Data collection and video annotation: weapon detection on the streets
System enabled recognition of 99% of weapon images of people on streets and indoors
- Industry and use case:
- Video systems and video analysis
- Data:
- 100 hours of video for annotation
- Project duration:
- 28 days
Task:
The client needed to create a system for detecting weapons on people in the streets of a large city. This required creating the necessary data domain and detecting weapons in open areas and city streets. There were no open datasets with weapons available, and parsing provided an insufficient amount of data. The client did not know how to organize the data collection process, so they contacted the Unidata team.
Solution:
To create the necessary data domain, we formed several groups of extras, provided them with prop weapons of different sizes, and planned routes in the city to ensure that the extras would frequently appear on external surveillance cameras.
Each extra was filmed in 10 scenes: 5 indoors and 5 outdoors. After that, the videos from the cameras were collected and given to the team of assessors for bounding box detection.
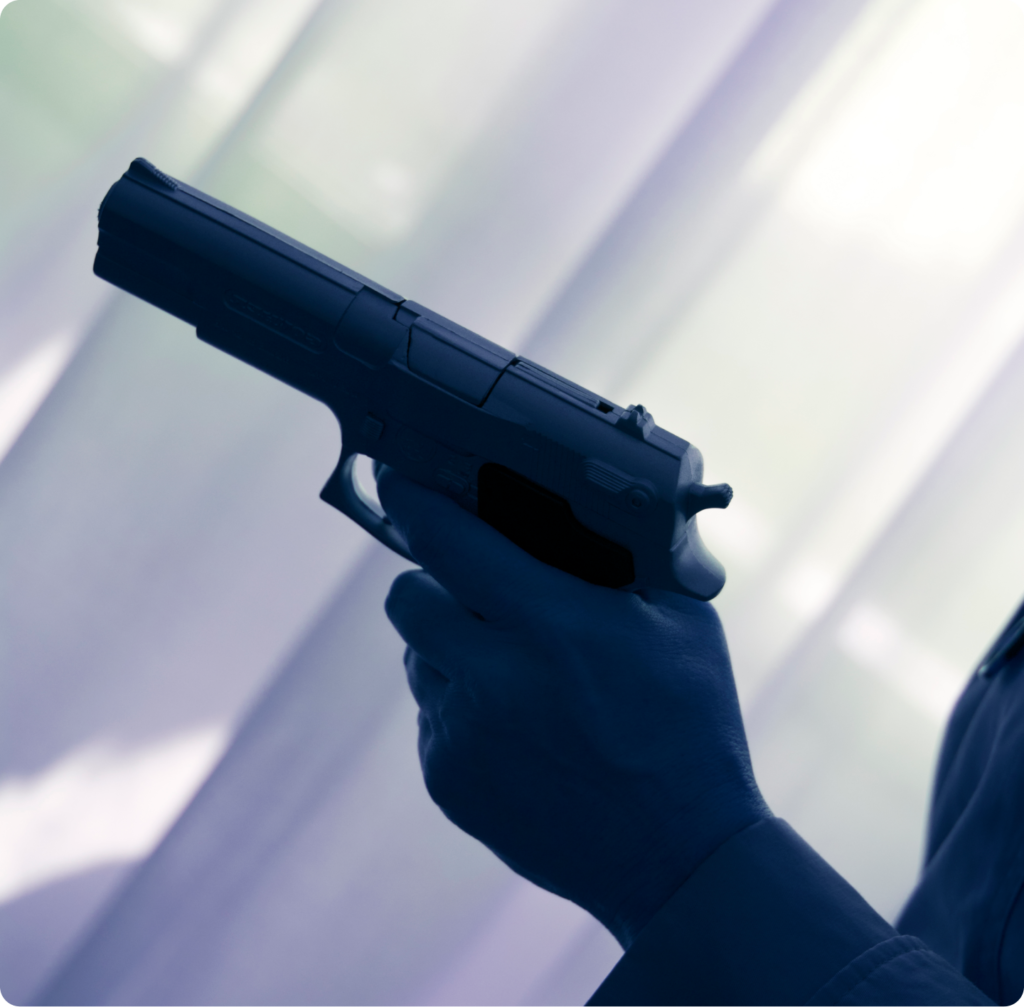
Results:
- The client received a quality dataset according to the specifications and trained a neural network with a 99% weapon detection accuracy.
- A process for collecting staged videos and photos from scratch in a short time frame was organized, and the experience was documented for similar tasks.
Our Cases
-
Within a month, more than 10% of the entire database with over 2,000 photographs was collected
-
Through data collection, the client improved their biometric system for facial and voice recognition by 21%.
-
Content moderation on the video hosting platform enabled a 99% reduction in the influx of prohibited content
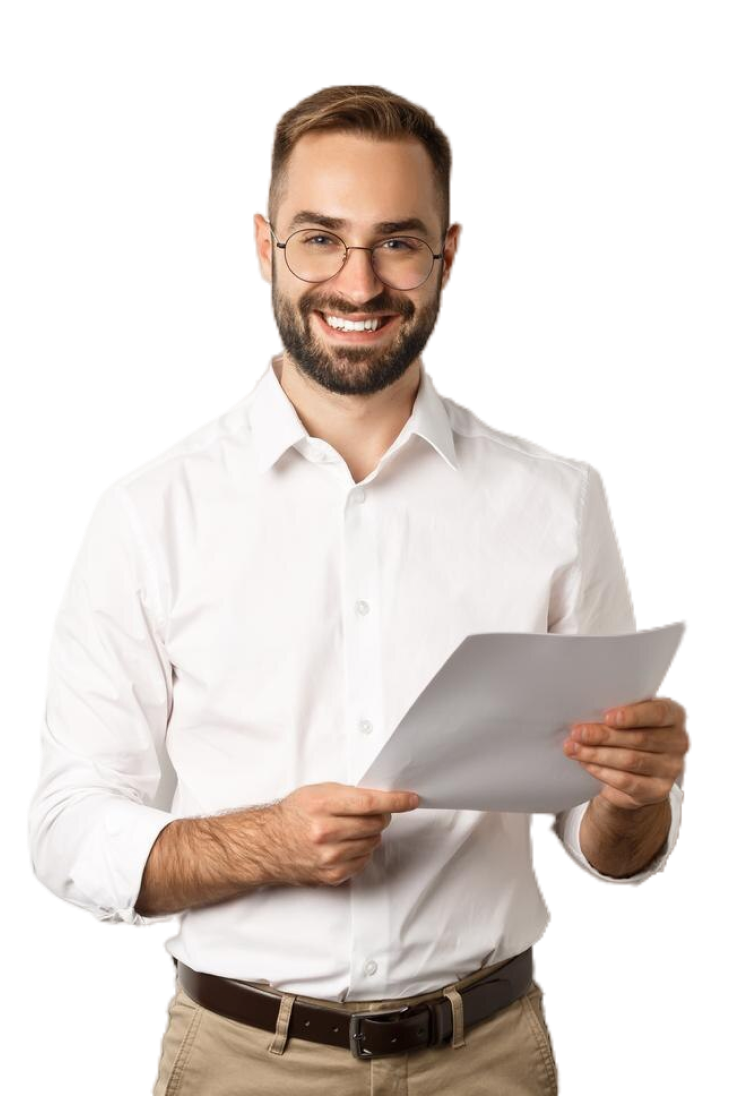