iBeta Certification Definition
Imagine a world where your face, voice, or fingerprint unlocks everything from your phone to your bank account and home. Biometric technology is transforming security, but also attracting cybercriminals. How can you ensure your digital identity is safe? iBeta Certification is the solution. It not only ensures compliance but identifies vulnerabilities, strengthening systems against advanced attacks.
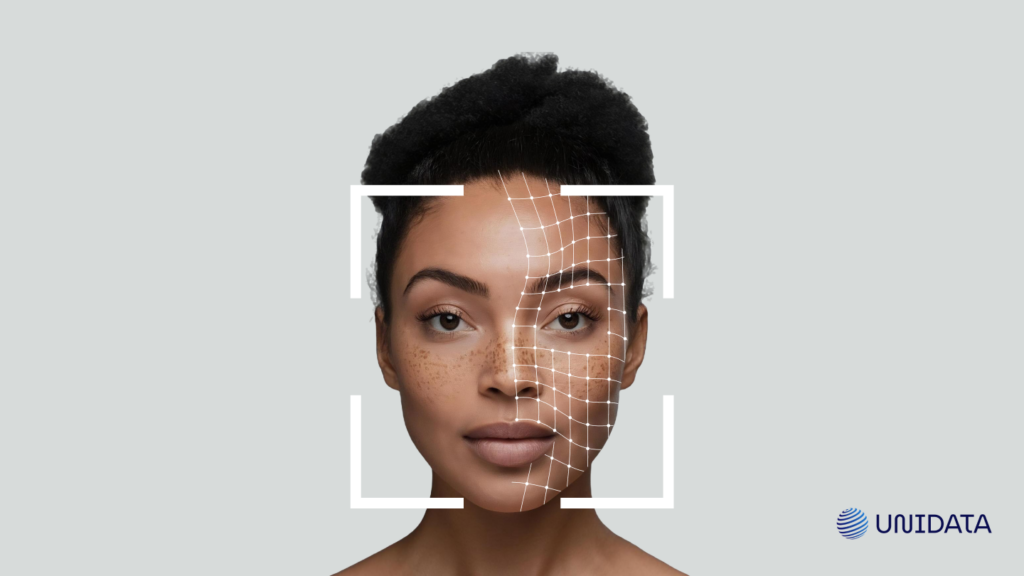
This article explores how iBeta is setting a new standard for biometric security and digital trust.
iBeta distinguishes itself from other certification bodies through its depth of expertise and adherence to the highest international standards. However, which components make iBeta Certification unique? Let us explore this in this article.
Why iBeta Certification?
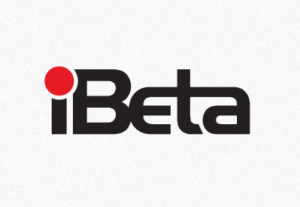
iBeta is a global leader in biometric system testing and certification, known for its rigorous and comprehensive evaluation processes. Choosing iBeta Biometric Certification ensures that the biometric technology is not only compliant with international standards but also robust against real-world threats and sophisticated attacks. Here are the key reasons to choose iBeta for biometric certification:
Global Recognition and NVLAP Accreditation
iBeta is accredited by the National Voluntary Laboratory Accreditation Program (NVLAP), which operates under NIST (National Institute of Standards and Technology). This accreditation means that iBeta’s testing and certification results are recognized worldwide and comply with ISO/IEC 17025 for laboratory competence.
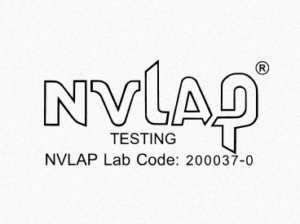
Certifications issued by iBeta hold significant weight across industries like finance, healthcare, and government sectors, ensuring global trust and acceptance of the biometric systems.
Compliance with International Standards (ISO/IEC 30107)
iBeta’s certification process strictly adheres to the ISO/IEC 30107 standard for Presentation Attack Detection (PAD), which is the benchmark for ensuring that biometric systems are secure against spoofing attacks.
With iBeta’s certification, the system demonstrates compliance with critical security standards, which is increasingly essential for meeting regulations like GDPR in Europe, CCPA in California, and industry-specific standards.
Comprehensive and Real-World Testing
iBeta’s testing procedures go beyond standard evaluations by simulating real-world attacks and challenging environments to ensure your system performs reliably in practical scenarios. The certification process includes:
- Liveness detection testing to ensure the system can distinguish between live users and spoofed inputs (e.g., photos, masks, or synthetic fingerprints).
- Environmental stress testing to assess the system’s reliability under different lighting conditions, extreme temperatures, and humidity levels, ensuring robustness across diverse user environments.
- Performance metrics such as False Acceptance Rate (FAR) and False Rejection Rate (FRR) testing, which balance security with user convenience, guaranteeing both security and user-friendliness.
Advanced Threat Mitigation (PAD Testing)
iBeta is a leader in Presentation Attack Detection (PAD), where it tests the system's ability to detect and resist spoofing techniques such as high-quality masks, fake fingerprints, or deepfake videos. This is critical as biometric spoofing methods become more advanced.
iBeta tests both Level 1 (basic) and Level 2 (sophisticated) attacks, ensuring that the biometric system can withstand even the most advanced hacking attempts, providing unmatched security for high-stakes applications like banking and government identification.
Wide Modalities and Multimodal Testing
iBeta offers certification for a broad range of biometric modalities, including:
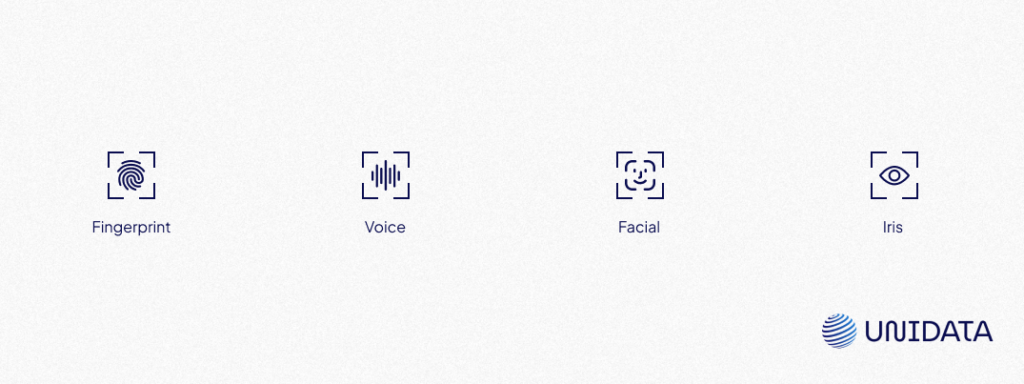
It also evaluates multimodal systems, where two or more biometric types (e.g., facial and fingerprint recognition) are used together. This ensures that all aspects of the biometric solution are tested thoroughly, providing a complete and validated assessment of security and usability.
Trusted Across Critical Industries
iBeta certifications are relied upon in highly sensitive sectors where security and accuracy are paramount, including:
- Finance: Protecting against identity fraud in mobile banking, ATMs, and payment authentication.
- Healthcare: Safeguarding patient data and ensuring secure access to medical records.
- Government: Ensuring secure biometric authentication for passports, national IDs, and border control.
- Telecommunications: Securing mobile device unlocking and carrier services.
Data Privacy and Regulatory Compliance
iBeta’s certification process ensures that biometric systems comply with stringent data privacy regulations like the General Data Protection Regulation (GDPR) and California Consumer Privacy Act (CCPA).
iBeta ensures that biometric systems implement secure data handling, encryption, and user consent mechanisms, which are critical for privacy-focused industries such as healthcare and financial services.
With iBeta certification, your biometric system meets the latest compliance requirements, reducing the risk of penalties or legal challenges due to non-compliance.
Tailored Testing for Specific Needs
iBeta offers customizable testing solutions tailored to the unique security, operational, and regulatory requirements of the biometric system. Whether your system operates in extreme environments, integrates multiple biometric modalities, or faces particular security threats, iBeta’s testing is designed to meet your specific needs.
This tailored approach ensures that the certification is relevant and comprehensive, giving the stakeholders confidence in the system’s performance in real-world conditions.
Independent, Impartial Testing
iBeta operates independently and is not affiliated with any biometric vendor or technology provider. This ensures that testing is conducted with impartiality, focusing solely on the system’s ability to meet technical and security standards without any conflicts of interest.
This independence ensures unbiased results, giving the credible, third-party validation of the biometric system’s capabilities.
Recertification and Ongoing Testing
iBeta offers ongoing testing and recertification services to ensure that the biometric system remains secure against emerging threats and technological advancements. As spoofing techniques and attack vectors evolve, regular testing ensures that the system stays ahead of potential vulnerabilities.
This continuous validation process helps the biometric solution remain secure and compliant over time, protecting it from becoming outdated or compromised.
Enhanced Market Trust and Confidence
Gaining iBeta Biometric Certification enhances the system’s credibility and instills confidence among end-users, customers, and stakeholders. Certified systems demonstrate a proven level of security and performance, which can serve as a differentiator in competitive markets, particularly in industries where security is paramount.
Certification from iBeta can also streamline adoption by regulatory agencies and industry bodies, accelerating the approval process for launching biometric technologies.
How to pass iBeta?
Achieving iBeta Certification under ISO/IEC 30107-3 is a multi-step journey requiring robust preparation, precise execution, and strategic refinement. This guide provides detailed insights into each phase of the certification process, drawing from real-world examples, advanced research, and actionable methodologies to help biometric systems meet the rigorous requirements of iBeta Certification. iBeta offers Liveness Detection and Biometric Systems testing. Liveness detection is an integral component of biometric systems, ensuring that only real, live users can access protected systems.
This certification evaluates a system’s ability to resist spoofing attempts like static photos, videos, or even sophisticated artefacts like 3D masks. iBeta provides different certification levels to validate liveness detection for varying application needs.
Level | Example PAIs | Use Case | Challenge |
---|---|---|---|
Level 1 | Printed images, basic masks | Entry-level security (e.g., apps) | Limited variability |
Level 2 | Silicone masks, resin props | Banking, healthcare | More sophisticated attacks |
iBeta Level 1
Level 1 Liveness Detection certification focuses on detecting basic presentation attacks, such as photos, videos, or printed masks. The goal is to ensure that the biometric system can reliably distinguish between a live user and static, easily replicated artifacts. This certification is essential for applications like smartphones for secure device unlocking, where security needs are lower, or entry-level authentication systems that deal with less sensitive information.
The testing methods for Level 1 include motion analysis, where natural movements like blinking or slight head tilts are detected to confirm a live user. Texture analysis is also used to identify surface patterns that distinguish skin from artifacts, while reflection detection checks for inconsistencies in photos or screens that would indicate a spoofing attempt.
iBeta Level 2
Level 2 Liveness Detection certification takes a step further by addressing more advanced spoofing attempts, such as 3D masks or deepfake videos. At this level, the biometric system must show enhanced resistance to more complex and engineered attacks while ensuring it remains user-friendly. This certification is crucial for high-security applications such as access control at sensitive facilities or online banking and healthcare, where the protection of financial or medical data is paramount.
Testing for Level 2 certification involves more sophisticated techniques. Depth analysis using infrared or stereoscopic imaging helps verify the 3D characteristics of a live face, while advanced motion detection tracks subtle micro-movements or gestures to confirm liveness. Additionally, AI-powered texture recognition leverages machine learning models to analyze skin micro-patterns and detect potential artifacts.
iBeta Level 3
Currently, Level 3 iBeta PAD Certification does not officially exist within the ISO/IEC 30107-3 framework. While Level 3 is often referenced conceptually as the next frontier of advanced testing, there is no formalized Level 3 certification process offered by iBeta or defined in the ISO/IEC 30107-3 standard at this time.
Thresholds for Certification
Each level sets specific benchmarks for two critical metrics:
- APCER (Attack Presentation Classification Error Rate): Measures the system's failure rate in rejecting attacks.
- BPCER (Bona Fide Presentation Classification Error Rate): Evaluates the system's rejection rate for genuine users.
- Level 1: APCER < 1.5%, BPCER < 2.0%
- Level 2: APCER < 1.0%, BPCER < 1.5%
Biometric Modalities Certification
Beyond liveness detection, iBeta offers comprehensive testing for biometric systems, certifying their accuracy, robustness, and resistance to spoofing across multiple modalities.
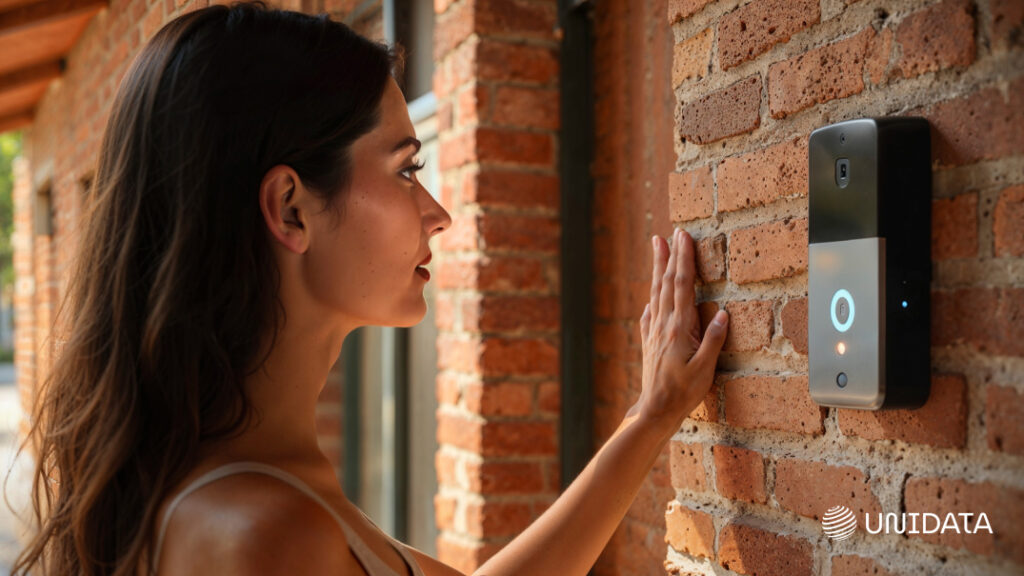
Facial Recognition systems are rigorously tested to ensure they can prevent spoofing attempts using static photos, videos, or masks. This testing ensures that the system can accurately differentiate between a live person and a fake representation. Common applications for facial recognition include device unlocking, such as smartphones and laptops, which offer secure biometric access; identity verification for online processes like eKYC (electronic Know Your Customer); and airport security where automated border control gates rely on this technology to maintain secure and efficient passenger management.
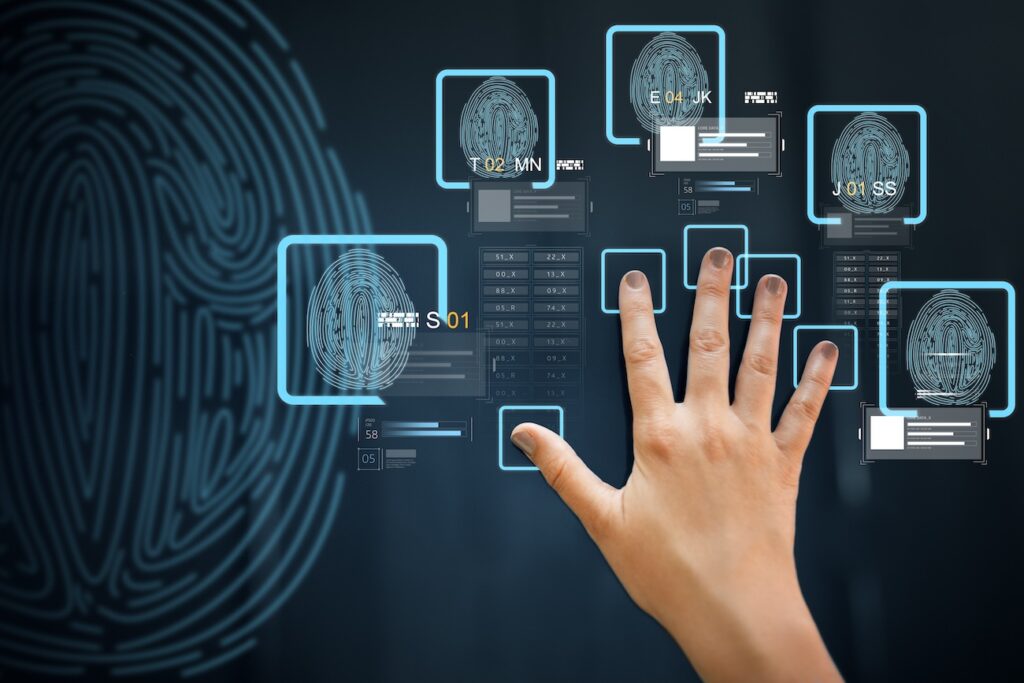
Fingerprint Recognition systems are tested to ensure they can resist spoofing attempts using high-quality artifacts like silicone or rubber molds. These tests are designed to confirm that systems can tell the difference between genuine fingerprints and replicas. Fingerprint recognition plays a critical role in banking systems, where it is used for secure transaction authorization in ATMs or mobile apps, as well as in attendance management systems for accurate time tracking in workplaces or schools. It's also used for device access, including laptops and other biometric-locked devices.
Voice Recognition systems are evaluated for their ability to detect live voices and reject playback or synthesized audio attacks. This is essential for applications like smart assistants, ensuring secure responses only to authorized users; call center authentication, where customer identity is verified during support interactions; and remote account access, where it helps prevent unauthorized access to sensitive online accounts.
Iris Scanning systems are tested to ensure they can differentiate between live irises and spoof attempts using high-resolution printed images or textured contact lenses. Iris scanning is used in government facilities for restricted access to highly secure locations, border control at international checkpoints to verify passenger identities, and top-tier security environments, including high-security installations such as research labs and defense facilities.
Common Testing Scenarios
Preparing for iBeta Certification involves simulating realistic testing scenarios to validate a biometric system’s resistance to Presentation Attacks (PAs) and ensure compliance with key performance benchmarks.
Breakdown of iBeta’s Testing Methodology
- Number of Attempts and Attack Types:
- iBeta evaluates systems using 360–1,200 spoofing attempts, depending on the testing level.
- Attacks include static artefacts (e.g., printed photos, screen displays) for Level 1 and dynamic artefacts (e.g., 3D masks, deepfake videos) for Level 2.
- Devices and Conditions:
- Systems are tested across multiple devices, including varying camera resolutions and sensor qualities.
- Conditions such as lighting variability (e.g., bright, dim, or uneven light) and angles are introduced to mimic real-world usage.
Key Metrics and Benchmarks
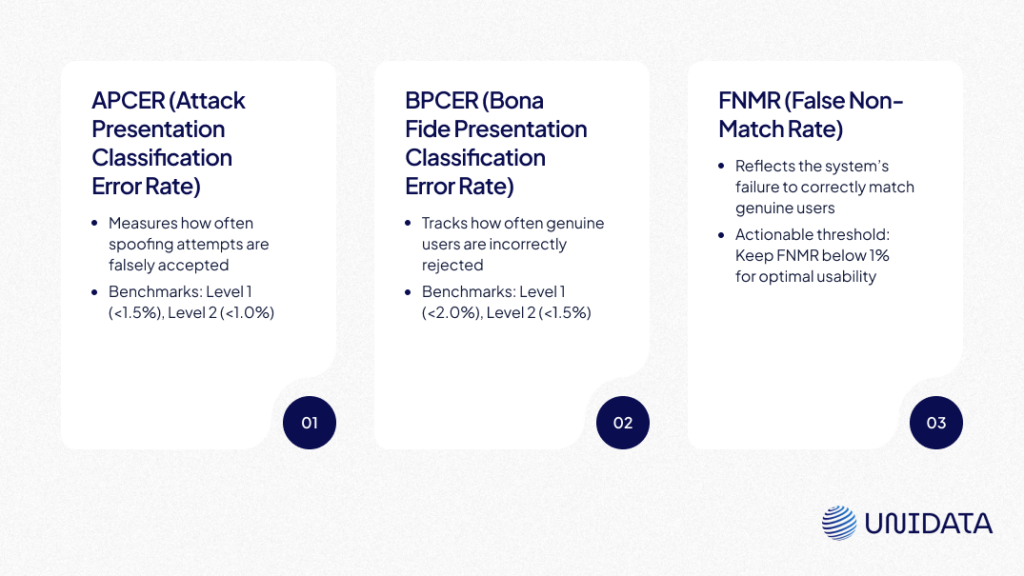
We will now take a deeper dive into the process of achieving iBeta Liveness Certification.
Pre-Certification Preparation
Below is a detailed, step-by-step preparation guide, divided into Level 1 and Level 2 testing requirements. These levels correspond to increasing complexities of Presentation Attack Detection (PAD), as outlined by ISO/IEC 30107-3.
Level 1: Preparing for Basic Liveness Detection
Step 1: Data Collection and Preprocessing
To ensure the system is robust, collect facial images and videos across a wide demographic. This should include a diverse range of age, gender, ethnicity, and lighting conditions (bright, dim, natural). Additionally, data should come from both indoor and outdoor settings to reflect real-world environments. You can use datasets like CASIA-WebFace for face recognition training, or collect custom data that matches your target user base.
Enhance your dataset’s variability using data augmentation techniques like geometric transformations (rotation, scaling, flipping), color adjustments (brightness, contrast, hue), and simulating random occlusions (e.g., glasses, scarves). Libraries like Albumentations or TensorFlow are excellent for applying these changes. It’s also essential to preprocess and clean the data by removing noisy or low-quality images, normalizing image sizes and brightness levels, and filtering out duplicates.
Step 2: Developing Basic Anti-Spoofing Capabilities
For Level 1 testing, implement basic Presentation Attack Detection (PAD) techniques. This includes motion analysis, such as detecting eye blinks or head tilts, and texture analysis to identify the micro-patterns on skin, which are absent in photos or screens. Train Convolutional Neural Networks (CNNs) to identify liveness cues like depth and natural texture variations. Use tools like OpenCV to analyze surface reflections and irregularities.
Simulate simple spoofing attempts, including printed photos and low-resolution video loops, under consistent lighting and stable camera angles to test the system’s resistance to these attacks.
Step 3: Performance Metrics and Threshold Adjustment
Achieving a balance between False Acceptance Rate (FAR) and False Rejection Rate (FRR) is key. Ensure that FAR is low to avoid spoofing attempts being falsely accepted, and FRR is within an acceptable range to prevent legitimate users from being rejected frequently. Fine-tune the system's decision thresholds during iterative testing to meet the required Level 1 metrics: APCER < 1.5% and BPCER < 2.0%.
Step 4: Conducting Internal Testing
Simulate real-world conditions by testing the system under various scenarios like poor lighting, glare, different camera angles, and users wearing hats, glasses, or masks. Conduct several test cycles to iterate and refine the system based on feedback. Analyze system logs for any missed detections or false positives and retrain models with augmented data where necessary to address any gaps. Finally, ensure that the system meets all iBeta Level 1 thresholds and standards before formal submission for testing.
Level 2: Preparing for Advanced Liveness Detection
Step 1: Data Collection and Preprocessing
For advanced liveness detection, you need to expand your data collection process. This should include high-quality datasets that reflect real-world complexities, such as users in motion (walking or turning their heads), and varied lighting conditions, like backlighting or uneven illumination. Suggested datasets include custom-collected, high-resolution data that’s tailored to your target environment.
Advanced data augmentation techniques are crucial for simulating challenging conditions. This can include applying motion blur to simulate user movement, introducing occlusions (like masks, glasses, or hand gestures partially covering the face), and generating synthetic artefacts, such as realistic, synthetic faces using StyleGAN. For enhanced preprocessing, normalize color channels to ensure consistent skin tone detection and apply Gaussian noise or sharpening filters to simulate device-level artefacts.
Step 2: Developing Advanced Anti-Spoofing Capabilities
At Level 2, you need to integrate advanced Presentation Attack Detection (PAD) techniques. Depth analysis is key, using stereoscopic or infrared imaging to detect the 3D features of a real face. Additionally, incorporate advanced behavioral biometrics, such as validating user gestures like nodding or smiling.
Utilizing AI-powered tools is essential at this level. Frameworks like PyTorch or TensorFlow can be used to train models on sophisticated liveness cues, and adversarial training tools like CleverHans can improve resilience against engineered attacks.
Your testing setup should simulate high-quality spoofing attempts using silicone and latex masks that have been fabricated by taking molds directly from human faces, along with deepfake videos created using platforms like DeepFake Lab.
Step 3: Performance Metrics and Threshold Adjustment
At Level 2, refining your performance metrics is critical. Set tighter thresholds to meet the required standards: APCER < 1.0% and BPCER < 1.5%. Dynamic testing is essential to continuously adjust these thresholds. Perform multi-condition tests, varying angles, distances, and camera types to simulate real-world conditions, and test against spoofing artefacts under real-world settings.
Step 4: Conducting Internal Testing
Simulate a variety of advanced attack scenarios to test the system’s resistance. This includes using high-quality silicone masks, resin replicas, deepfake videos, and adversarial video inputs. Conduct multiple test cycles, incorporating feedback from each round to retrain models on missed spoof cases and optimize algorithms for specific environmental challenges.Finally, ensure your system complies with iBeta’s Level 2 standards. Use the official standards checklist to confirm that the system meets the necessary thresholds before formal submission.
Testing with Presentation Attack Instruments (PAIs)
Effective testing with Presentation Attack Instruments (PAIs) is crucial to prepare a system for the challenges of iBeta Certification. This involves replicating potential attack scenarios to assess the system's resilience.
Creating and Testing with PAIs
Developing PAIs tailored to the certification level is essential. Silicone masks, crafted by molding directly from human faces, offer unmatched realism, capturing intricate details like pores, wrinkles, and natural contours. These lifelike qualities make them ideal for simulating advanced spoofing attempts and evaluating liveness detection accuracy.
In contrast, 3D printing, while accessible, often fails to replicate the fine textures and depth of real human skin. Features like translucency and irregularities are difficult to achieve, limiting their effectiveness compared to silicone-based masks.
Alongside these physical artefacts, synthetic faces generated using StyleGAN add another layer of complexity by simulating high-resolution facial features. DeepFake Lab can further contribute by creating spoofing videos to evaluate the robustness of liveness detection systems.
Example: A mobile banking app PAD system was enhanced by testing with spoofing videos generated through DeepFake Lab, leading to improvements in detecting unnatural blinking patterns and skin inconsistencies.
Key Evaluation Metrics
Metrics like APCER (Attack Presentation Classification Error Rate) and BPCER (Bona Fide Presentation Classification Error Rate) guide refinement efforts. If an initial test reveals an APCER of 2% for high-gloss photos, introducing additional training data and adjusting texture analysis algorithms can bring the metric below the required 1% threshold for Level 2 certification.
Training and Testing Processes for Biometric Certification
Biometric certification processes for different modalities involve rigorous training and testing to meet iBeta and ISO/IEC standards. Each modality requires specific methods to ensure systems are robust against spoofing attempts and meet accuracy, security, and usability benchmarks.
Face Recognition
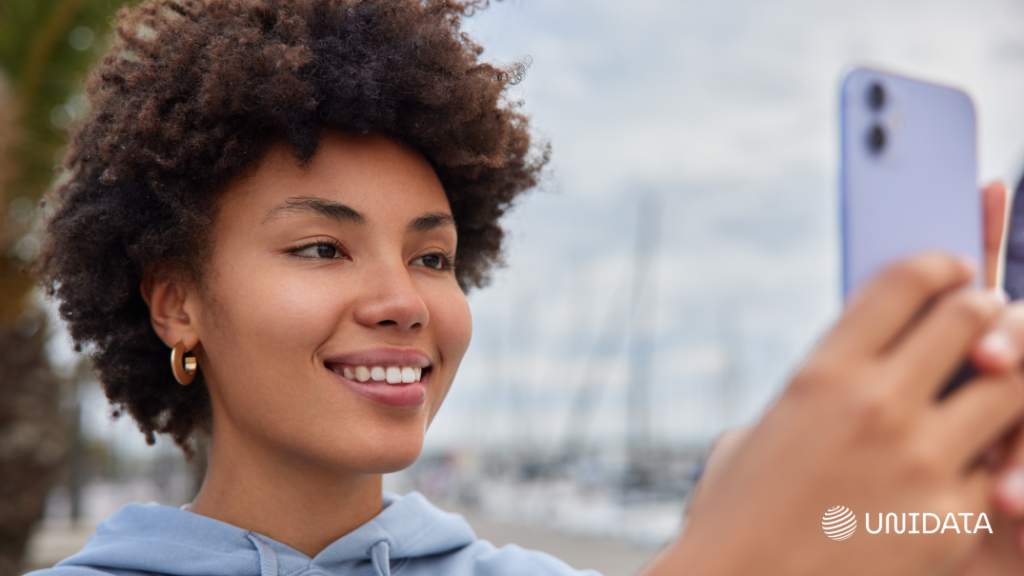
Face recognition systems are assessed for their ability to detect live users and resist spoofing attempts, such as printed photos, videos, or 3D masks.
Training Process
The first step involves compiling diverse datasets, such as CASIA-WebFace or VGGFace, ensuring inclusivity across demographics and lighting conditions. Data augmentation follows, including transformations like rotation, scaling, and occlusions (e.g., glasses or scarves) to simulate real-world variability.
For liveness detection, machine learning models are trained to detect texture, motion (such as blinking), and depth cues, often using CNNs or multi-camera setups.
Testing Process
The testing phase involves simulating various attack scenarios, such as printed photos, deepfake videos, and silicone masks. Key performance metrics are the False Acceptance Rate (FAR) and False Rejection Rate (FRR), ensuring they meet iBeta's thresholds, like APCER < 1.0% for Level 2 certification.
Voice Recognition
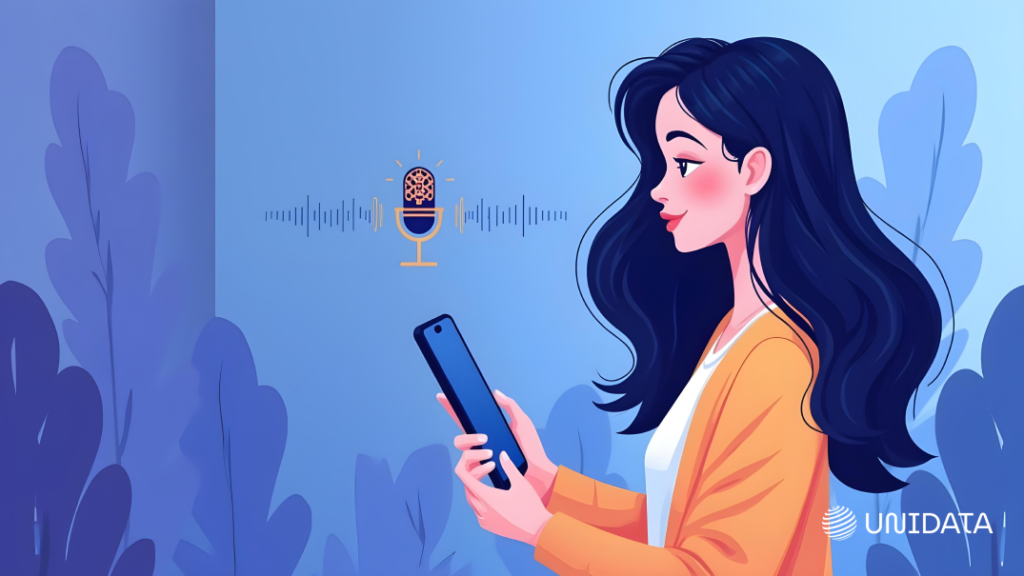
Voice recognition systems must distinguish between live voices and attempts to spoof the system with playback or synthetic audio.
Training Process
Data collection involves gathering audio samples from diverse environments, including noisy backgrounds and varying accents and ages. Data augmentation techniques such as pitch shifting, adding noise, and time-stretching help the system generalize better.
Anti-spoofing training focuses on spectrogram analysis to detect anomalies in frequency patterns typical of playback or synthesized voices.
Testing Process
The testing process includes simulating attacks with recorded voices, synthesized audio, and deepfake audio, such as those generated using WaveFake. Performance metrics to evaluate include Equal Error Rate (EER) and FAR/FRR across different environments.
Fingerprint Recognition
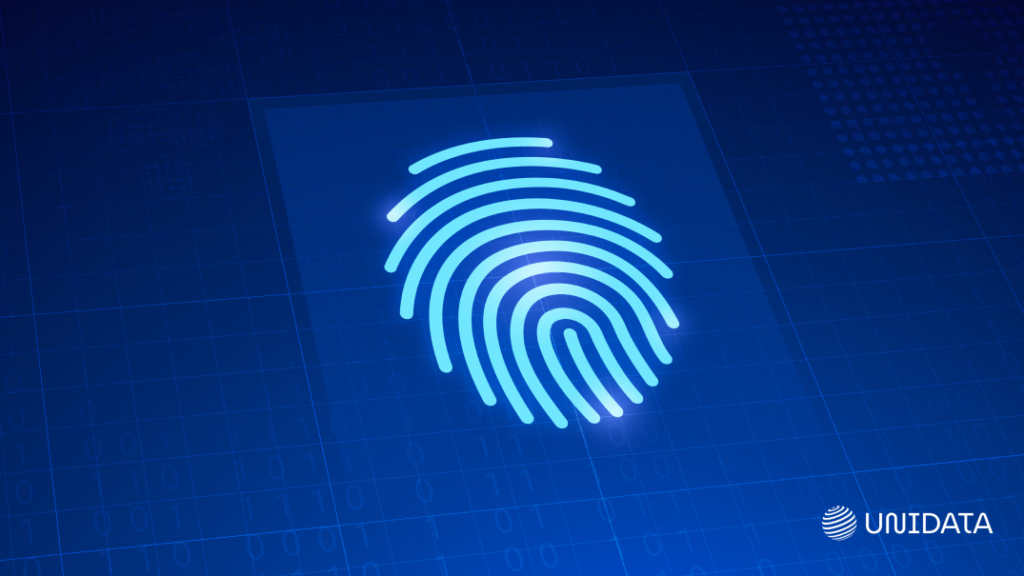
Fingerprint recognition systems need to show high accuracy and resistance to spoofing attempts, such as fake fingerprints or environmental variability.
Training Process
To ensure dataset diversity, publicly available datasets like FVC2002/FVC2004 and LivDet Fingerprint Database are used. The data should reflect different demographics, environments, and sensor types. Additionally, data augmentation includes distortion simulation, moisture variations, and synthetic fingerprint generation through GAN-based models.
For anti-spoofing, models are trained to analyze texture and ridge flow, minutiae points, and sweat pores. They also identify fake fingerprints made from materials like silicone or latex.
Testing Process
Testing against presentation attack instruments (PAIs) involves simulating attacks using 3D molds, fake materials (silicone, resin), and latent prints. Environmental testing simulates conditions like moisture variability, partial prints, and sensor quality.
Key performance metrics include False Acceptance Rate (FAR), False Rejection Rate (FRR), Equal Error Rate (EER), and Attack Presentation Classification Error Rate (APCER), ensuring the system's resistance to PAIs.
Iris Recognition
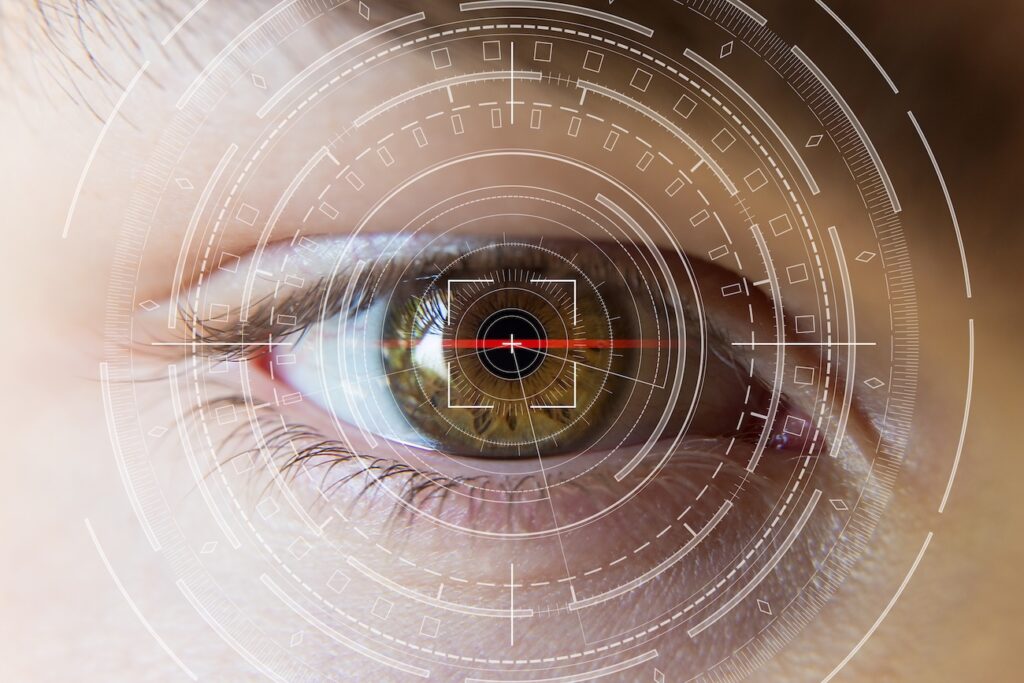
Iris recognition systems are evaluated on their ability to detect live irises and distinguish them from spoofed attempts using printed eyes, textured contact lenses, or 3D-printed models.
Training Process
For dataset collection, resources like CASIA-Iris and ND-Iris-0405 are used, including images captured under varied lighting and environmental conditions. Models are trained to detect micro-patterns and reflections unique to live irises, and multi-spectral imaging is employed for depth analysis.
Testing Process
During testing, the system's resistance to high-quality printed replicas, 3D models, and textured contact lenses is evaluated. Performance metrics focus on validating APCER/BPCER thresholds, ensuring accuracy across different sensors and environmental conditions.
Submitting for iBeta Testing
Documenting Test Results
The submission of test results is a crucial step in the iBeta certification process. Reports must include:
- System Performance Metrics: Include FAR, FRR, APCER, and BPCER values.
- Attack Resistance: A summary of the simulated attacks and how the system responded.
- Environmental Details: Information on lighting conditions, device types, and testing environment.
For clarity, use tables and graphs to present the metrics, providing annotations on any anomalies and explaining the corrective measures taken.
What to Expect During iBeta Testing
Testing Phases
iBeta conducts testing in controlled lab environments, where standardized presentation attack instruments (PAIs) are applied to evaluate the system’s robustness. These simulated attacks test the system’s resistance to various spoofing methods.
Timeline
The certification process generally takes 2 to 6 weeks, depending on the system’s complexity. If the system does not pass initial tests, feedback loops will be incorporated, allowing for necessary adjustments and retesting.
Cost
Costs vary based on the project's specific requirements, but they are generally accessible for basic tests. For detailed pricing, specific quotes can be obtained directly from iBeta.
Common Pitfalls to Avoid
- Insufficient Testing: Failing to simulate enough attack types, especially advanced PAIs for Level 2.
- Inadequate Data Preprocessing: Overlooking normalization or noise reduction in datasets can reduce accuracy.
- Ignoring Environmental Factors: Neglecting variability in lighting, user angles, or device quality may lead to false rejections or acceptances.
How We Collect Data for Our Clients' Certification
Achieving iBeta Certification requires a detailed and collaborative process to ensure the collected data meets the highest standards. Below is an overview of our workflow, designed to guide clients through the critical steps of data preparation and validation for biometric system compliance.
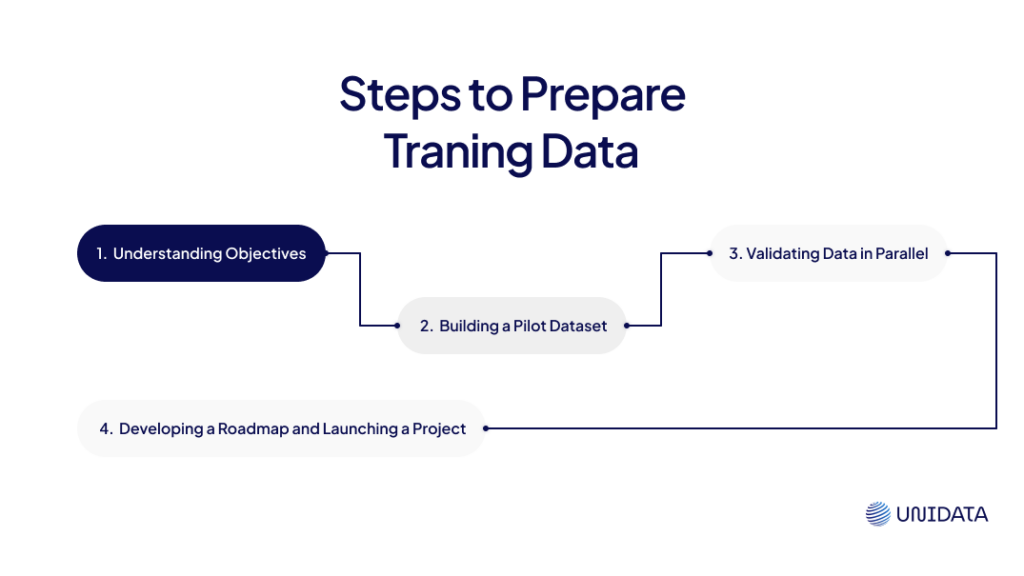
1. Understanding Objectives
At Unidata, we begin by developing a Technical Specification (TS) in close collaboration with our clients. This document outlines all the requirements, ensuring we’re aligned on clear deliverables and expectations. We share the TS with the client for review and refinement at every stage. Once approved, the TS serves as the foundation for the entire project.
2. Building a Pilot Dataset
Next, we create an initial test set to verify compatibility with system requirements and to identify any immediate gaps. Based on the feedback, we refine the dataset. This may include improving diversity in data or adjusting parameters. After validating the test set, we finalize the format for broader data collection.
3. Validating Data in Parallel
We take a real-time validation approach: As we collect additional data, we run continuous checks to ensure:
- Data consistency across demographics and environmental conditions.
- No anomalies or inconsistencies in the dataset.
This proactive validation helps us detect potential issues early on, allowing us to fix any processing errors or gaps before the larger data collection phase.
4. Developing a Roadmap and Launching a Project
To keep things on track, we develop a structured timeline that outlines key milestones such as dataset completion deadlines. Regular progress checks allow us to adjust the plan as needed, ensuring the project remains aligned with the client’s evolving goals and scope.
Specifics of Incorporating Specialized Mask
To meet iBeta’s standards, we introduce diverse 3D mask features into the dataset. This includes variations in hair types (like wigs and facial hair) and mask sizes extending to the collarbone. We also simulate real-world scenarios to better reflect actual use cases.
For advanced 3D mask fabrication, we partner with trusted vendors who provide high-quality masks. Our team coordinates closely with external specialists to maintain consistency and meet all compliance requirements.
Why Our Workflow Works
By following this structured, collaborative approach, we ensure that every client project is tailored to meet both iBeta standards and the unique needs of their biometric system. From early-stage validation to specialized data preparation, we focus on precision, flexibility, and client satisfaction at every step.
Post-Testing Steps
Receiving Feedback from iBeta
- Post-Test Reports:
- iBeta provides a detailed analysis, highlighting strengths and areas for improvement.
- Feedback includes metrics like APCER spikes under specific conditions or FAR inconsistencies.
- Interpreting Feedback:
- Pinpoint the exact conditions or attack types where the system underperformed.
- Use feedback to refine models and retrain with augmented datasets.
Ensuring Long-Term Success
- Regular Updates:
- Continuously update models with real-world data and emerging spoofing threats.
- Integrate adaptive learning algorithms to improve performance over time.
- Ongoing Testing:
- Periodically validate the system using internal tests that replicate iBeta’s methodology.
- Keep pace with advancements in PAD and adversarial attack techniques.
- Continuous Learning:
- Monitor developments in biometric technology and standards, including future updates to ISO/IEC 30107-3.
- Incorporate the latest research in AI-driven PAD techniques.
Tips:
- Monitoring Systems with Prometheus and Grafana
A robust monitoring setup ensures operational readiness. Prometheus captures real-time metrics, including FAR, FRR, and APCER, while Grafana visualizes trends and anomalies. For example, a system might detect an unexpected rise in APCER during a new app feature rollout. By identifying such patterns, engineers can isolate the issue and deploy quick fixes.
Practical Guide: Setting up a Prometheus + Grafana pipeline not only tracks system health but also integrates alert mechanisms for early intervention. See Prometheus Documentation for guidance.
- Implementing Feedback for Continuous Improvement
Feedback from certification testing often highlights areas for improvement. For example, if printed photo artefacts caused high APCER in low-light conditions, engineers might add infrared-based detection and retrain models with augmented datasets. These improvements could then be validated in simulated environments, ensuring the system remains robust under similar conditions.
Iterative Improvements from Real-World Certifications
Suprema’s Iterative Process
Suprema faced a challenge during their initial Level 1 tests, as the system struggled to detect glossy printed photos under specific lighting conditions. To address this, they introduced infrared-based liveness detection, allowing the system to better assess depth and material properties. Additionally, they retrained the convolutional neural networks (CNNs) with an enhanced dataset that included synthetic artefacts, which improved the system’s ability to generalize across different conditions. As a result, Suprema achieved certification with an APCER of <0.5%, showing strong performance across a range of environmental conditions. iBeta Testing
Advance Intelligence’s Journey
Advance Intelligence initially encountered difficulties detecting silicone masks, as their liveness detection relied too heavily on static features. To resolve this, they integrated passive liveness detection, which analyzed light reflectance to more accurately differentiate between genuine and fake inputs. They also conducted stress tests under variable lighting to ensure consistent performance. Thanks to these refinements, the system earned Level 2 certification with an APCER of 0.8%, setting a new benchmark for robustness, particularly in mobile applications. iBeta Testing
Adaptation to Advanced Threat
As attacks become increasingly sophisticated, systems must proactively adapt to new threat vectors, including adversarial and multimodal challenges.
Preparing for Adversarial Attacks
Adversarial testing is a vital component of threat mitigation. Using tools like Foolbox or CleverHans, engineers can create artefacts that exploit model weaknesses, such as subtle pixel alterations designed to bypass detection. Incorporating these adversarial examples into training improves system resilience.
Integrating Multimodal Biometrics
Combining modalities—such as face, fingerprint, and iris recognition—provides layered defenses. For instance, discrepancies between facial features and voice inputs can help identify deepfake attacks, adding a safeguard against emerging threats.
Ethical Considerations and Bias Mitigation
Addressing bias ensures biometric systems perform equitably across diverse populations. This is not just a technical necessity but a vital ethical responsibility.
Fairness Testing
Fairness evaluations involve analyzing system performance across various demographic groups. Tools like Aequitas enable engineers to identify discrepancies in metrics like FAR and FRR. For instance, a system might initially underperform for older users or darker-skinned individuals. Retraining on a dataset balanced with these demographics can significantly reduce such disparities.
Historical Case: In 2020, a widely used system faced scrutiny for exhibiting a 10% higher FAR for darker-skinned users. Retraining with demographically inclusive datasets reduced this disparity to 2%, ensuring fairness.
Anticipated Updates to ISO Standards
Anticipating updates to standards helps organizations future-proof their systems. The upcoming ISO/IEC 30107-4, expected by 2025, introduces advancements to address evolving biometric challenges.
Key Expected Updates
- Adaptive Learning: Models will need to incorporate live data for continuous improvement, allowing them to adapt dynamically to emerging attack types.
- Expanded Multimodal Standards: Guidelines for integrating multiple biometric modalities, such as face, voice, and fingerprint, will become central to certification.
Preparing for the Future
Organizations can start adopting adaptive learning frameworks to refine their systems in real-time. For example, a PAD system could be trained to detect novel deepfake attacks as they emerge, reducing response time and costs associated with retrofitting older models.
Forward-Looking Strategy: Teams that proactively integrate these capabilities into their development pipelines will position themselves ahead of the competition as the new standards roll out.
Conclusion
The journey to iBeta Certification requires careful planning, iterative testing, and leveraging advanced tools and research. By following this expanded guide, integrating best practices, and staying updated on emerging standards, biometric systems can achieve certification and lead in a rapidly evolving security landscape.
Appendix: Resources for Further Reading
iBeta Testing Documentation
- iBeta PAD Testing Overview
Provides detailed insights into how iBeta conducts PAD testing for biometric systems, including liveness detection for ISO/IEC 30107-3 compliance.
iBeta Biometric Testing Services - iBeta Liveness Detection and PAD Testing Standards
Comprehensive guidelines for both Level 1 and Level 2 testing, including metrics like APCER and BPCER.
iBeta Standards for Liveness Detection
ISO/IEC 30107-3 Documentation and Related Standards
- ISO/IEC 30107-3 Presentation Attack Detection Standard
Official description of the ISO framework and principles for PAD testing. Access through ISO's main site or licensed distributors for full documentation.
Learn more: ISO Official Site - Detailed Comparisons of PAD Testing Levels
Explains Level 1 and Level 2 attack simulation and metrics required for compliance. It includes species of attacks and penetration limits.
iBeta ISO PAD Standards Comparison
Anti-Spoofing Technology and Tools
- CleverHans and Foolbox for Adversarial Testing
These libraries provide tools to simulate and test adversarial attacks against biometric systems.- CleverHans: GitHub Repository
- Foolbox: GitHub Repository
- StyleGAN for Synthetic Dataset Generation
Generate synthetic face images to augment datasets for face recognition systems.
StyleGAN GitHub Repository
Dataset Resources
- LivDet and FVC Databases for Fingerprint Testing
Datasets designed for PAD testing, including genuine and spoofed fingerprints.- LivDet: LivDet Official Site
Biometric Testing Tutorials
- WaveFake for Voice Recognition PAD
Tools for synthesizing adversarial audio attacks and improving voice PAD systems.
WaveFake GitHub Repository
Companies that have achieved iBeta Certification
When discussing the confirmation of respect for iBeta, it’s important to recognize the companies that have achieved iBeta Certification.
This certification signifies a commitment to quality and excellence in various fields. Here’s a list of some of those certified companies:
Company | Description | Industry |
---|---|---|
NEC | A global leader in IT and network technologies | Technology, IT Services |
Tencent | A Chinese technology giant known for WeChat, gaming, and cloud services. | Technology, IT Services |
Microsoft Azure Cognitive Services | Microsoft’s cloud AI services for building intelligent applications | Technology, IT Services |
Amazon Development Center U.S., Inc. | Amazon’s R&D division focusing on cloud computing and AI innovations. | Technology, IT Services |
Dicio | Focuses on AI-powered decision-making tools for businesses. | Technology, IT Services |
Shopee | A leading e-commerce platform in Southeast Asia and Taiwan. | E-commerce |
GoTo Financial | Provides digital payment and financial services across Southeast Asia. | Financial Services, FinTech |
E.SUN COMMERCIAL BANK | A leading Taiwanese bank focusing on innovative financial services | Financial Services, FinTech |
Sumsub | Provides KYC and anti-fraud services with identity verification. | Financial Services, FinTech |
SenseTime | A major AI company, specializing in facial recognition and smart cities. | Cybersecurity, Fraud, Identity Verification |
Innovatrics | Provides biometric identification and AI-based identity management tools. | Cybersecurity, Fraud, Identity Verification |
FacePhi | Provides facial recognition and biometric identity solutions. | Cybersecurity, Fraud, Identity Verification |
IDfy | Specializes in identity verification and KYC solutions. | Cybersecurity, Fraud, Identity Verification |
authID.ai (Ipsidy, Inc.) | Provides biometric identity verification solutions for secure transactions. | Cybersecurity, Fraud, Identity Verification |
FaceTec | Specializes in 3D facial authentication technology for secure onboarding. | Cybersecurity, Fraud, Identity Verification |
Fraud.com | Offers fraud management and prevention solutions for online businesses. | Cybersecurity, Fraud, Identity Verification |