Audio Labeling services for ml
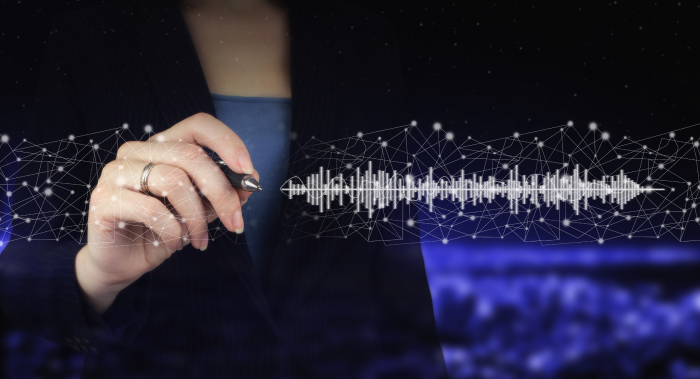
Unidata offers professional audio labeling services that provide precise annotation and labeling of audio data to enhance speech recognition, transcription, and audio analysis across diverse industries.
Our skilled annotators meticulously label audio recordings with crucial information, including speaker identification, transcription, and acoustic events, ensuring high-quality data for your projects
Trusted by the world’s leading tech brands
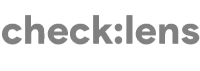
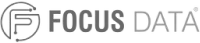
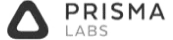
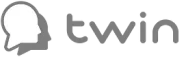
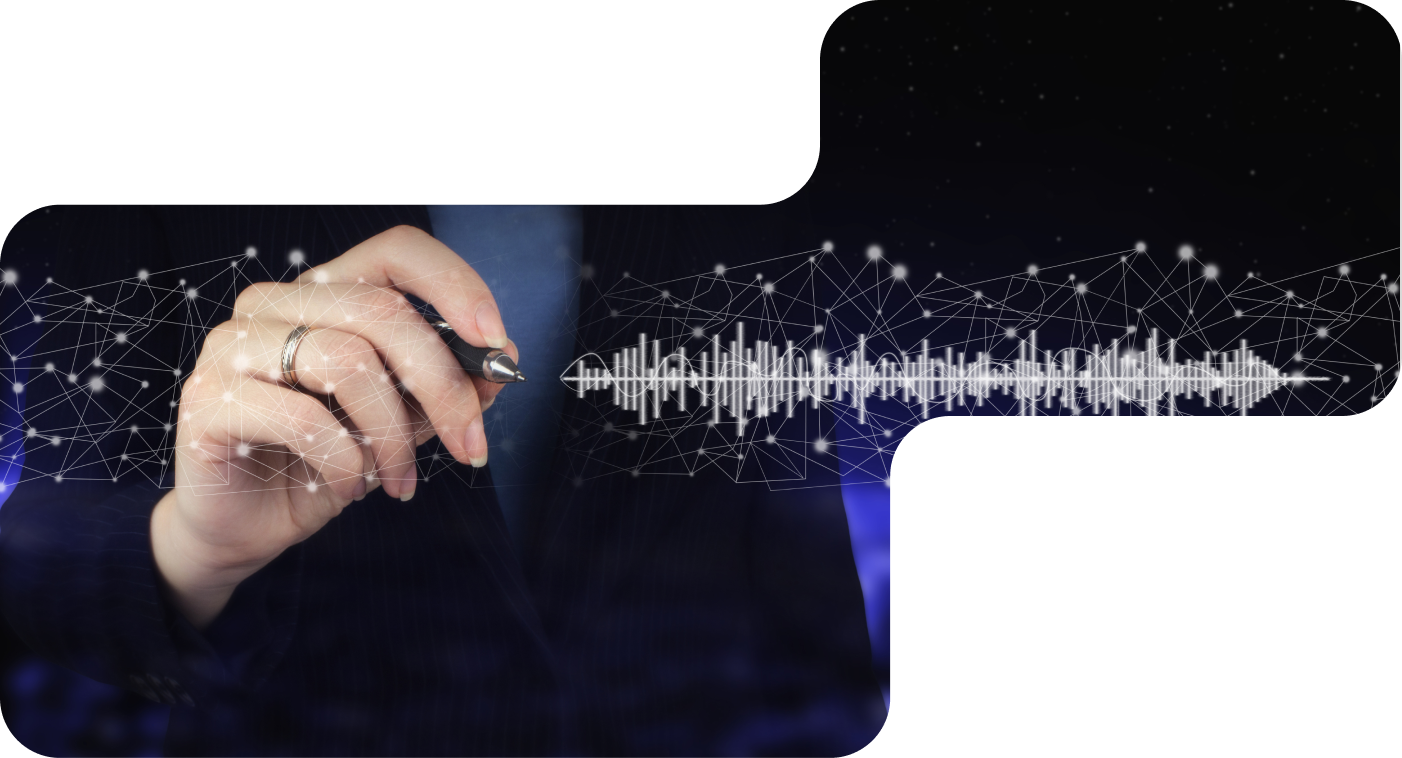
24/7*
- 6+
- years experience with various projects
- 79%
- Extra growth for your company.
What is Audio Labeling?
Audio labeling is the process of annotating and tagging audio data to enhance its usability for machine learning and artificial intelligence applications. This involves identifying and categorizing elements within audio recordings, such as speech transcription, speaker identification, and acoustic events. Audio labeling is crucial for improving the performance of speech recognition systems, transcription services, and audio analysis tools.How we deliver audio labeling services
The best software for audio labeling tasks
Types of audio labeling services
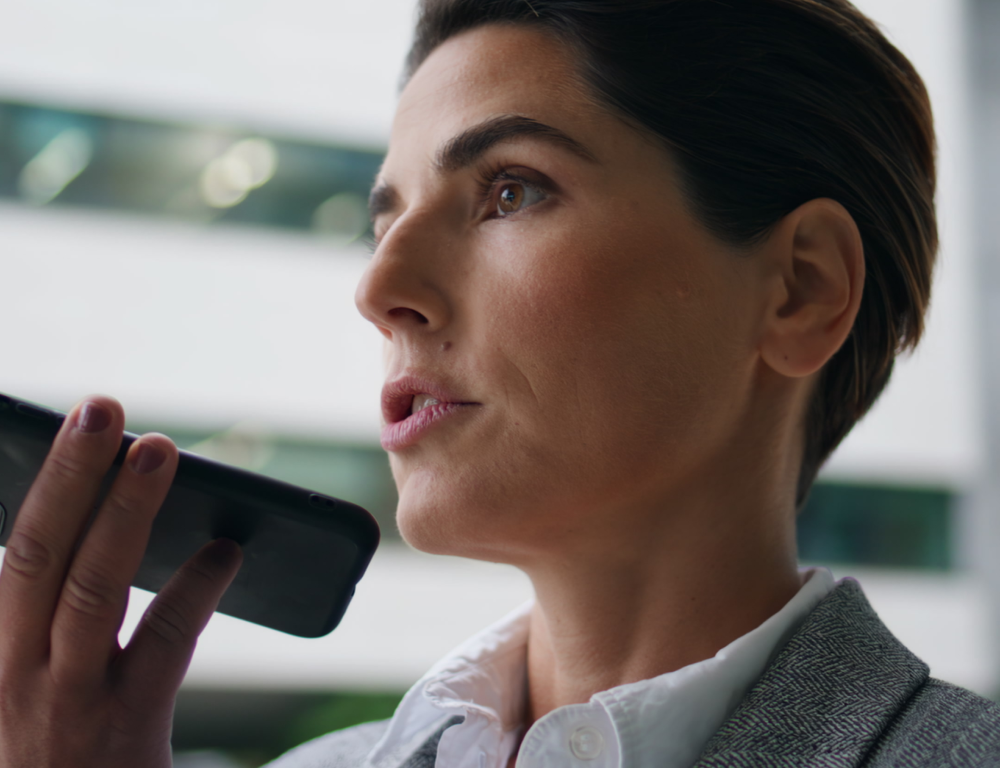
Speech-to-Text Transcription
This form of audio labeling involves converting spoken language in audio files into written text. It is often used in applications such as voice assistants, transcription services, and speech recognition models. Transcriptions can be done verbatim (word-for-word) or with some level of cleaning to remove filler words and hesitations.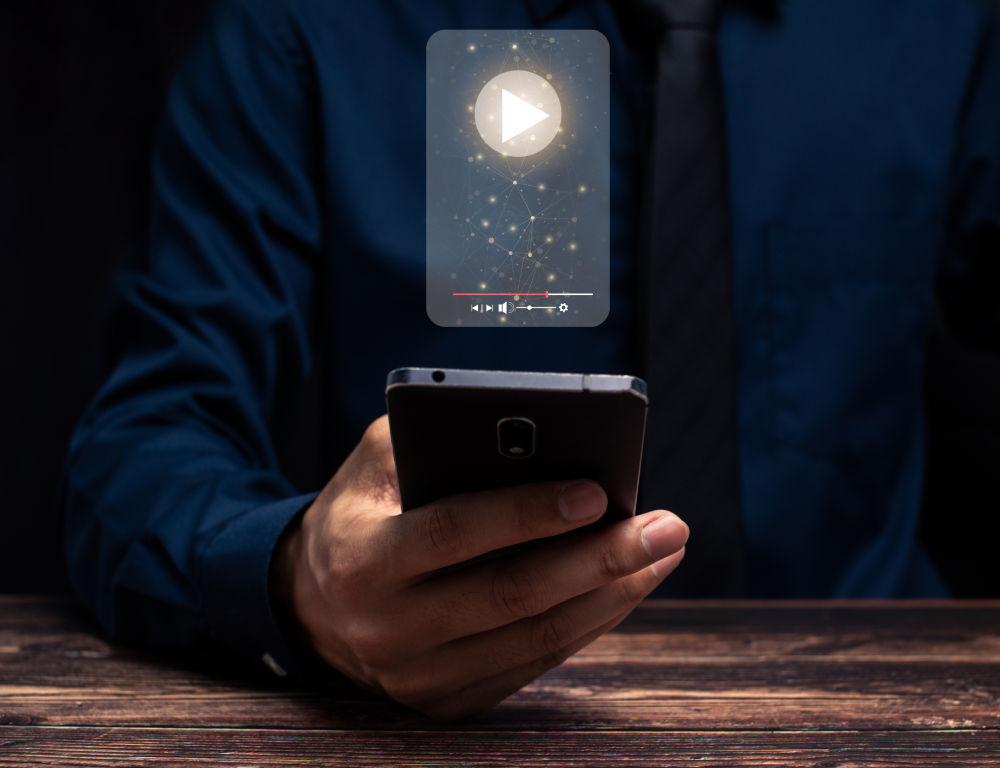
Speaker Diarization
Speaker diarization refers to labeling audio data to identify when speakers change within a conversation. The process involves segmenting the audio and assigning a unique label to each speaker. This is critical in multi-speaker environments, such as meetings, interviews, or podcasts, and helps machine learning models recognize and differentiate between speakers.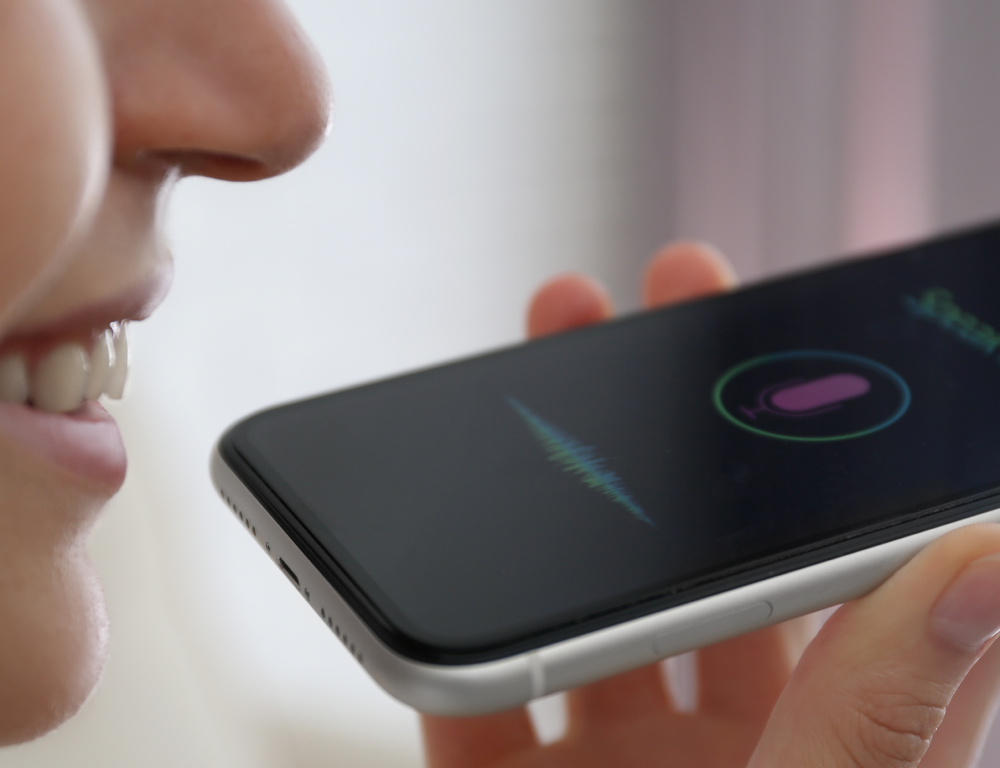
Emotion Labeling
Emotion labeling involves annotating the emotional tone of the speaker’s voice within audio data. Emotions such as happiness, anger, sadness, or neutrality are tagged to specific segments of the audio. This type of labeling is important for applications in sentiment analysis, customer service, and virtual assistants.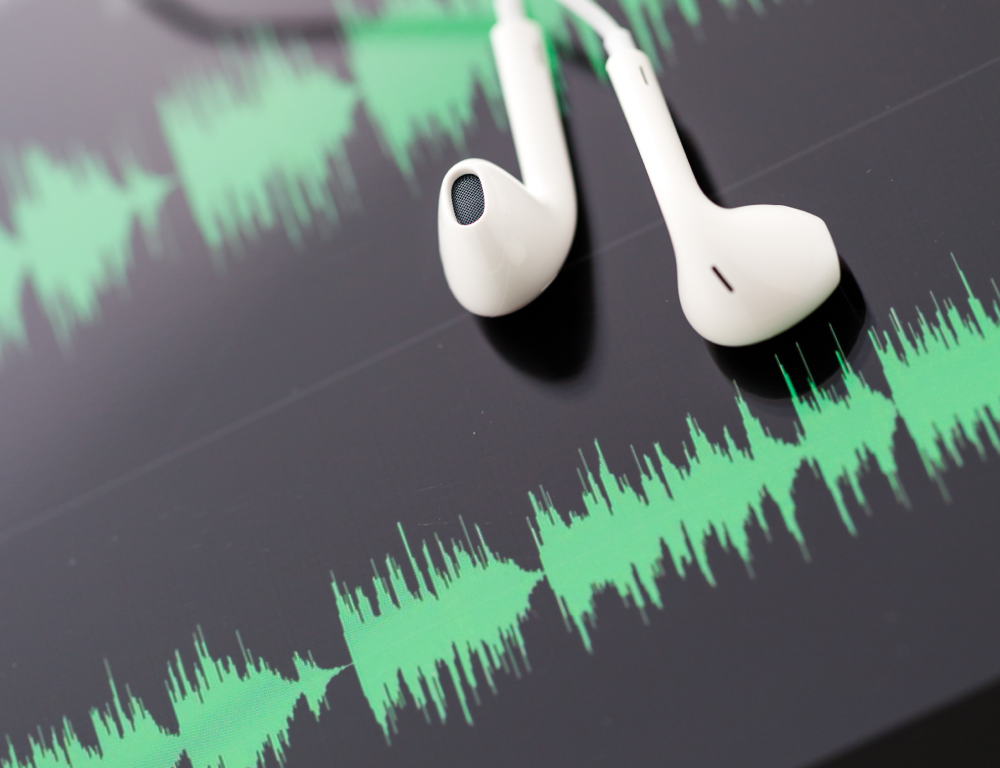
Sound Event Detection
In sound event detection, audio segments are labeled with specific sound events, such as clapping, coughing, sirens, or doorbells. This form of labeling is used in projects that require machine learning models to detect and classify environmental sounds, such as smart home devices, security systems, or audio surveillance.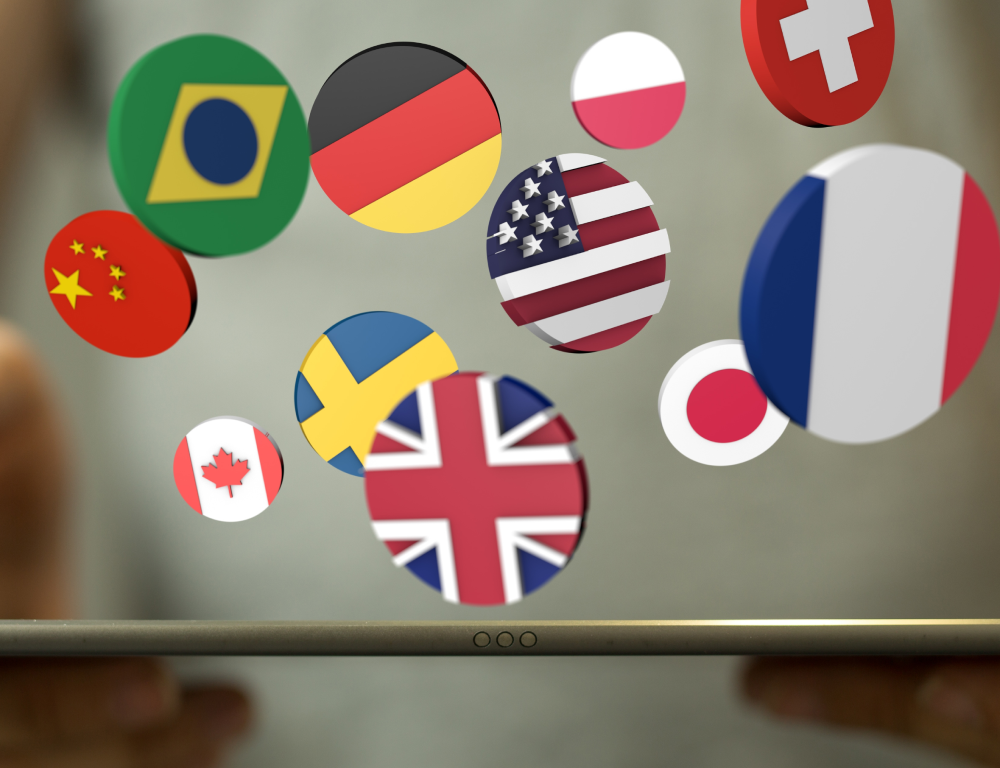
Phoneme Labeling
Phoneme labeling involves annotating the smallest units of sound in speech, known as phonemes, which represent individual sounds that make up words. This type of annotation is typically used in linguistics research and speech synthesis technologies, such as text-to-speech systems.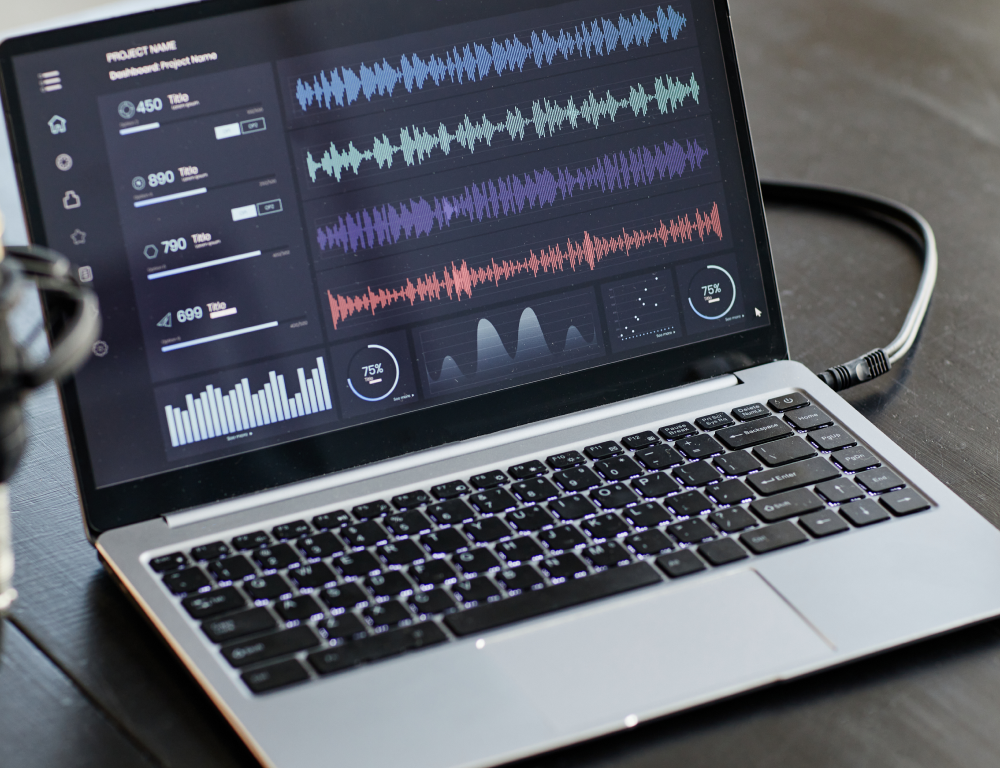
Word Timing Annotation
This type of labeling provides precise timestamps for when specific words or phrases occur within the audio. It’s often used in conjunction with speech-to-text transcription to help synchronize audio with text for use in closed captioning, subtitles, or AI-driven search functions in media.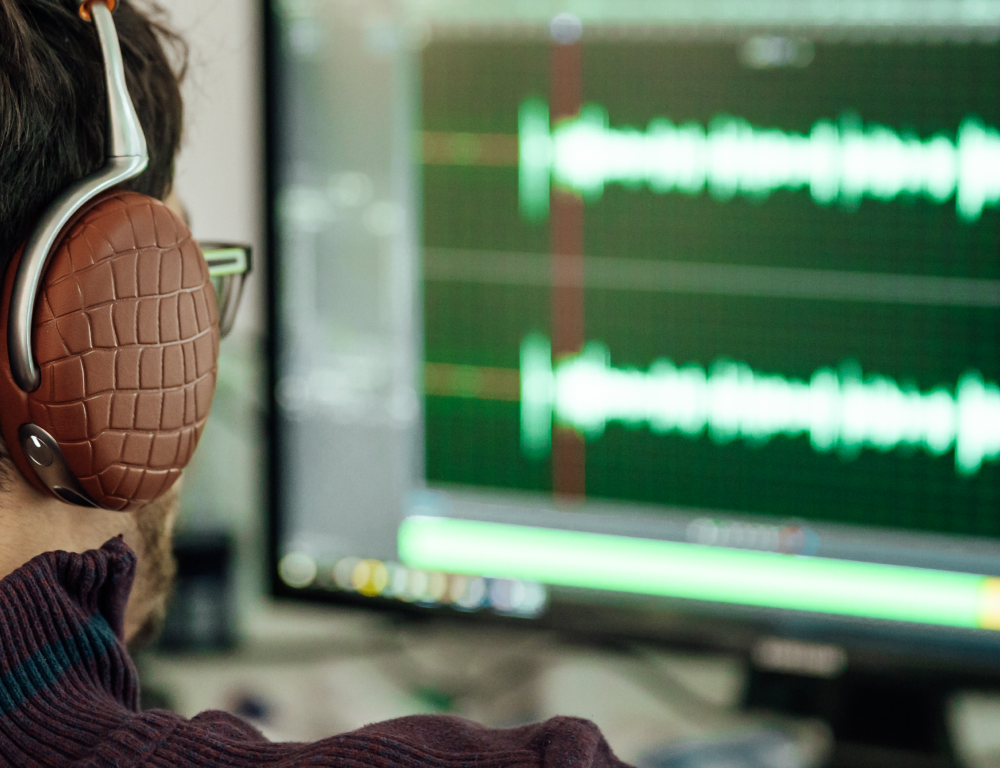
Language Identification
Language identification involves labeling audio segments with the language being spoken. This service is used in multilingual datasets where it's important to identify and distinguish different languages within a single audio file. It is commonly applied in language processing tools, call centers, and language translation applications.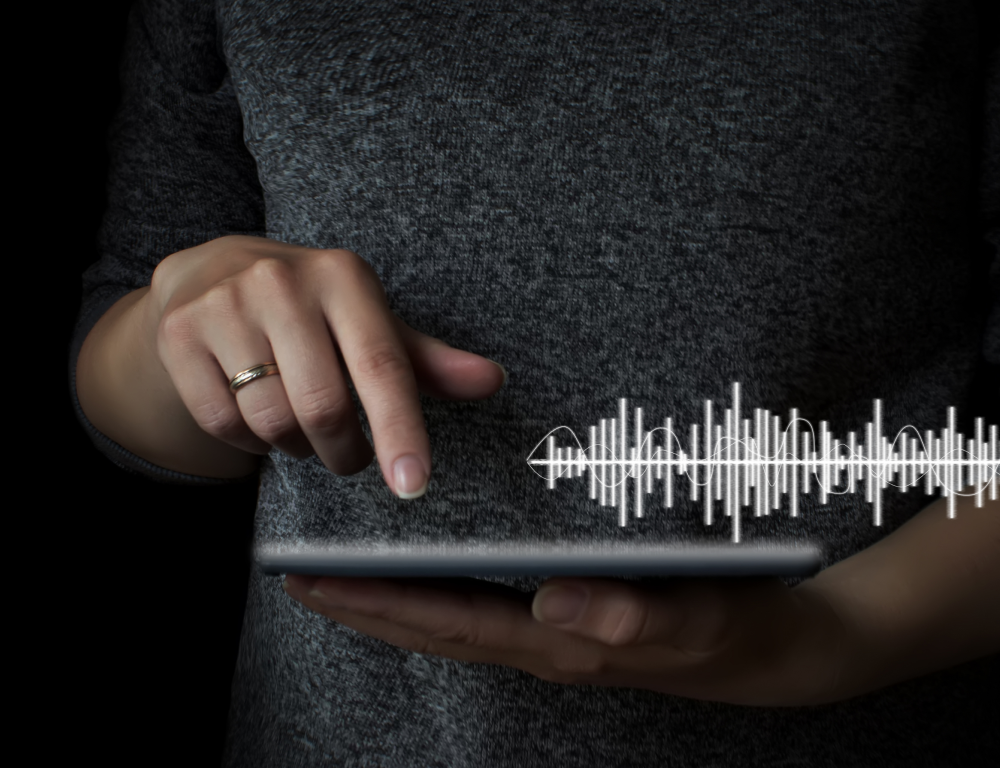
Acoustic Scene Classification
Acoustic scene classification involves labeling audio data based on the environment or scene in which the sound was recorded. Examples include identifying whether a recording was made in a park, office, city street, or home environment. This type of labeling is valuable for context-aware systems and urban sound mapping.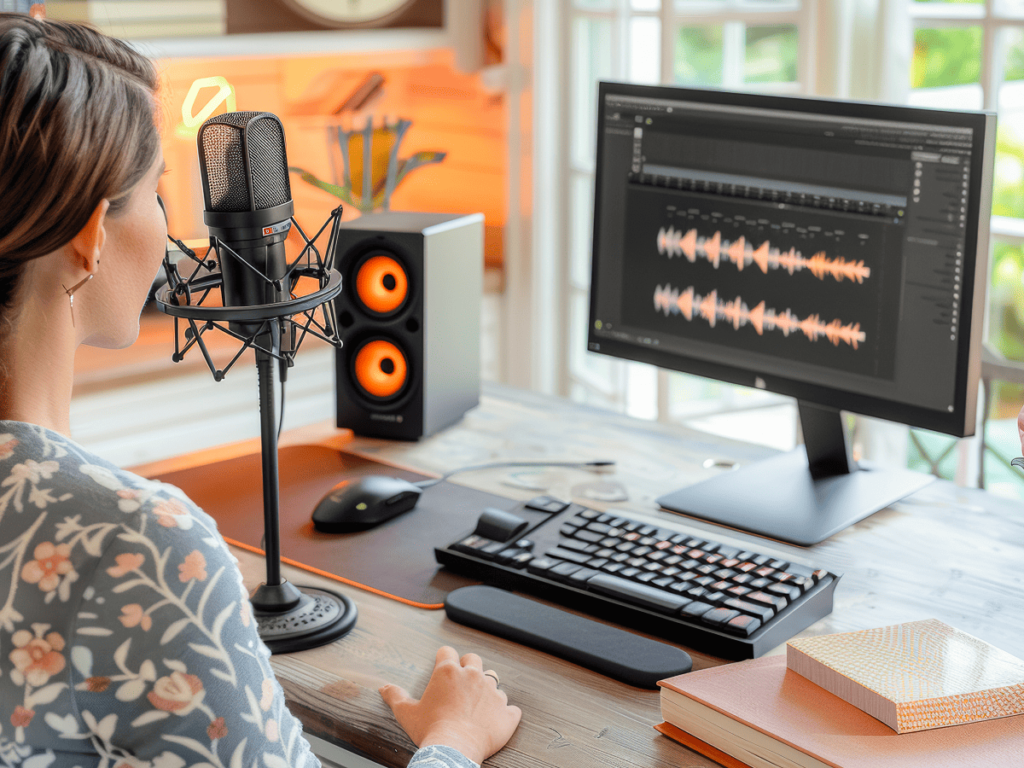
Noise Labeling
Noise labeling annotates non-speech elements within an audio file, such as background noise, static, or other auditory interferences. This type of labeling is useful in applications where it's important to detect and filter out noise from useful audio signals, such as in hearing aids, telecommunication systems, or audio enhancement software.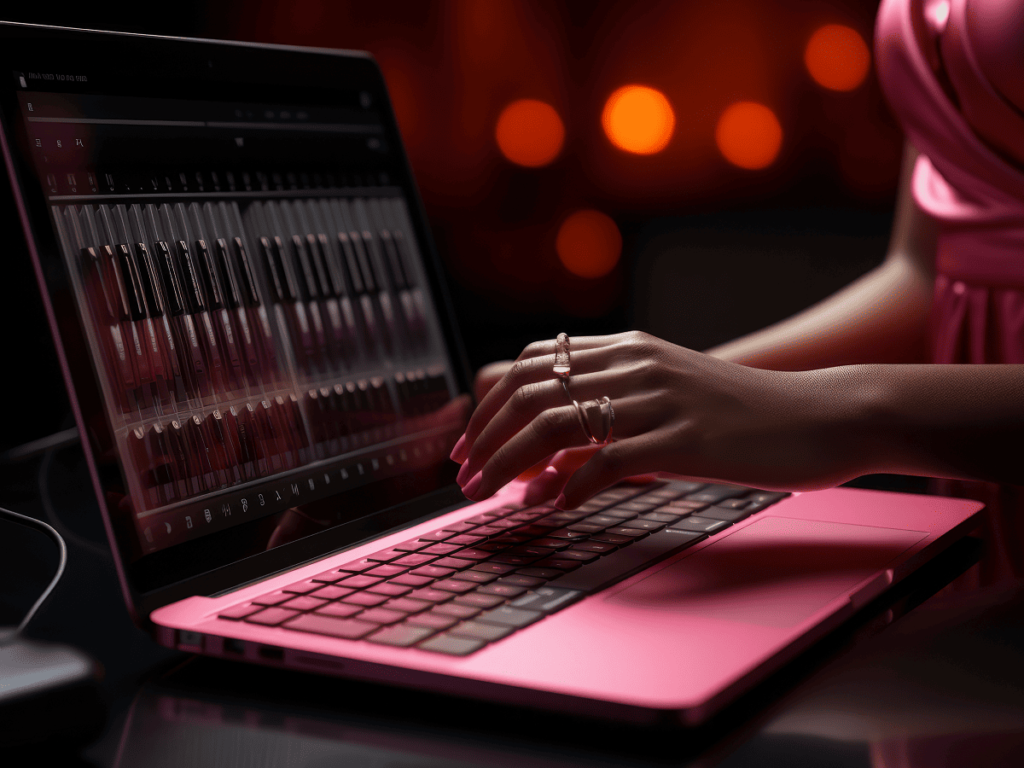
Music Annotation
Music annotation involves labeling components of music tracks, such as identifying instruments, tempo, genre, or specific segments like chorus and verse. This type of labeling is widely used in music recommendation systems, audio search engines, and automated music analysis tools.Audio Labeling Use Cases
-
01
Healthcare
In healthcare, audio labeling annotates data from doctor-patient interactions, medical consultations, and diagnostic recordings. Labeling specific terms like symptoms, diagnoses, and medical history helps AI systems recognize important information, enabling faster and more accurate decision-making. Annotating patient conversations also assists in predicting health outcomes and streamlining administrative tasks. -
02
Automotive (Autonomous Vehicles)
This technology helps to train AI to understand and respond to voice commands, environmental sounds, and traffic-related audio. Labeling sounds such as sirens, honking, or pedestrian voices enables AI to react in real time to dynamic road conditions. Annotating audio data related to in-vehicle conversations or commands also enhances the vehicle’s ability to interact with passengers and navigate safely. -
03
Retail & E-commerce
This service improves customer service by analyzing voice interactions between customers and service agents. Labeling audio data from customer queries, complaints, and product inquiries helps AI understand intent and provide more accurate responses. Annotating customer feedback and product-related audio also helps enhance recommendation systems and optimize voice search capabilities on e-commerce platforms. -
04
Agriculture
In agriculture, audio labeling helps to monitor and analyze sounds in farming environments, such as animal behavior, machinery noise, or environmental sounds. By labeling animal sounds, AI can detect distress signals or health issues. Annotating machinery and equipment sounds also helps identify malfunctions or inefficiencies, allowing for predictive maintenance and improved farming practices. -
05
Finance
Such labeling methods are used to transcribe and analyze customer service calls, investment meetings, and financial discussions. By labeling keywords and financial terms in conversations, AI can extract valuable insights, improve customer support, and detect fraud. Annotating audio data from earnings calls and financial reports also helps in assessing market trends and making informed decisions. -
06
Security & Surveillance
Audio annotation is crucial in security and surveillance systems for identifying key sounds such as gunshots, alarms, or unauthorized access. Labeling audio from surveillance cameras or emergency calls enables AI to detect and alert security teams to potential threats in real time. Annotating sounds related to suspicious activity, like breaking glass or yelling, enhances threat detection and response times. -
07
Manufacturing
In manufacturing, tagging is used to monitor machinery and production lines. By annotating sounds such as machinery malfunctions, irregular vibrations, or alarms, AI can help detect potential issues before they lead to downtime. Labeling audio data from workers’ communications also assists in safety protocols and improves operational efficiency by ensuring that critical alerts are quickly recognized. -
08
Entertainment & Media
In the entertainment industry, this technique is used to analyze dialogue, sound effects, and music in videos or movies. Labeling voice data, background sounds, or specific themes in dialogues helps improve transcription accuracy and enables better content categorization. Annotating music and sound effects also helps improve audio search functionality and recommendation systems for streaming platforms.
Other Services
Ready to get started?
Tell us what you need — we’ll reply within 24h with a free estimate
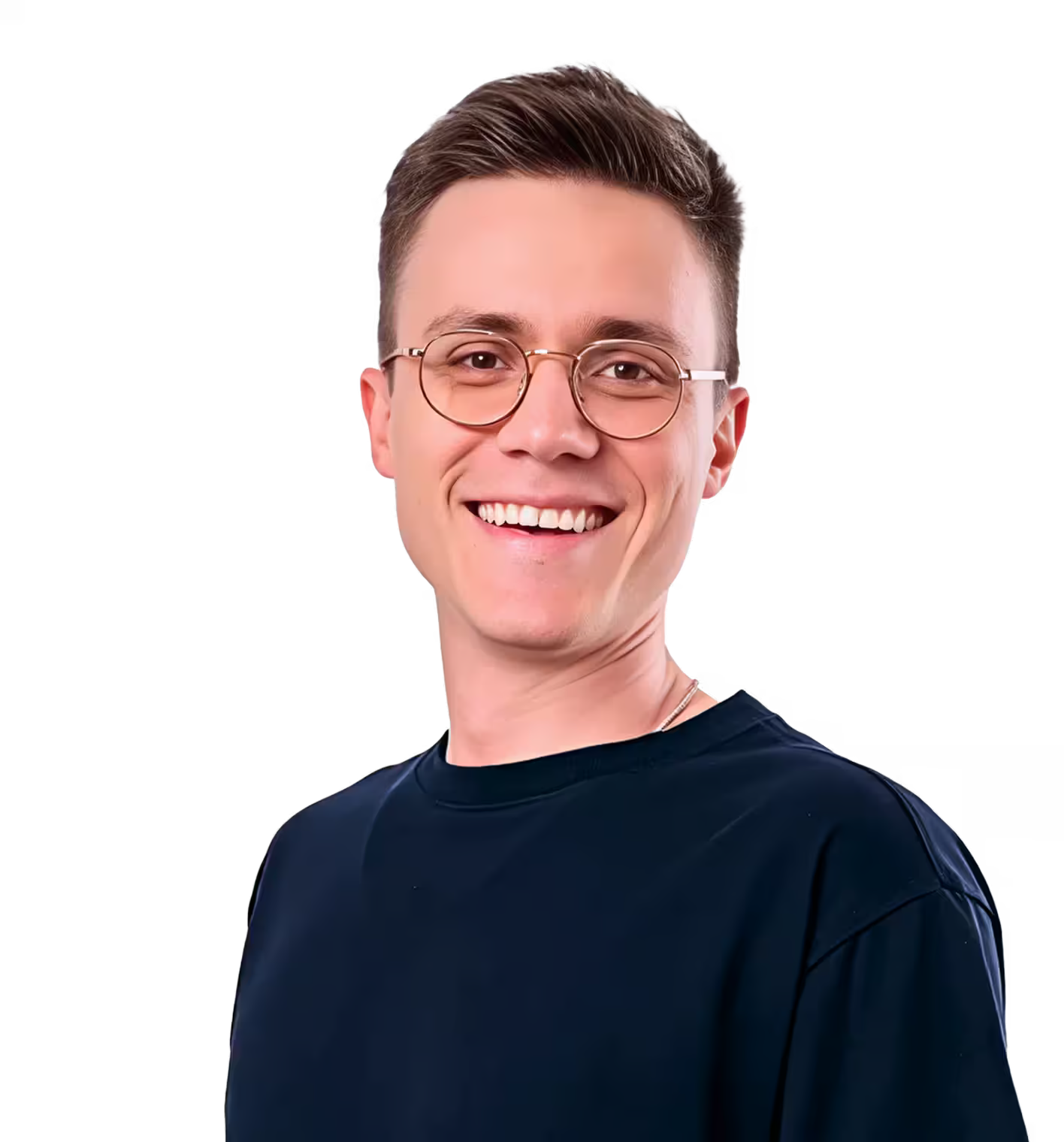
- Andrew
- Head of Client Success
— I'll guide you through every step, from your first
message to full project delivery
Thank you for your
message
We use cookies to enhance your experience, personalize content, ads, and analyze traffic. By clicking 'Accept All', you agree to our Cookie Policy.
Ready to get started?
Tell us what you need — we’ll reply within 24h with a free estimate