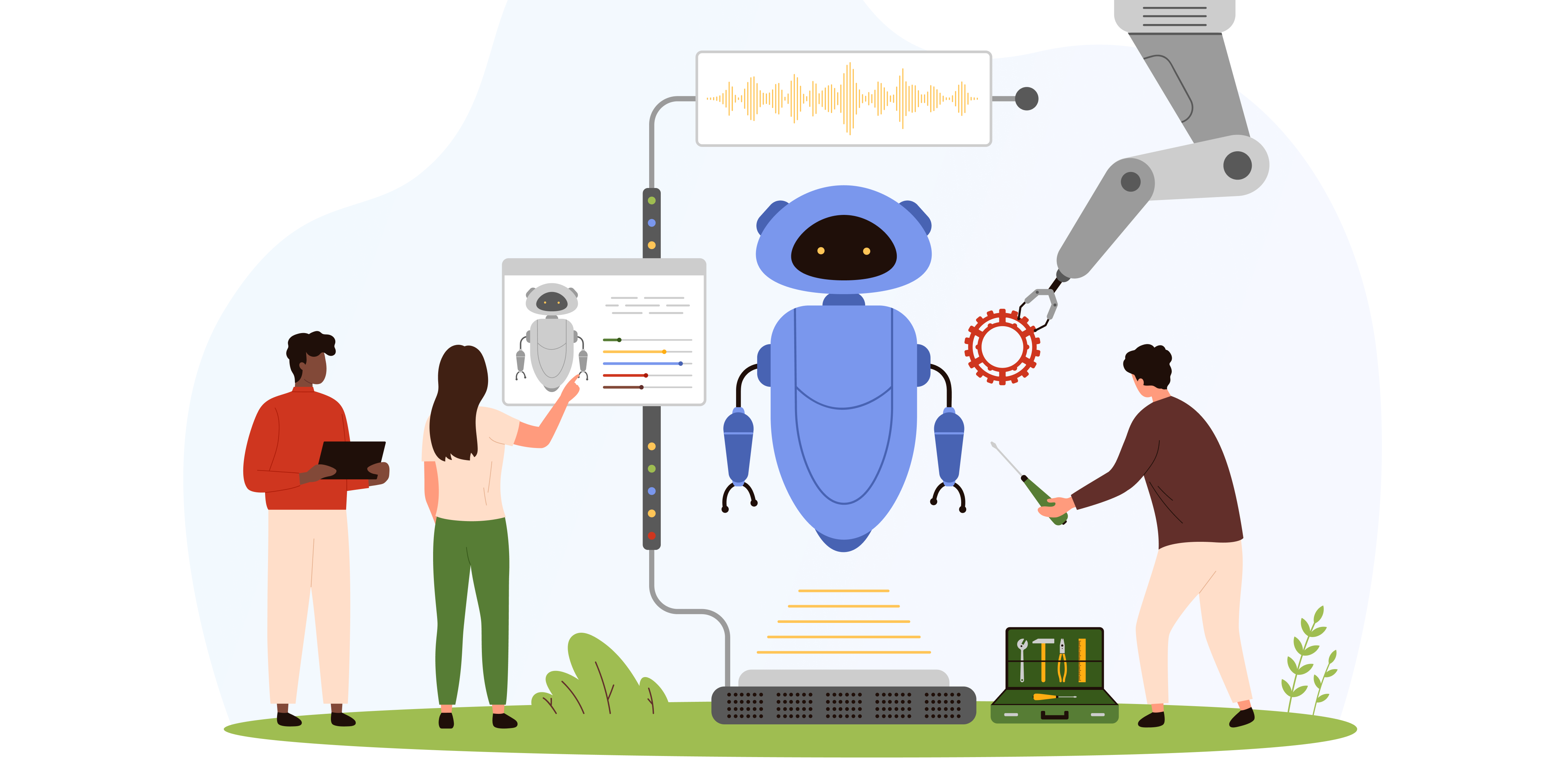
Getting deeper into machine learning, we come across the concept of Human-on-the-Loop (HOTL). This is an approach where human intelligence takes control of AI improvement and creates a balance between automation and human insight.
In this article, we aim to cover the basic concepts, approaches, and real-life applications of human-on-the-loop. Let’s dive deeper into uncovering the role of humans in machine learning.
What Is Human-on-the-Loop in Machine Learning?
Human-on-the-loop (HOTL) in machine learning is a unified approach to integrating human feedback into AI system operations. This helps to have continuous oversight and improvement of machine learning models.
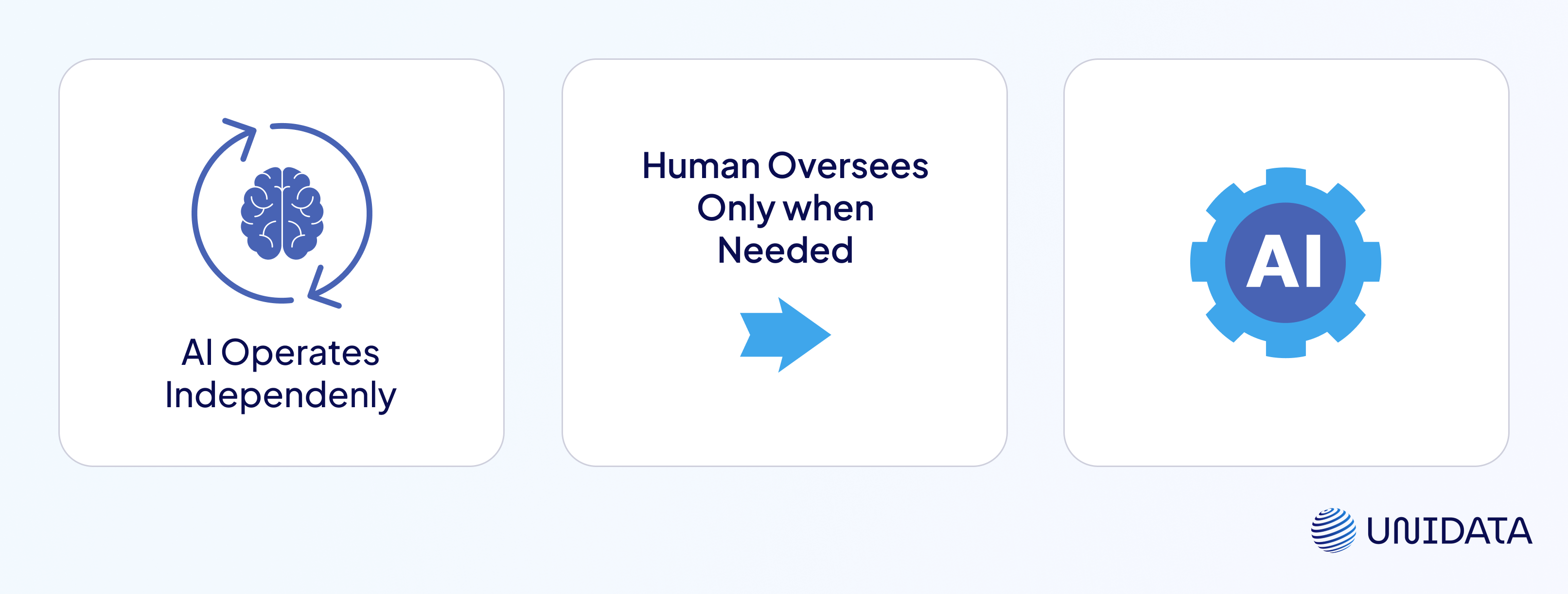
Unlike Human-in-the-Loop (HITL), where human intervention is required for each decision or action the machine makes, HOTL integrates humans as supervisors or auditors who step in as needed to guide, correct, or improve the system based on its performance and outcomes.
In HOTL setups, the ML system operates autonomously for the most part, processing data, making decisions, or providing recommendations. However, it's designed to involve human feedback at critical points, such as when the system encounters scenarios it's unsure about, when it needs to learn from new or evolving data patterns, or when its decisions have significant implications that require human judgment.
The human feedback loop guarantees that the AI system is aligned with human values, ethics, and expectations. It improves the system's accuracy and reliability over time by learning from the insights provided by human overseers.
By keeping humans "on the loop," we can maintain control over AI operations and be able to intervene when necessary, thus fostering more trustworthy and effective AI solutions.
Key Components of Human-on-the-Loop
A survey of human-in-the-loop for machine learning emphasized that integrating human knowledge improves model training and decision-making accuracy. Applying similar principles, HOTL structures systems to maintain continual human involvement for learning and risk mitigation.
The Human-on-the-Loop (HOTL) approach in machine learning and AI systems involves several key components to ensure the effective integration of human oversight with autonomous machine operations.
These components work together to create a dynamic system where human expertise and machine efficiency complement each other. Here are the essential elements of HOTL:
Autonomous AI System
The foundation of a HOTL setup is the autonomous AI system designed to perform tasks, make decisions, or analyze data with minimal human intervention. AI systems are the primary action takers, processing inputs, and executing tasks based on learned algorithms and data models.
Intervention Mechanisms
These are predefined rules or triggers that determine when human intervention is required. They can be based on confidence scores (where the AI's certainty in its decision falls below a certain threshold), ethical considerations, or cases that are unexplored or rare.
These mechanisms ensure that humans are brought into the loop at critical moments to guide the AI system, preventing errors or addressing situations the AI is not equipped to handle alone.
Human Expertise
The involvement of human experts or operators who monitor the AI system's performance and intervene when necessary. These human experts provide the necessary feedback, corrections, or decision-making that the AI system cannot autonomously handle. This component is crucial for the continuous improvement of the AI system, guaranteeing accuracy, reliability, and ethical alignment with human values and expectations.
Feedback Loop for Continuous Learning
A feedback loop is a mechanism that allows the system to learn from human interventions and feedback, incorporating this new information into its operations and decision-making processes.
AI systems use this to adapt and improve over time, reducing the frequency of errors or the need for human intervention as the system becomes more capable of handling complex or unknown situations independently.
Human-AI Interaction Interface
The user interface or platform is used to realize effective communication between humans and the AI system. This includes tools for monitoring system performance, providing feedback, and adjusting system parameters. This helps create an environment for meaningful human interventions, allowing humans to easily understand the system's outputs and input their feedback or corrections.
Adaptation and Evolution Strategies
Strategies and methodologies for updating the AI system based on feedback and learning from human interventions, including retraining models with new data or adjusting algorithms. This can help the system to evolve in response to new information, challenges, and environmental changes, enhancing its long-term effectiveness and autonomy.
By incorporating these components, the Human-on-the-Loop approach creates a powerful synergy between human expertise and machine efficiency, ensuring that AI systems remain adaptable, reliable, and aligned with human ethical standards.
Applications and Examples
Human-on-the-Loop (HOTL) has a wide range of applications across various industries, leveraging human oversight to enhance the performance, reliability, and ethical considerations of AI systems. Here are several applications and examples illustrating how HOTL is used in real-world scenarios:
Healthcare: Clinical Decision Support Systems
Human-on-the-loop can be integrated into clinical decision support systems where AI analyzes medical data to suggest diagnoses or treatment plans. Doctors review these suggestions, providing feedback that helps refine the AI's accuracy and ensuring that patient care remains at the forefront of healthcare decisions.
Example: IBM Watson for Oncology (used in hospitals like Manipal Hospitals in India)
IBM Watson analyzes vast volumes of medical literature and patient data to recommend cancer treatment options. Oncologists review these suggestions before making final decisions. Their feedback is used to retrain the AI and refine its future recommendations, keeping patient safety and care central to the process.
Autonomous Vehicles: Safety and Ethical Decision-making
In the development of autonomous driving technology, HOTL approaches can be used to evaluate and improve decision-making algorithms. Human operators monitor the system's performance and intervene in scenarios where the AI faces ethical dilemmas or safety-critical decisions, using these instances to train and improve the system.
Example: Waymo (Alphabet/Google's self-driving car project)
Waymo vehicles operate autonomously but are continuously monitored by human safety operators—especially during testing phases. When the vehicle encounters edge cases like unusual traffic patterns or construction zones, human drivers can take over, and the system logs these events to improve its decision-making models.
Social Media Platforms: Content Moderation
AI systems are employed to filter and moderate content on social media platforms, but they often require human moderators to review decisions on ambiguous content, hate speech, or misinformation. Feedback from these human reviews is used to continuously improve the AI's understanding and handling of complex content moderation issues.
Example: Meta (Facebook/Instagram)
Meta’s AI flags potentially harmful content like hate speech or misinformation, but final decisions are reviewed by human moderators, especially in nuanced or culturally sensitive cases. These human interventions are used to train the AI to better detect similar violations in the future.
Customer Service: Chatbots and Virtual Assistants
Human-on-the-loop is applied in customer service, where AI-driven chatbots handle initial inquiries. Human agents step in when the chatbot encounters queries it cannot handle or when the customer requests human interaction. The chatbots learn from these interactions, gradually improving their ability to autonomously resolve a wider range of customer issues.
Example: Intercom & Zendesk chatbots
These AI-driven support systems handle frequently asked questions, product inquiries, and troubleshooting. When they encounter complex issues or customer frustration signals (like repeated messages or “talk to a human”), the conversation is escalated to a human agent. Agents can then annotate or label the query to improve the chatbot's future responses.
Finance: Fraud Detection Systems
AI models are used to detect potentially fraudulent transactions. HOTL allows human analysts to review cases flagged by the AI as suspicious, providing feedback that refines the model's ability to distinguish between legitimate and fraudulent activities accurately.
Example: American Express
AI systems at AmEx scan transactions in real time for anomalies. When something suspicious is flagged (like a sudden foreign purchase), a human fraud analyst reviews the alert before action is taken. The system learns from both confirmed fraud and false positives, making future fraud detection more accurate.
Stock Market Datasets for Machine Learning
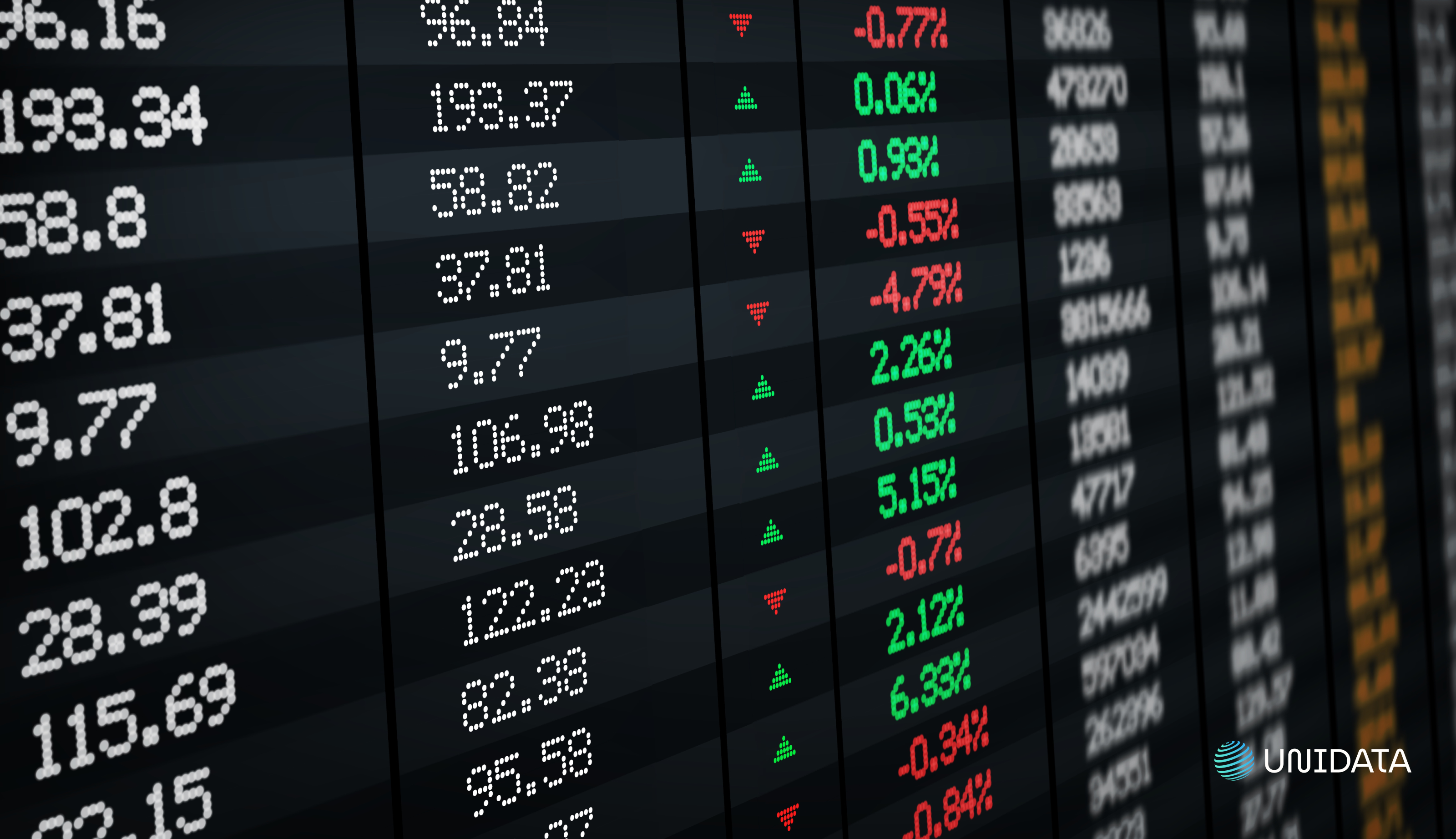
Education: Personalized Learning Platforms
AI-driven learning platforms adapt educational content based on student performance and preferences. Teachers, acting in a Human-on-the-loop capacity, can intervene to provide additional resources or change the learning trajectory based on their assessment of a student's needs, helping the AI to better personalize learning experiences.
Example: Carnegie Learning’s MATHia or Knewton (used in U.S. schools)
These platforms adjust learning content based on student interaction. Teachers can step in if a student is struggling despite AI recommendations, providing additional instruction, or modifying content. These human inputs help refine the algorithm’s adaptation models for future learners.
Manufacturing: Quality Control
In manufacturing, AI systems can identify defects or issues in products. Human supervisors review and validate these findings, using their expertise to inform the AI system, which in turn improves its detection capabilities and reduces false positives or negatives.
Example: Siemens Smart Manufacturing Solutions
AI-driven cameras inspect products for visual defects. When anomalies are detected, human quality assurance staff review them to confirm whether they’re true defects or false positives. Their decisions help train the computer vision system to improve over time, reducing unnecessary product rejections.
These examples demonstrate the versatility and value of HOTL in enhancing AI systems across different fields. By combining human insight with machine efficiency, Human-on-the-loop approaches help create more accurate, ethical, and user-friendly AI solutions that are better aligned with human needs and societal values.
Advantages of Human-on-the-loop
The Human-on-the-Loop (HOTL) approach in machine learning and AI systems presents several advantages, making it a valuable strategy for developing and deploying intelligent systems. Here are some key benefits:
- Improved accuracy and reliability: The iterative human feedback and improvement process reduces errors and enhances the model’s performance in ambiguous situations.
- Enhanced learning from real-world interactions: AI systems become more adaptable and capable of handling new or evolving scenarios, improving their applicability and effectiveness across different domains.
- Better ethical and legal compliance: The human-on-the-loop approach in machine learning reduces the risk of unethical outcomes or legal issues, fostering public trust in AI technologies.
- Customization to user needs and preferences: Leads to more personalized and user-friendly AI services and products, enhancing user satisfaction and engagement.
- Bridging the gap between AI and human expertise: Enables the development of solutions that are both technically advanced and deeply attuned to human contexts and values, fostering more effective collaboration between humans and machines.
- Risk reduction: Human-on-the-loop protects against the escalation of errors or biases, ensuring the AI system remains a safe and reliable tool. A survey by Abraham et al. (2021, IEEE WISH Workshop) in safety-critical systems (e.g., robotics), demonstrated that HOTL saves lives by enabling immediate human intervention when AI confidence drops.
Challenges and Considerations
Implementing Human-on-the-Loop (HOTL) involves navigating several challenges and considerations:
- Scalability: Balancing human intervention without compromising the system's ability to handle large volumes of data.
- Human expertise availability: Ensuring access to sufficiently trained personnel for effective oversight.
- Quality and consistency of feedback: Maintaining accurate and unbiased human input to guide AI learning.
- Integration with existing systems: Seamlessly incorporating HOTL mechanisms into current workflows.
- Ethical and privacy concerns: Safeguarding user privacy and upholding ethical standards during human intervention.
- Cost: Managing additional expenses related to training and maintaining a human oversight workforce.
- Dependence on human intervention: Striking a balance between human input and AI autonomy to encourage system improvement and independence.
What Human-on-the-loop Isn’t
While the Human-on-the-Loop (HOTL) approach is essential in creating efficient, adaptable, and ethically aligned AI systems, there are common misconceptions about what it entails.
Understanding what Human-on-the-loop is not is crucial to fully grasp its benefits and how it differs from other human-AI collaboration models.
So here we have a list of things that human-on-the-loop is not:
- Not a replacement for human expertise
Human-on-the-loop does not aim to replace human expertise or decision-making capabilities with AI. Instead, it leverages human intelligence to guide and improve AI systems, ensuring that these technologies enhance rather than supplant human skills and judgment.
- Not fully autonomous AI
Unlike fully autonomous systems that operate without any human intervention, Human-on-the-loop involves periodic human oversight and intervention. The goal is to maintain a balance between using AI's capabilities and ensuring human values and ethics guide its operations.
- Not Human-in-the-Loop (HITL)
Human-on-the-loop is often confused with Human-in-the-loop (HITL), but there’s a distinction. While HITL requires human input or approval for every decision or action taken by the AI, HOTL involves humans primarily for oversight, periodic checks, and interventions when necessary. In the section below, we have clarified the key differences of human on the loop vs human in the loop.
- Not a static system
Human-on-the-loop (HOTL) is not a one-time setup or a static system. It is a dynamic, evolving framework where human feedback and AI learning continuously interact. This process allows AI systems to adapt over time, improving their performance and reducing the frequency of necessary human interventions.
- Not a solution to all AI challenges
While HOTL can significantly improve AI systems' performance and ethical alignment, it is not a cure-all for all challenges in AI development. Issues such as algorithmic bias, data privacy, and the digital divide require broader solutions that encompass technical, regulatory, and societal efforts.
Understanding what Human-on-the-Loop doesn’t help clarify its role and potential in the development and deployment of AI systems. By recognizing these distinctions, we can better design, implement, and manage AI technologies in a way that maximizes benefits while minimizing risks and ethical concerns.
Key Differences between Human-on-the-loop and Human-in-the-loop
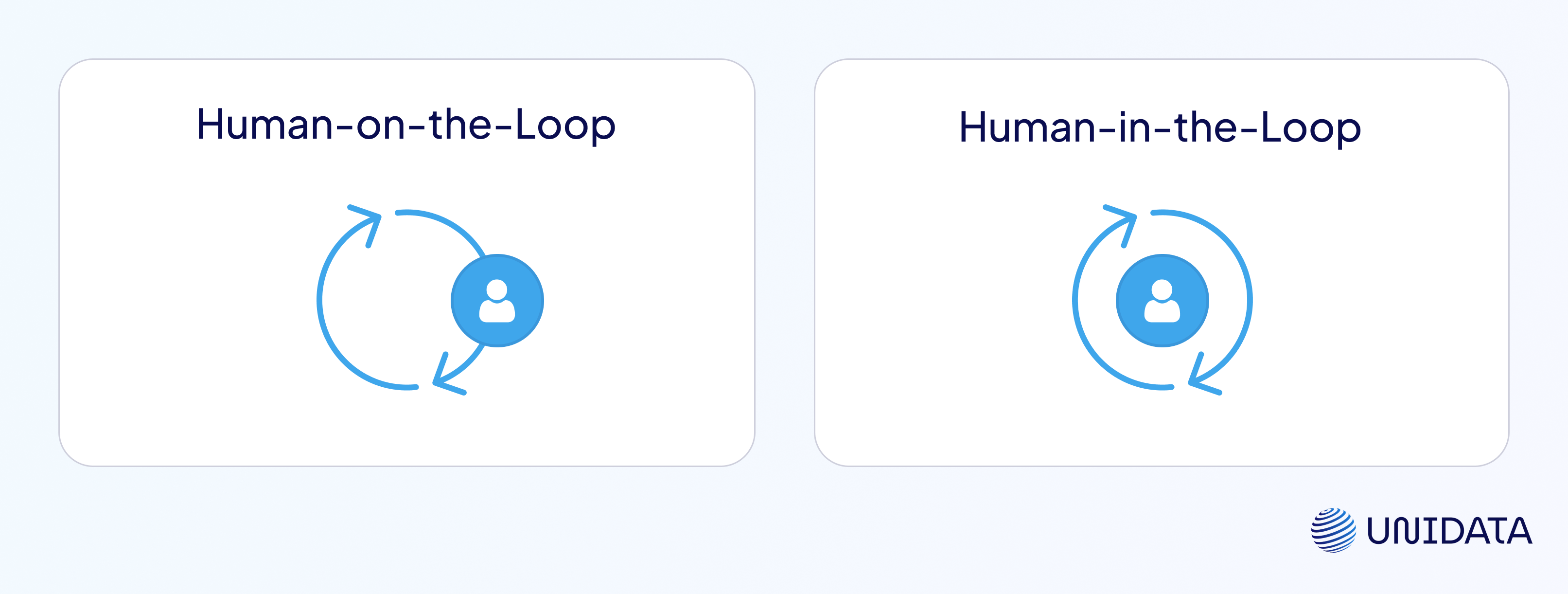
While both HITL and HOTL aim to balance automation with human insight, their roles within the machine learning process differ significantly. Below is a breakdown of how each model works in practice, highlighting where and when human interaction takes place.
Human-on-the-Loop (HOTL): Step-by-Step Process
HOTL focuses on autonomous operation with human oversight and feedback at key checkpoints. It’s particularly suitable for large-scale or real-time systems that require minimal interruption but benefit from periodic human intervention.
1. Autonomous Data Processing
The AI system independently ingests and analyzes data, applying pre-trained models to make predictions or decisions.
2. Monitoring & Detection of Uncertainty
The system flags output where confidence is low, anomalies are detected, or outcomes may have ethical implications.
3. Human Oversight & Review
A human operator reviews flagged cases intervenes if needed, and can override or adjust decisions.
4. Feedback Integration
The system incorporates human corrections into future predictions, either via retraining or continuous learning pipelines.
Human-in-the-Loop (HITL): Step-by-Step Process
HITL is designed for scenarios where human input is necessary throughout the AI workflow, particularly during model training and prediction stages. This approach is common in tasks requiring fine-tuned judgment, high-stakes decision-making, or active data labeling.
1. Data Collection & Labeling
Humans manually label or verify training data to ensure high-quality inputs for the model.
2. Model Training with Supervised Feedback
The model is trained using labeled data, and humans may guide the training process through iterative tuning.
3. Real-Time Decision Involvement
During inference, the system pauses at key decision points and sends output or recommendations to a human for approval or correction.
4. Feedback Loop
Human responses are logged and fed back into the system to retrain and refine the model over time.
Here's a comparison table highlighting the key differences between Human-on-the-Loop (HOTL) and Human-in-the-Loop (HITL) to clarify their distinct roles and implementations in AI systems:
Aspect | Human-on-the-Loop (HOTL) | Human-in-the-Loop (HITL) |
AI autonomy | AI operates autonomously for the most part, with humans providing oversight and intervention as needed. | AI requires human input or decision-making at key points in its operation, significantly relying on human interaction. |
Human intervention | Intervention is periodic, triggered by specific scenarios or when the AI requests assistance. | Human intervention is a continuous part of the process, necessary for the AI to complete tasks or make decisions. |
Role of humans | Humans act more as supervisors or auditors, stepping in to guide, correct, or approve AI decisions intermittently. | Humans are integral to the operational loop, actively participating in the decision-making process alongside the AI. |
System design | Designed to maximize AI independence while ensuring human oversight can be applied effectively when necessary. | Designed with the intention of integrating human judgments and inputs directly into the AI’s decision-making process. |
Use cases | Suitable for applications where AI can handle tasks autonomously but may require human insight for complex or novel issues. | Ideal for scenarios where human expertise is crucial for the task at hand, requiring close collaboration between AI and humans. |
Feedback loop | Feedback is used to improve the AI system over time, focusing on reducing the need for human intervention. | Continuous human feedback is essential for the AI’s operation, with a focus on real-time collaboration and adjustment. |
Objective | To enhance the efficiency and scalability of AI operations while retaining the ability to incorporate human judgment. | To leverage human expertise directly in AI processes, ensuring decisions are informed by human values and knowledge. |
Conclusion
Human-on-the-Loop (HOTL) presents a compelling approach to modern machine learning—one that blends the strengths of human judgment with the speed and efficiency of AI. Instead of relying solely on human input or giving complete control to machines, HOTL establishes a smart middle ground. It empowers AI systems to function autonomously while ensuring human oversight remains a vital part of the loop, stepping in when it matters most.
Throughout this article, we explored the core ideas behind HOTL, its structure, applications, and benefits. We also looked at the challenges, clarified common misconceptions, and compared them to Human-in-the-Loop systems.
In a world where AI continues to evolve rapidly, keeping humans on the loop is not just a technical strategy—it’s a safeguard. It ensures our technologies stay ethical, reliable, and aligned with human values. As we move forward, HOTL will likely play an essential role in shaping AI systems that we can trust, learn from, and work alongside.