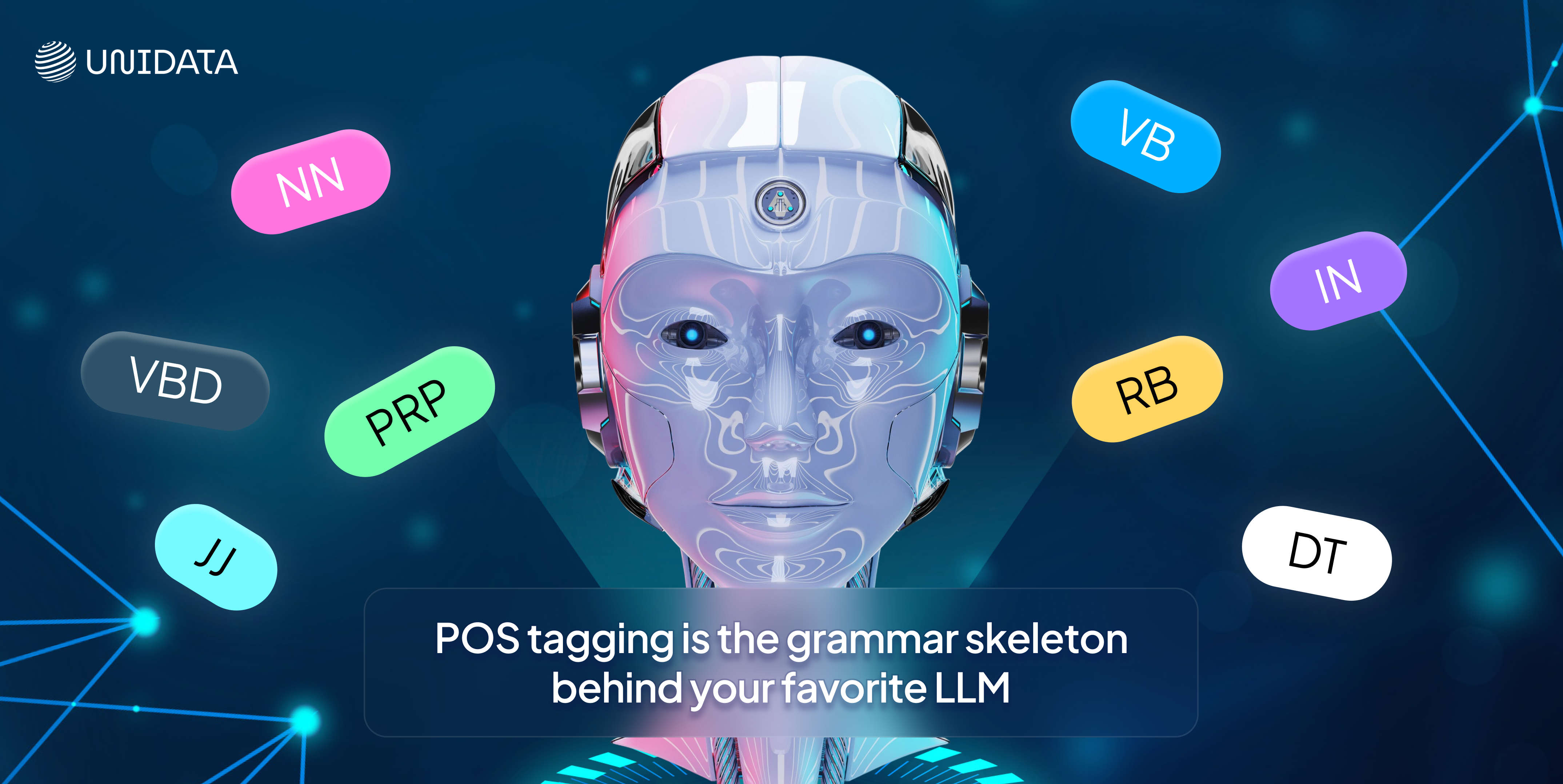
In short: POS tagging still has plenty of runway. It might be behind the curtain, but it’s part of the magic show that makes NLP feel like... well, language.
And the next time you hear "POS tagging," don’t think of it as tagging just nouns and verbs. Think of it as the grammar backbone keeping your favorite AI assistant from sounding like a confused toddler.
Further Reading & References: