Data Labeling Services for ML Models
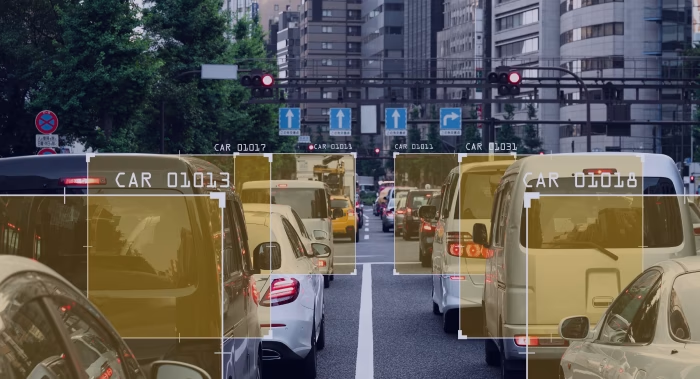
At Unidata, we offer high-quality data labeling services tailored for machine learning projects. Our expert team provides precise tagging to help you build robust models, ensuring optimal quality and performance with advanced tools and extensive expertise.
Trusted by the world’s leading tech brands
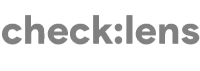
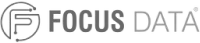
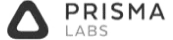
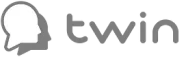

24/7*
- 6+
- years experience with various projects
What is Data Labeling?
Data labeling is the process of categorizing data to prepare it for machine learning and artificial intelligence applications. This essential step involves assigning meaningful labels or tags to various types of data, such as images, text, audio, and video, enabling algorithms to learn from the information accurately. High-quality data labeling enhances the performance of machine learning models by providing clear and structured inputs, allowing organizations to derive actionable insights and drive innovation.How we deliver data labeling services
The best software for data labeling tasks
Types of data labeling services
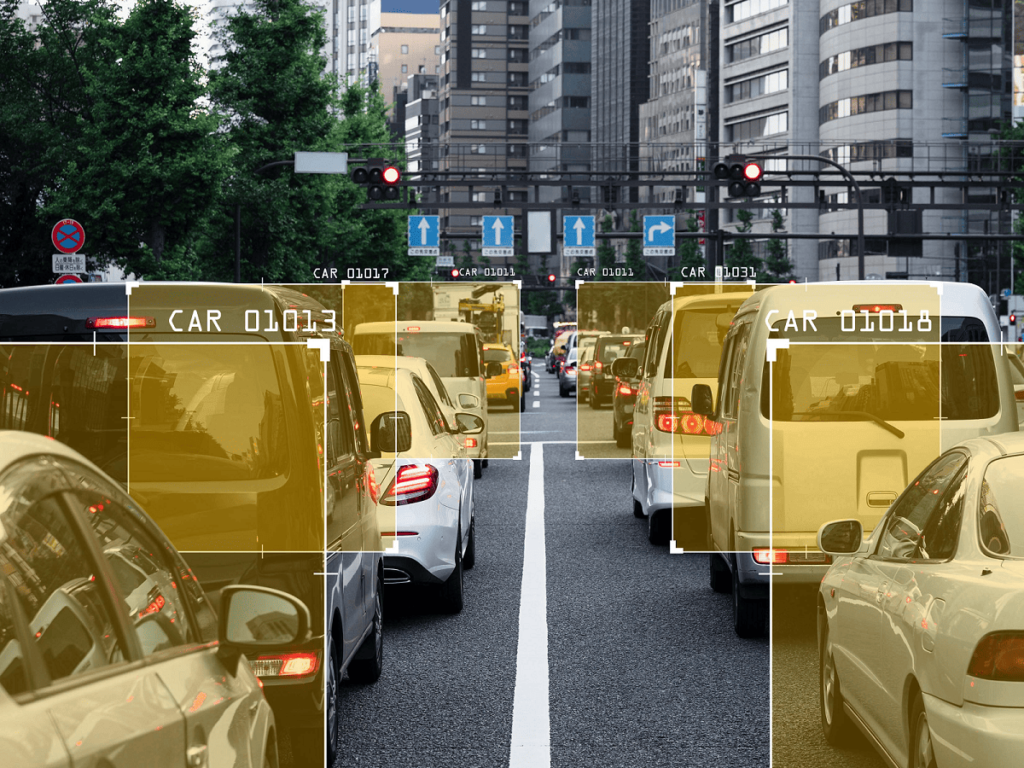
Image Annotation
Image annotation involves labeling objects within images to train machine learning models for tasks like object detection, classification, or segmentation. Common forms of image annotation include: Bounding Boxes: Drawing rectangles around objects of interest within an image. Polygon Annotation: Creating precise shapes around irregular objects for higher accuracy. Semantic Segmentation: Assigning each pixel in an image to a particular class or label. Keypoint Annotation: Marking specific points of interest, such as facial landmarks or body joints.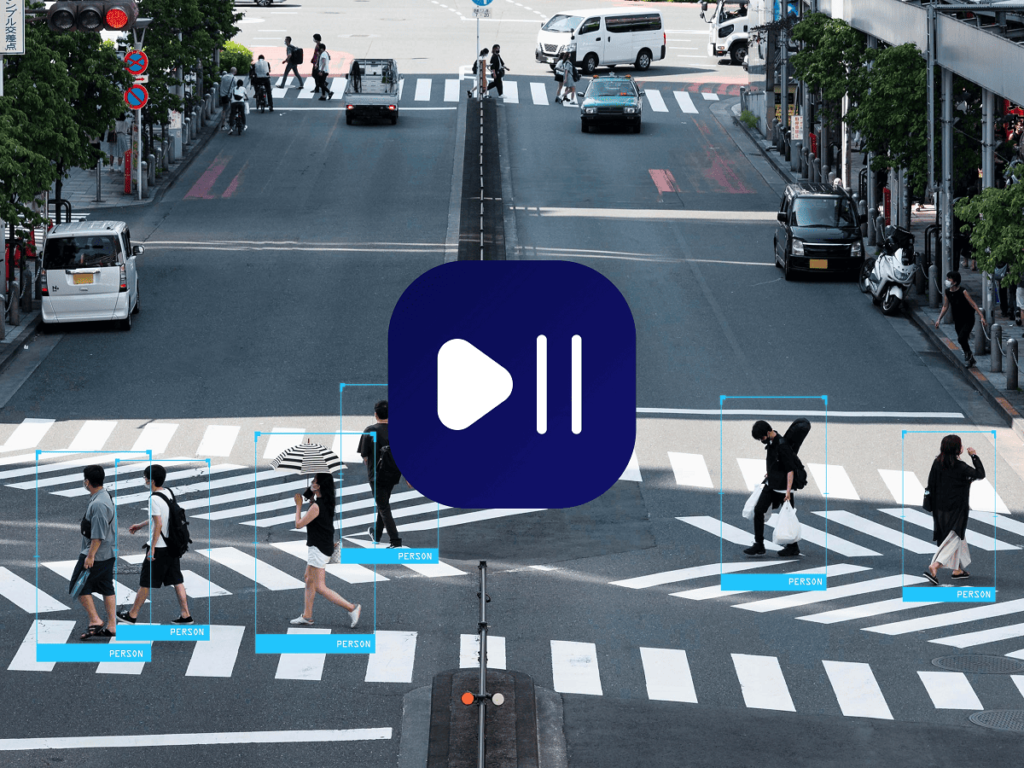
Video Annotation
Video annotation is used to label objects frame by frame within video data, enabling machine learning models to recognize and track objects over time. This is critical for applications like autonomous driving, security surveillance, and sports analytics. Types of video annotation include: Object Tracking: Labeling objects and following their movements across frames. Event Detection: Identifying and labeling specific events or actions within the video. Frame Classification: Labeling each video frame according to predefined categories.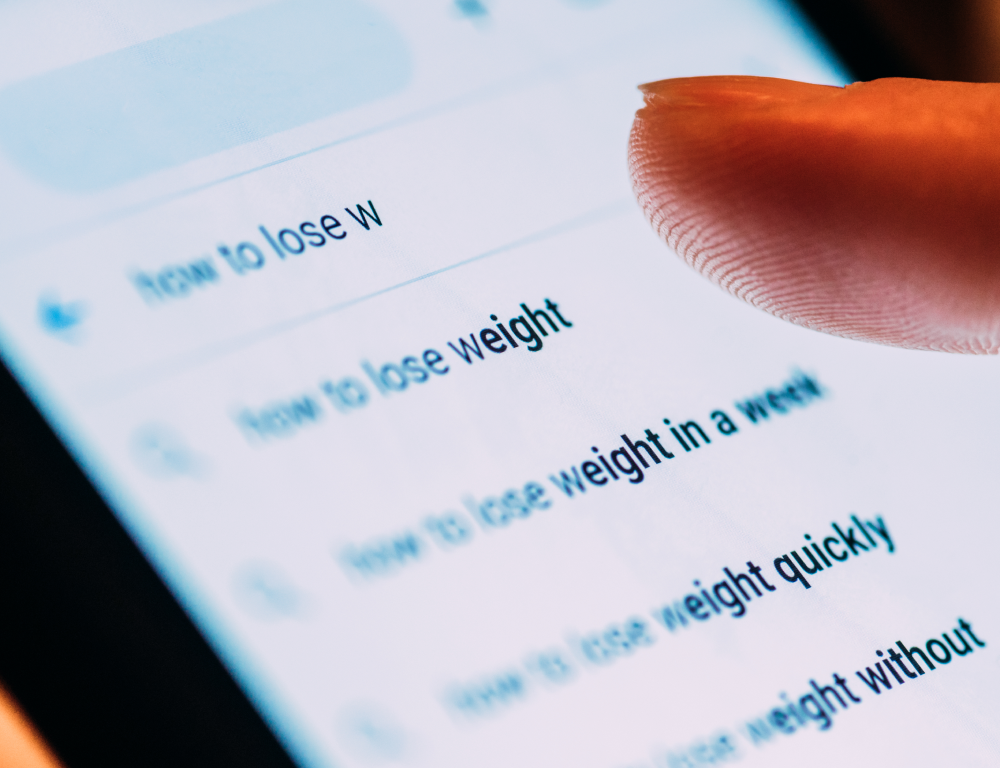
Text Annotation
Text annotation involves labeling segments of text data to help models understand language and context. This is essential for natural language processing (NLP) tasks. Common forms include: Entity Recognition: Identifying and labeling entities such as names, locations, or organizations within text. Sentiment Annotation: Categorizing text based on its emotional tone (positive, negative, or neutral). Text Classification: Assigning predefined categories or labels to entire text documents or segments.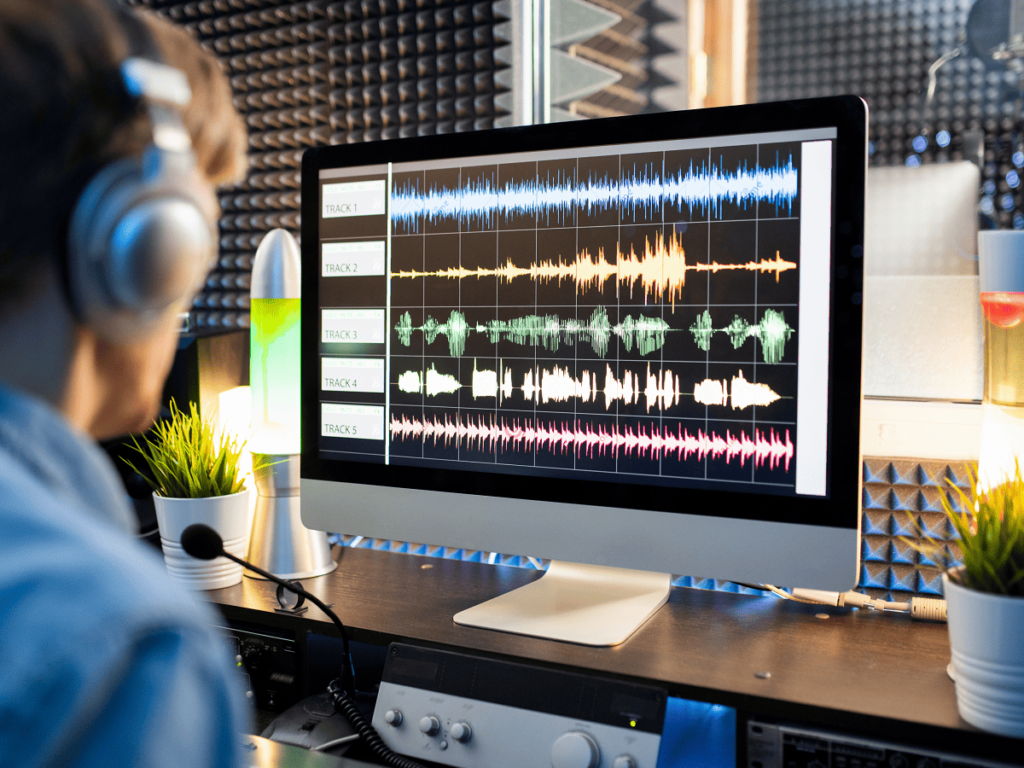
Audio Annotation
Audio annotation is the process of labeling sound data to train models for speech recognition, language processing, or sound classification. This can involve: Speech-to-Text Annotation: Transcribing spoken words into text. Speaker Identification: Labeling different speakers within an audio file. Sound Classification: Categorizing audio clips into classes such as music, noise, or specific sound events (e.g., sirens, applause).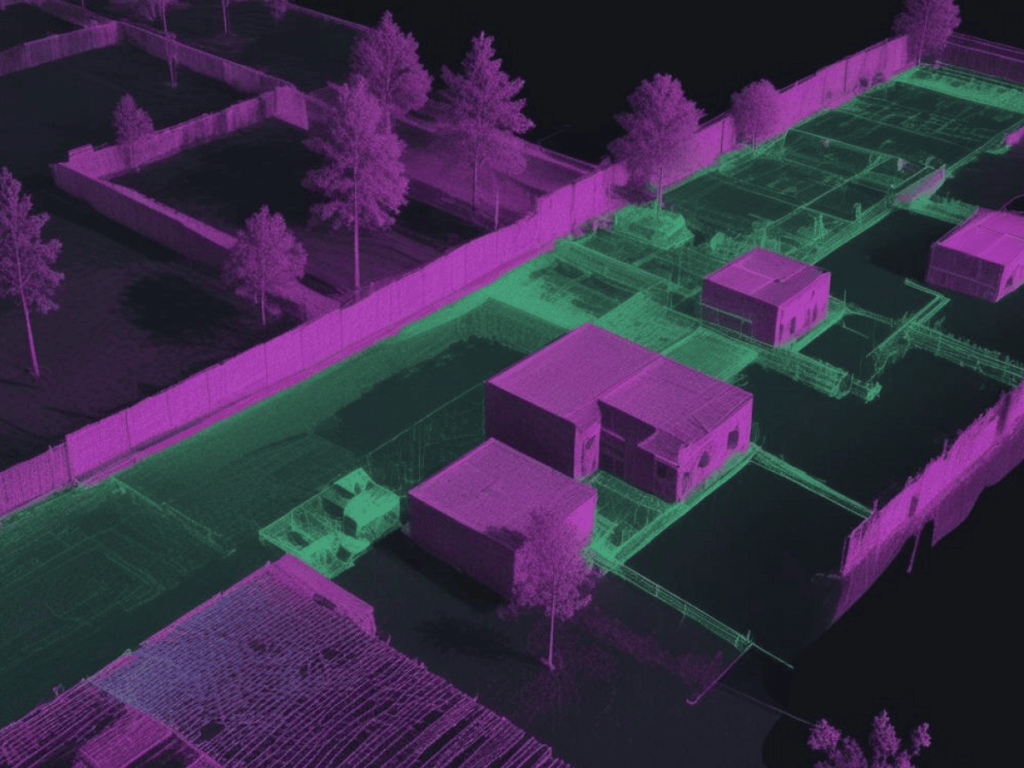
3D Point Cloud Annotation
3D point cloud annotation is used to label data generated by LiDAR sensors, commonly used in autonomous vehicles, robotics, and mapping. Types of 3D annotation include: 3D Bounding Boxes: Drawing three-dimensional boxes around objects in point cloud data. Point-Level Annotation: Labeling individual points in the cloud data for detailed object classification. Segmentation: Grouping points in the cloud data into specific categories or classes (e.g., vehicles, pedestrians, road signs).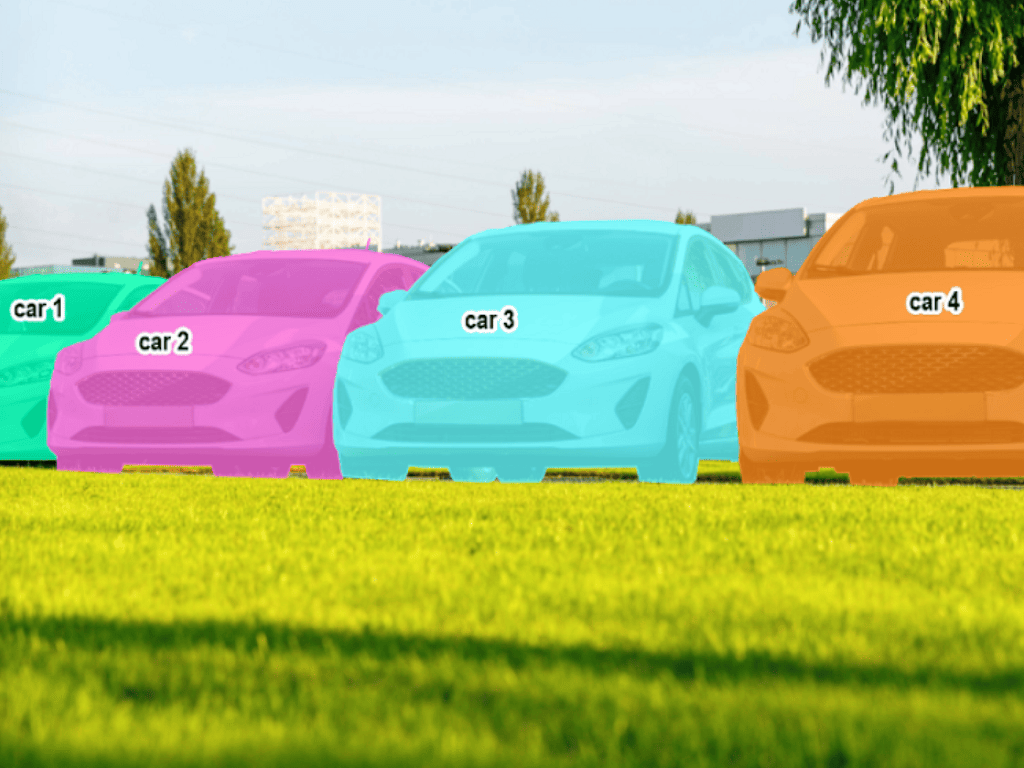
Image and Video Classification
This form of data labeling involves categorizing images or video frames into predefined categories without specifically annotating the objects within them. It is used for applications like scene classification, product categorization, or identifying actions in video.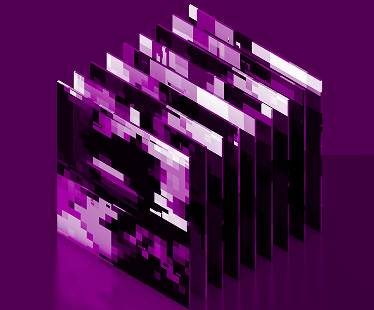
Entity Annotation for Structured Data
This involves labeling structured data formats, such as tables or spreadsheets, to categorize and tag specific entities or values within the data. This type of annotation is often used in machine learning for financial analysis, business intelligence, and other data-heavy applications.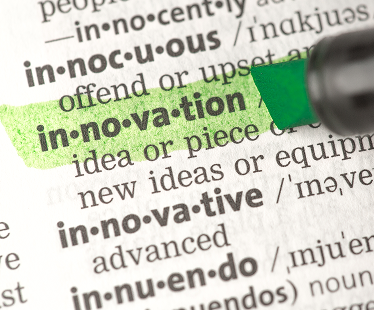
OCR Annotation (Optical Character Recognition)
OCR annotation involves labeling characters, words, or blocks of text within images or scanned documents. It is used to train models that convert visual text into machine-readable formats, often used in document digitization and automation.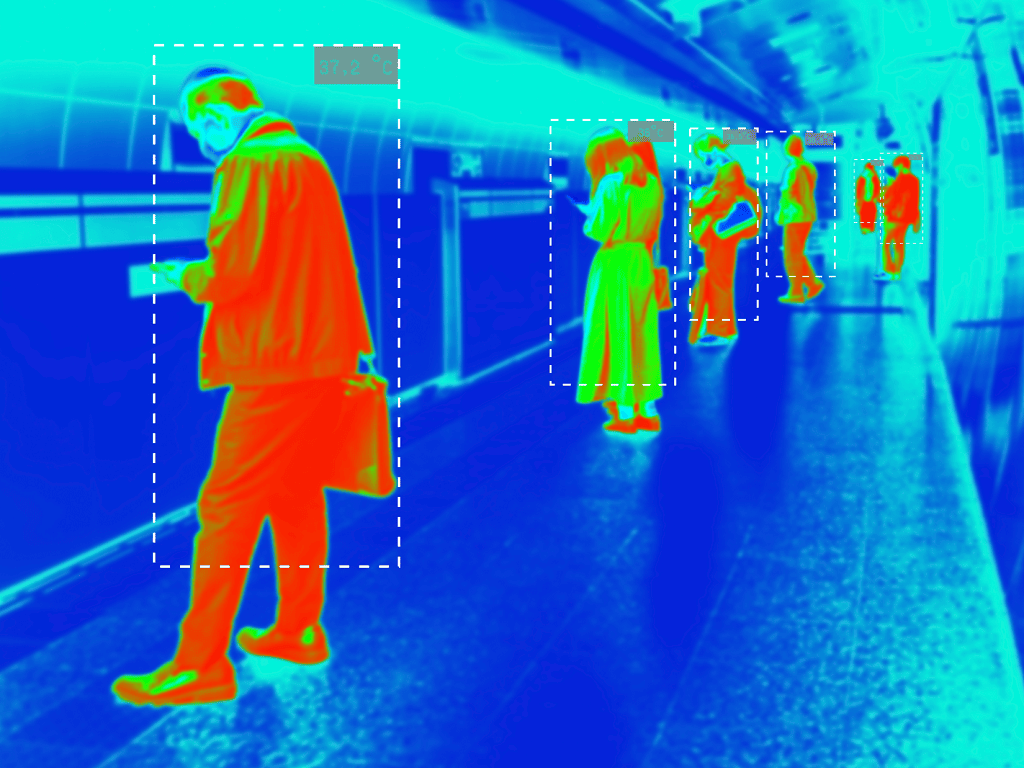
Sensor Data Annotation
This form of annotation involves labeling data from various sensors, such as accelerometers, gyroscopes, or GPS. This type of data labeling is particularly important for applications like wearable device analysis, IoT (Internet of Things), and activity recognition.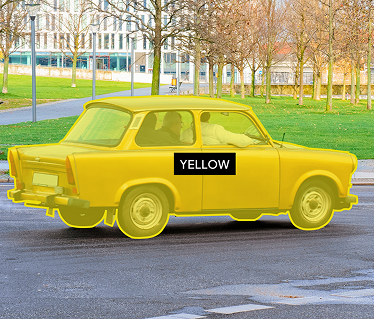
Attribute Annotation
Attribute annotation involves adding additional metadata to labeled objects, such as the color of a car, the breed of an animal, or the sentiment of a piece of text. It enhances the depth of the annotation by providing more context for the labeled data. Each type of data labeling service plays a critical role in building machine learning models tailored to specific tasks across industries such as autonomous driving, healthcare, finance, e-commerce, and more.Data Labeling Use Cases
-
01
Healthcare
Data labeling helps AI understand medical images, such as X-rays and MRIs, in healthcare, by tagging regions with abnormalities like tumors or fractures. Annotating patient records and clinical notes enables AI to track conditions over time, predict outcomes, and recommend personalized treatment plans. This process supports better diagnosis and faster decision-making in patient care. -
02
Automotive (Autonomous Vehicles)
This service is important for training AI to navigate roads safely. Labeling objects such as pedestrians, vehicles, and traffic signs allow AI systems to identify and react to these objects in real time. Annotating road conditions and lane markings helps improve vehicle navigation while tagging pedestrian movement enables the vehicle to avoid accidents by predicting potential dangers. -
03
Retail & E-commerce
For e-commerce businesses, labeling improves product searches and recommendations by categorizing product images and descriptions with attributes like color, size, and brand. Labeling customer feedback and reviews allows AI to assess consumer sentiment, helping businesses personalize marketing strategies and optimize inventory management. -
04
Agriculture
In agriculture, data annotation helps monitor crop health by tagging satellite and drone images to identify signs of diseases, pests, or poor soil conditions. Labeling crops, weeds, and other elements in images enables AI to differentiate between beneficial plants and harmful ones, improving pest management and crop yield. Annotating images of livestock supports monitoring animal health and behavior, leading to better farm management. -
05
Finance
Data labeling in finance helps AI detect fraudulent activities by tagging transaction data with details like account numbers, transaction amounts, and timestamps. Annotating financial documents such as invoices and contracts allows AI to extract and process relevant data more efficiently. Labeling customer profiles with information like credit scores and transaction history aids in improving credit assessments and loan decisions. -
06
Security & Surveillance
In security and surveillance, this service helps improve facial recognition systems by tagging faces and key identifiers in video footage. Labeling objects like vehicles, suspicious movements, and areas of interest enables AI to detect potential threats in real time, ensuring faster responses to security breaches. This enhances surveillance systems and provides valuable insights for law enforcement. -
07
Manufacturing
In manufacturing, these techniques are used to detect defects in products by tagging images from assembly lines with details about imperfections like scratches, dents, or misalignments. Annotating sensor data from machinery helps predict potential failures and schedule maintenance, while labeling assembly steps, enables robots to perform tasks more efficiently, reducing errors and improving production processes. -
08
Entertainment & Media
Data labeling helps content moderation systems detect inappropriate material in videos and images. Labeling scenes and characters enables AI to improve content recommendations based on user preferences. Annotating videos with time-stamped captions makes content more accessible, and performing sentiment analysis on media content helps brands adjust marketing strategies according to audience reactions.
Ready to get started?
Tell us what you need — we’ll reply within 24h with a free estimate
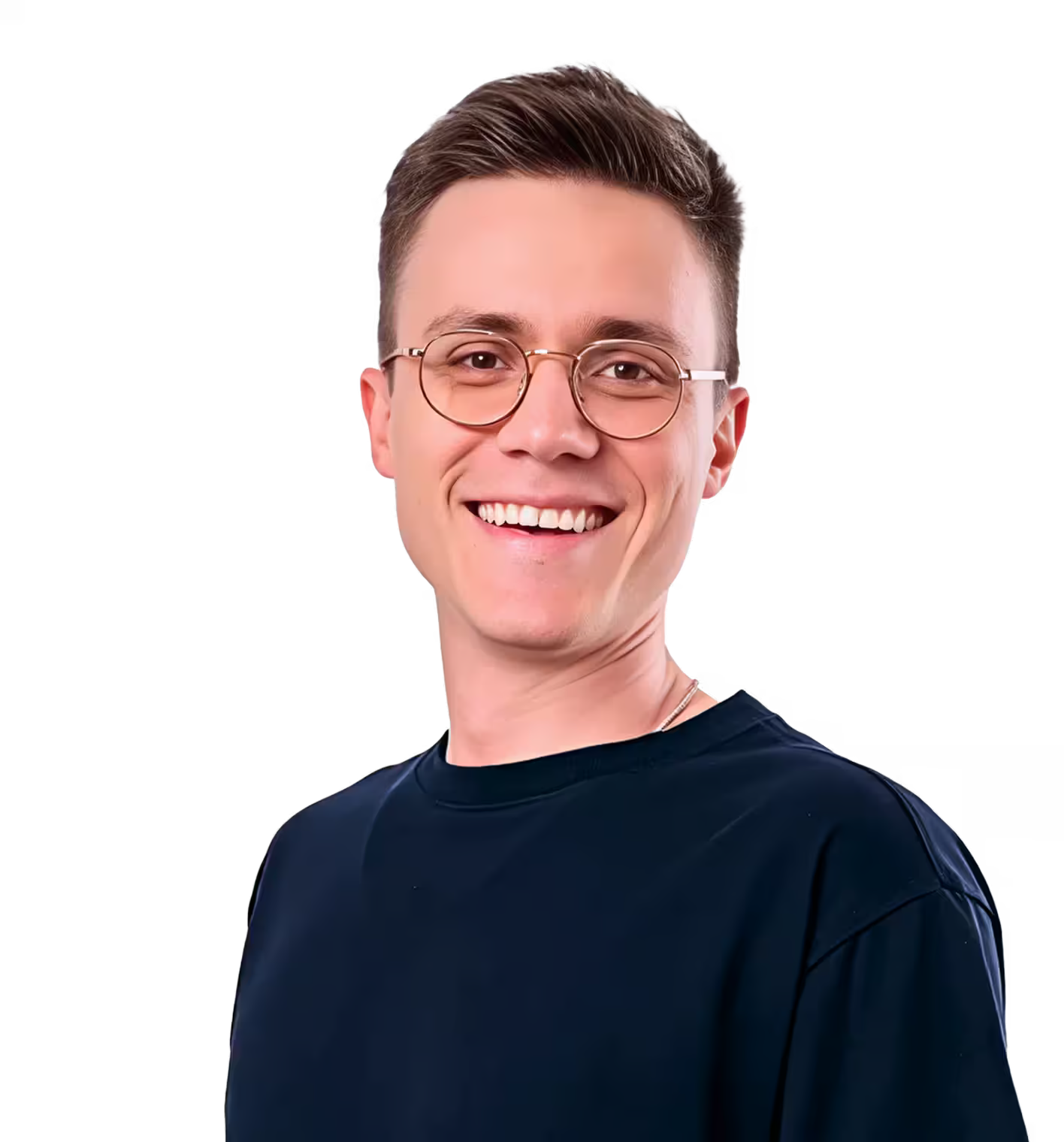
- Andrew
- Head of Client Success
— I'll guide you through every step, from your first
message to full project delivery
Thank you for your
message
This website uses cookies to enhance your experience, analyze traffic, and deliver personalized content and ads. By clicking "Accept", you consent to the use of cookies, as described in our Cookie Policy. Please choose your cookie preference.